Re-evaluating GPT-4’s bar exam performance
Abstract
Perhaps the most widely touted of GPT-4’s at-launch, zero-shot capabilities has been its reported 90th-percentile performance on the Uniform Bar Exam. This paper begins by investigating the methodological challenges in documenting and verifying the 90th-percentile claim, presenting four sets of findings that indicate that OpenAI’s estimates of GPT-4’s UBE percentile are overinflated.
First, although GPT-4’s UBE score nears the 90th percentile when examining approximate conversions from February administrations of the Illinois Bar Exam, these estimates are heavily skewed towards repeat test-takers who failed the July administration and score significantly lower than the general test-taking population. Second, data from a recent July administration of the same exam suggests GPT-4’s overall UBE percentile was below the 69th percentile, and ∼48th percentile on essays. Third, examining official NCBE data and using several conservative statistical assumptions, GPT-4’s performance against first- time test takers is estimated to be ∼62nd percentile, including ∼42nd percentile on essays. Fourth, when examining only those who passed the exam (i.e. licensed or license-pending attorneys), GPT-4’s performance is estimated to drop to ∼48th percentile overall, and ∼15th percentile on essays. In addition to investigating the validity of the percentile claim, the paper also investigates the validity of GPT-4’s reported scaled UBE score of 298. The paper successfully replicates the MBE score, but highlights several methodological issues in the grading of the MPT + MEE components of the exam, which call into question the validity of the reported essay score.
Finally, the paper investigates the effect of different hyperparameter combi- nations on GPT-4’s MBE performance, finding no significant effect of adjusting temperature settings, and a significant effect of few-shot chain-of-thought prompt- ing over basic zero-shot prompting.
Taken together, these findings carry timely insights for the desirability and feasibility of outsourcing legally relevant tasks to AI models, as well as for the importance for AI developers to implement rigorous and transparent capabilities evaluations to help secure safe and trustworthy AI.
1. Introduction
On March 14th, 2023, OpenAI launched GPT-4, said to be the latest milestone in the company’s effort in scaling up deep learning [1]. As part of its launch, OpenAI revealed details regarding the model’s “human-level performance on various professional and academic benchmarks” [1]. Perhaps none of these capabilities was as widely publicized as GPT-4’s performance on the Uniform Bar Examination, with OpenAI prominently displaying on various pages of its website and technical report that GPT-4 scored in or around the “90th percentile,” [1-3] or “the top 10% of test-takers,” [1, 2] and various prominent media outlets [4–8] and legal scholars [9] resharing and discussing the implications of these results for the legal profession and the future of AI.
Of course, assessing the capabilities of an AI system as compared to those of a human is no easy task [10–15], and in the context of the legal profession specifically, there are various reasons to doubt the usefulness of the bar exam as a proxy for lawyerly competence (both for humans and AI systems), given that, for example: (a) the content on the UBE is very general and does not pertain to the legal doctrine of any jurisdiction in the United States [16], and thus knowledge (or ignorance) of that content does not necessarily translate to knowledge (or ignorance) of relevant legal doctrine for a practicing lawyer of any jurisdiction; and (b) the tasks involved on the bar exam, particularly multiple-choice questions, do not reflect the tasks of practicing lawyers, and thus mastery (or lack of mastery) of those tasks does not necessarily reflect mastery (or lack of mastery) of the tasks of practicing lawyers.
Moreover, although the UBE is a closed-book exam for humans, GPT-4’s huge training corpus largely distilled in its parameters means that it can effectively take the UBE “open-book”, indicating that UBE may not only be an accurate proxy for lawyerly competence but is also likely to provide an overly favorable estimate of GPT-4’s lawyerly capabilities relative to humans.
Notwithstanding these concerns, the bar exam results appeared especially startling compared to GPT-4’s other capabilities, for various reasons. Aside from the sheer complexity of the law in form [17–19] and content [20–22], the first is that the boost in performance of GPT-4 over its predecessor GPT-3.5 (80 percentile points) far exceeded that of any other test, including seemingly related tests such as the LSAT (40 percentile points), GRE verbal (36 percentile points), and GRE Writing (0 percentile points) [2, 3].
The second is that half of the Uniform Bar Exam consists of writing essays[16],[ref 1] and GPT-4 seems to have scored much lower on other exams involving writing, such as AP English Language and Composition (14th-44th percentile), AP English Literature and Composition (8th-22nd percentile) and GRE Writing (~54th percentile) [1, 2]. In each of these three exams, GPT-4 failed to achieve a higher percentile performance over GPT-3.5, and failed to achieve a percentile score anywhere near the 90th percentile.
Moreover, in its technical report, GPT-4 claims that its percentile estimates are “conservative” estimates meant to reflect “the lower bound of the percentile range,” [2, p. 6] implying that GPT-4’s actual capabilities may be even greater than its estimates.
Methodologically, however, there appear to be various uncertainties related to the calculation of GPT’s bar exam percentile. For example, unlike the administrators of other tests that GPT-4 took, the administrators of the Uniform Bar Exam (the NCBE as well as different state bars) do not release official percentiles of the UBE [27, 28], and different states in their own releases almost uniformly report only passage rates as opposed to percentiles [29, 30], as only the former are considered relevant to licensing requirements and employment prospects.
Furthermore, unlike its documentation for the other exams it tested [2, p. 25], OpenAI’s technical report provides no direct citation for how the UBE percentile was computed, creating further uncertainty over both the original source and validity of the 90th percentile claim.
The reliability and transparency of this estimate has important implications on both the legal practice front and AI safety front. On the legal practice front, there is great debate regarding to what extent and when legal tasks can and should be automated [31–34]. To the extent that capabilities estimates for generative AI in the context law are overblown, this may lead both lawyers and non-lawyers to rely on generative AI tools when they otherwise wouldn’t and arguably shouldn’t, plausibly increasing the prevalence of bad legal outcomes as a result of (a) judges misapplying the law; (b) lawyers engaging in malpractice and/or poor representation of their clients; and (c) non-lawyers engaging in ineffective pro se representation.Meanwhile, on the AI safety front, there appear to be growing concerns of transparency[ref 2] among developers of the most powerful AI systems [36, 37]. To the extent that transparency is important to ensuring the safe deployment of AI, a lack of transparency could undermine our confidence in the prospect of safe deployment of AI [38, 39]. In particular, releasing models without an accurate and transparent assessment of their capabilities (including by third-party developers) might lead to unexpected misuse/misapplication of those models (within and beyond legal contexts), which might have detrimental (perhaps even catastrophic) consequences moving forward [40, 41].
Given these considerations, this paper begins by investigating some of the key methodological challenges in verifying the claim that GPT-4 achieved 90th percentile performance on the Uniform Bar Examination. The paper’s findings in this regard are fourfold. First, although GPT-4’s UBE score nears the 90th percentile when examining approximate conversions from February administrations of the Illinois Bar Exam, these estimates appear heavily skewed towards those who failed the July administration and whose scores are much lower compared to the general test-taking population. Second, using data from a recent July administration of the same exam reveals GPT-4’s percentile to be below the 69th percentile on the UBE, and ~48th percentile on essays. Third, examining official NCBE data and using several conservative statistical assumptions, GPT-4’s performance against first-time test takers is estimated to be ~62nd percentile, including 42nd percentile on essays. Fourth, when examining only those who passed the exam, GPT-4’s performance is estimated to drop to ~48th percentile overall, and ~15th percentile on essays.
Next, whereas the above four findings take for granted the scaled score achieved by GPT-4 as reported by OpenAI, the paper then proceeds to investigate the validity of that score, given the importance (and often neglectedness) of replication and reproducibility within computer science and scientific fields more broadly [42–46]. The paper successfully replicates the MBE score of 158, but highlights several methodological issues in the grading of the MPT + MEE components of the exam, which call into question the validity of the essay score (140).
Finally, the paper also investigates the effect of adjusting temperature settings and prompting techniques on GPT-4’s MBE performance, finding no significant effect of adjusting temperature settings on performance, and some significant effect of prompt engineering on model performance when compared to a minimally tailored baseline condition.
Taken together, these findings suggest that OpenAI’s estimates of GPT-4’s UBE percentile, though clearly an impressive leap over those of GPT-3.5, are likely overinflated, particularly if taken as a “conservative” estimate representing “the lower range of percentiles,” and even moreso if meant to reflect the actual capabilities of a practicing lawyer. These findings carry timely insights for the desirability and feasibility of outsourcing legally relevant tasks to AI models, as well as for the importance for generative AI developers to implement rigorous and transparent capabilities evaluations to help secure safer and more trustworthy AI.
2. Evaluating the 90th Percentile Estimate
2.1. Evidence from OpenAI
Investigating the OpenAI website, as well as the GPT-4 technical report, reveals a multitude of claims regarding the estimated percentile of GPT-4’s Uniform Bar Examination performance but a dearth of documentation regarding the backing of such claims. For example, the first paragraph of the official GPT-4 research page on the OpenAI website states that “it [GPT-4] passes a simulated bar exam with a score around the top 10% of test takers” [1]. This claim is repeated several times later in this and other webpages, both visually and textually, each time without explicit backing.[ref 3]
Similarly undocumented claims are reported in the official GPT-4 Technical Report.[ref 4] Although OpenAI details the methodology for computing most of its percentiles in A.5 of the Appendix of the technical report, there does not appear to be any such documentation for the methodology behind computing the UBE percentile. For example, after providing relatively detailed breakdowns of its methodology for scoring the SAT, GRE, SAT, AP, and AMC, the report states that “[o]ther percentiles were based on official score distributions,” followed by a string of references to relevant sources [2, p. 25].
Examining these references, however, none of the sources contains any information regarding the Uniform Bar Exam, let alone its “official score distributions” [2, p. 22-23]. Moreover, aside from the Appendix, there are no other direct references to the methodology of computing UBE scores, nor any indirect references aside from a brief acknowledgement thanking “our collaborators at Casetext and Stanford CodeX for conducting the simulated bar exam” [2, p. 18].
2.2. Evidence from GPT-4 Passes the Bar
Another potential source of evidence for the 90th percentile claim comes from an early draft version of the paper, “GPT-4 passes the bar exam,” written by the administrators of the simulated bar exam referenced in OpenAI’s technical report [47]. The paper is very well-documented and transparent about its methodology in computing raw and scaled scores, both in the main text and in its comprehensive appendices. Unlike the GPT-4 technical report, however, the focus of the paper is not on percentiles but rather on the model’s scaled score compared to that of the average test taker, based on publicly available NCBE data. In fact, one of the only mentions of percentiles is in a footnote, where the authors state, in passing: “Using a percentile chart from a recent exam administration (which is generally available online), ChatGPT would receive a score below the 10th percentile of test-takers while GPT-4 would receive a combined score approaching the 90th percentile of test-takers.” [47, p. 10]
2.3. Evidence Online
As explained by [27], The National Conference of Bar Examiners (NCBE), the organization that writes the Uniform Bar Exam (UBE) does not release UBE percentiles.[ref 5] Because there is no official percentile chart for UBE, all generally available online estimates are unofficial. Perhaps the most prominent of such estimates are the percentile charts from pre-July 2019 Illinois bar exam. Pre-2019,[ref 6] Illinois, unlike other states, provided percentile charts of their own exam that allowed UBE test-takers to estimate their approximate percentile given the similarity between the two exams [27].[ref 7]
Examining these approximate conversion charts, however, yields conflicting results. For example, although the percentile chart from the February 2019 administration of the Illinois Bar Exam estimates a score of 300 (2-3 points higher thatn GPT-4’s score) to be at the 90th percentile, this estimate is heavily skewed compared to the general population of July exam takers,[ref 8] since the majority of those who take the February exam are repeat takers who failed the July exam [52][ref 9], and repeat takers score much lower[ref 10] and are much more likely to fail than are first-timers.[ref 11]
Indeed, examining the latest available percentile chart for the July exam estimates GPT-4’s UBE score to be ~68th percentile, well below the 90th percentile figure cited by OpenAI [54].
3. Towards a More Accurate Percentile Estimate
Although using the July bar exam percentiles from the Illinois Bar would seem to yield a more accurate estimate than the February data, the July figure is also biased towards lower scorers, since approximately 23% of test takers in July nationally are estimated to be re-takers and score, for example, 16 points below first-timers on the MBE [55]. Limiting the comparison to first-timers would provide a more accurate comparison that avoids double-counting those who have taken the exam again after failing once or more.
Relatedly, although (virtually) all licensed attorneys have passed the bar,[ref 12] not all those who take the bar become attorneys. To the extent that GPT-4’s UBE percentile is meant to reflect its performance against other attorneys, a more appropriate comparison would not only limit the sample to first-timers but also to those who achieved a passing score.
Moreover, the data discussed above is based on purely Illinois Bar exam data, which (at the time of the chart) was similar but not identical to the UBE in its content and scoring [27], whereas a more accurate estimate would be derived more directly from official NCBE sources.
3.1. Methods
To account for the issues with both OpenAI’s estimate as well the July estimate, more accurate estimates (for GPT-3.5 and GPT-4) were sought to be computed here based on first-time test-takers, including both (a) first-time test-takers overall, and (b) those who passed.
To do so, the parameters for a normal distribution of scores were separately estimated for the MBE and essay components (MEE + MPT), as well as the UBE score overall.[ref 13]
Assuming that UBE scores (as well as MBE and essay subscores) are normally distributed, percentiles of GPT’s score can be directly computed after computing the parameters of these distributions (i.e. the mean and standard deviation).
Thus, the methodology here was to first compute these parameters, then generate distributions with these parameters, and then compute (a) what per- centage of values on these distributions are lower than GPT’s scores (to estimate the percentile against first-timers); and (b) what percentage of values above the passing threshold are lower than GPT’s scores (to estimate the percentile against qualified attorneys).With regard to the mean, according to publicly available official NCBE data, the mean MBE score of first-time test-takers is 143.8 [55].
As explained by official NCBE publications, the essay component is scaled to the MBE data [59], such that the two components have approximately the same mean and standard deviation [53, 54, 59]. Thus, the methodology here assumed that the mean first-time essay score is 143.8.[ref 14]
Given that the total UBE score is computed directly by adding MBE and essay scores [60], an assumption was made that mean first-time UBE score is 287.6 (143.8 + 143.8).
With regard to standard deviations, information regarding the SD of first- timer scores is not publicly available. However, distributions of MBE scores for July scores (provided in 5 point-intervals) are publicly available on the NCBE website [58].
Under the assumption that first-timers have approximately the same SD as that of the general test-taking population in July, the standard deviation of first-time MBE scores was computed by (a) entering the publicly available distribution of MBE scores into R; and (b) taking the standard deviation of this distribution using the built-in sd() function (which calculates the standard deviation of a normal distribution).
Given that, as mentioned above, the distribution (mean and SD) of essay scores is the same as MBE scores, the SD for essay scores was computed similarly as above.
With regard to the UBE, Although UBE standard deviations are not publicly available for any official exam, they can be inferred from a combination of the mean UBE score for first-timers (287.6) and first-time pass rates.
For reference, standard deviations can be computed analytically as follows:
Where:
- x is the quantile (the value associated with a given percentile, such as a cutoff score),
- µ is the mean,
- z is the z-score corresponding to a given percentile,
- σ is the standard deviation.
Thus, by (a) subtracting the cutoff score of a given administration (x) from the mean (µ); and (b) dividing that by the z-score (z) corresponding to the percentile of the cutoff score (i.e., the percentage of people who did not pass), one is left with the standard deviation (σ).
Here, the standard deviation was calculated according to the above formula using the official first-timer mean, along with pass rate and cutoff score data from New York, which according to NCBE data has the highest number of examinees for any jurisdiction [61].[ref 15]
After obtaining these parameters, distributions of first-timer scores for the MBE component, essay component, and UBE overall were computed using the built-in rnorm function in R (which generates a normal distribution with a given mean and standard deviation).
Finally, after generating these distributions, percentiles were computed by calculating (a) what percentage of values on these distributions were lower than GPT’s scores (to estimate the percentile against first-timers); and (b) what percentage of values above the passing threshold were lower than GPT’s scores (to estimate the percentile against qualified attorneys).
With regard to the latter comparison, percentiles were computed after re- moving all UBE scores below 270, which is the most common score cutoff for states using the UBE [62]. To compute models’ performance on the individual components relative to qualified attorneys, a separate percentile was likewise computed after removing all subscores below 135.[ref 16]
3.2. Results
3.2.1. Performance against first-time test-takers
Results are visualized in Tables 1 and 2. For each component of the UBE, as well as the UBE overall, GPT-4’s estimated percentile among first-time July test takers is less than that of both the OpenAI estimate and the July estimate that include repeat takers.
With regard to the aggregate UBE score, GPT-4 scored in the 62nd percentile as compared to the ~90th percentile February estimate and the ~68th percentile July estimate. With regard to MBE, GPT-4 scored in the ~79th percentile as compared to the ~95th percentile February estimate and the 86th percentile July estimate. With regard to MEE + MPT, GPT-4 scored in the ~42nd percentile as compared to the ~69th percentile February estimate and the ~48th percentile July estimate.
With regard to GPT-3.5, its aggregate UBE score among first-timers was in the ~2nd percentile, as compared to the ~2nd percentile February estimate and
~1st percentile July estimate. Its MBE subscore was in the ~6th percentile, compared to the ~10th percentile February estimate ~7th percentile July estimate. Its essay subscore was in the ~0th percentile, compared to the ~1st percentile February estimate and ~0th percentile July estimate.
3.2.2. Performance against qualified attorneys
Predictably, when limiting the sample to those who passed the bar, the models’ percentile dropped further.
With regard to the aggregate UBE score, GPT-4 scored in the ~45th per- centile. With regard to MBE, GPT-4 scored in the ~69th percentile, whereas for the MEE + MPT, GPT-4 scored in the ~15th percentile.
With regard to GPT-3.5, its aggregate UBE score among qualified attorneys was 0th percentile, as were its percentiles for both subscores.
4. Re-Evaluating the Raw Score
So far, this analysis has taken for granted the scaled score achieved by GPT-4 as reported by OpenAI—that is, assuming GPT-4 scored a 298 on the UBE, is the 90th-percentile figure reported by OpenAI warranted?
However, given calls for the replication and reproducibility within the practice of science more broadly [42–46], it is worth scrutinizing the validity of the score itself—that is, did GPT-4 in fact score a 298 on the UBE?
Moreover, given the various potential hyperparameter settings available when using GPT-4 and other LLMs, it is worth assessing whether and to what extent adjusting such settings might influence the capabilities of GPT-4 on exam performance.
To that end, this section first attempts to replicate the MBE score reported by [1] and [47] using methods as close to the original paper as reasonably feasible. The section then attempts to get a sense of the floor and ceiling of GPT-4’s out-of-the-box capabilities by comparing GPT-4’s MBE performance using the best and worst hyperparameter settings.
Finally, the section re-examines GPT-4’s performance on the essays, eval- uating (a) the extent to which the methodology of grading GPT-4’s essays deviated that from official protocol used by the National Conference of Bar Examiners during actual bar exam administrations; and (b) the extent to which such deviations might undermine one’s confidence in the the scaled essay scores reported by [1] and [47].
4.1. Replicating the MBE Score
4.1.1. Methodology
Materials. As in [47], the materials used here were the official MBE questions released by the NCBE. The materials were purchased and downloaded in pdf format from an authorized NCBE reseller. Afterwards, the materials were converted into TXT format, and text analysis tools were used to format the questions in a way that was suitable for prompting, following [47]
Procedure. To replicate the MBE score reported by [1], this paper followed the protocol documented by [47], with some minor additions for robustness purposes. In [47], the authors tested GPT-4’s MBE performance using three different temperature settings: 0, .5 and 1. For each of these temperature settings, GPT- 4’s MBE performance was tested using two different prompts, including (1) a prompt where GPT was asked to provide a top-3 ranking of answer choices, along with a justification and authority/citation for its answer; and (2) a prompt where GPT-4 was asked to provide a top-3 ranking of answer choices, without providing a justification or authority/citation for its answer.
For each of these prompts, GPT-4 was also told that it should answer as if it were taking the bar exam.
For each of these prompts / temperature combinations, [47] tested GPT-4 three different times (“experiments” or “trials”) to control for variation.
The minor additions to this protocol were twofold. First, GPT-4 was tested under two additional temperature settings: .25 and .7. This brought the total temperature / prompt combinations to 10 as opposed to 6 in the original paper. Second, GPT-4 was tested 5 times under each temperature / prompt combination as opposed to 3 times, bringing the total number of trials to 50 as opposed to 18.
After prompting, raw scores were computed using the official answer key provided by the exam. Scaled scores were then computed following the method outlined in [63], by (a) multiplying the number of correct answers by 190, and dividing by 200; and (b) converting the resulting number to a scaled score using a conversion chart based on official NCBE data.
After scoring, scores from the replication trials were analyzed in comparison to those from [47] using the data from their publicly available github repository.To assess whether there was a significant difference between GPT-4’s accuracy in the replication trials as compared to the [47] paper, as well as to assess any significant effect of prompt type or temperature, a mixed-effects binary logistic regression was conducted with: (a) paper (replication vs original), temperature and prompt as fixed effects[ref 17]; and (b) question number and question category as random effects. These regressions were conducted using the lme4 [64] and lmertest [65] packages from R.
4.1.2. Results
Results are visualized in Table 4. Mean MBE accuracy across all trials in the replication here was 75.6% (95% CI: 74.7 to 76.4), whereas the mean accuracy across all trials in [47] was 75.7% (95% CI: 74.2 to 77.1).[ref 18]
The regression model did not reveal a main effect of “paper” on accuracy (p=.883), indicating that there was no significant difference between GPT-4’s raw accuracy as reported by [47] and GPT-4’s raw accuracy as performed in the replication here.
There was also no main effect of temperature (p>.1)[ref 19] or prompt (p=.741). That is, GPT-4’s raw accuracy was not significantly higher or lower at a given temperature setting or when fed a certain prompt as opposed to another (among the two prompts used in [47] and the replication here).
4.2. Assessing the Effect of Hyperparameters
4.2.1. Methods
Although the above analysis found no effect of prompt on model performance, this could be due to a lack of variety of prompts used by [47] in their original analysis.
To get a better sense of whether prompt engineering might have any effect on model performance, a follow-up experiment compared GPT-4’s performance in two novel conditions not tested in the original [47] paper.
In Condition 1 (“minimally tailored” condition), GPT-4 was tested using minimal prompting compared to [47], both in terms of formatting and substance. In particular, the message prompt in [47] and the above replication followed OpenAI’s Best practices for prompt engineering with the API [66] through the use of (a) helpful markers (e.g. ‘ “‘ ’) to separate instruction and context; (b) details regarding the desired output (i.e. specifying that the response should include ranked choices, as well as [in some cases] proper authority and citation; (c) an explicit template for the desired output (providing an example of the format in which GPT-4 should provide their response); and (d) perhaps most crucially, context regarding the type of question GPT-4 was answering (e.g. “please respond as if you are taking the bar exam”).
In contrast, in the minimally tailored prompting condition, the message prompt for a given question simply stated “Please answer the following question,” followed by the question and answer choices (a technique sometimes referred to as “basic prompting”: 67). No additional context or formatting cues were provided.
In Condition 2 (“maximally tailored” condition), GPT-4 was tested using the highest performing parameter combinations as revealed in the replication section above, with one addition, namely that: the system prompt, similar to the approaches used in [67, 68], was edited from its default (“you are a helpful assistant”) to a more tailored message that included included multiple example MBE questions with sample answer and explanations structured in the desired format (a technique sometimes referred to as “few-shot prompting”: [67]).
As in the replication section, 5 trials were conducted for each of the two conditions. Based on the lack of effect of temperature in the replication study, temperature was not a manipulated variable. Instead, both conditions featured the same temperature setting (.5).To assess whether there was a significant difference between GPT-4’s accuracy in the maximally tailored vs minimally tailored conditions, a mixed-effects binary logistic regression was conducted with: (a) condition as a fixed effect; and (b) question number and question category as random effects. As above, these regressions were conducted using the lme4 [64] and lmertest [65] packages from R.
4.2.2. Results
Mean MBE accuracy across all trials in the maximally tailored condition was descriptively higher at 79.5% (95% CI: 77.1 to 82.1), than in the minimally tailored condition at 70.9% (95% CI: 68.1 to 73.7).
The regression model revealed a main effect of condition on accuracy (β=1.395, SE=.192, p<.0001), such that GPT-4’s accuracy in the maximally tailored condition was significantly higher than its accuracy in the minimally tailored condition.
In terms of scaled score, GPT-4’s MBE score in the minimally tailored condition would be approximately 150, which would place it: (a) in the 70th percentile among July test takers; (b) 64th percentile among first-timers; and (c) 48th percentile among those who passed.
GPT-4’s score in the maximally tailored condition would be approximately 164—6 points higher than that reported by [47] and [1]). This would place it: (a) in the 95th percentile among July test takers; (b) 87th percentile among first-timers; and (c) 82th percentile among those who passed.
4.3. Re-examining the Essay Scores
As confirmed in the above subsection, the scaled MBE score (not percentile) reported by OpenAI was accurately computed using the methods documented in [47].
With regard to the essays (MPT + MEE), however, the method described by the authors significantly deviates in at least three aspects from the official method used by UBE states, to the point where one may not be confident that the essay scores reported by the authors reflect GPT models’ “true” essay scores (i.e., the score that essay examiners would have assigned to GPT had they been blindly scored using official grading protocol).
The first aspect relates to the (lack of) use of a formal rubric. For example, unlike NCBE protocol, which provides graders with (a) (in the case of the MEE) detailed “grading guidelines” for how to assign grades to essays and distinguish answers for a given MEE; and (b) (for both MEE and MPT) a specific “drafters’ point sheet” for each essay that includes detailed guidance from the drafting committee with a discussion of the issues raised and the intended analysis [69],
[47] do not report using an official or unofficial rubric of any kind, and instead simply describe comparing GPT-4’s answers to representative “good” answers from the state of Maryland.
Utilizing these answers as the basis for grading GPT-4’s answers in lieu of a formal rubric would seem to be particularly problematic considering it is unclear even what score these representative “good” answers received. As clarified by the Maryland bar examiners: “The Representative Good Answers are not ‘average’ passing answers nor are they necessarily ‘perfect’ answers. Instead, they are responses which, in the Board’s view, illustrate successful answers written by applicants who passed the UBE in Maryland for this session” [70].
Given that (a) it is unclear what score these representative good answers received; and (b) these answers appear to be the basis for determining the score that GPT-4’s essays received, it would seem to follow that (c) it is likewise unclear what score GPT-4’s answers should receive. Consequently, it would likewise follow that any reported scaled score or percentile would seem to be insufficiently justified so as to serve as a basis for a conclusive statement regarding GPT-4’s relative performance on essays as compared to humans (e.g. a reported percentile).
The second aspect relates to the lack of NCBE training of the graders of the essays. Official NCBE essay grading protocol mandates the use of trained bar exam graders, who in addition to using a specific rubric for each question undergo a standardized training process prior to grading [71, 72]. In contrast, the graders in [47] (a subset of the authors who were trained lawyers) do not report expertise or training in bar exam grading. Thus, although the graders of the essays were no doubt experts in legal reasoning more broadly, it seems unlikely that they would have been sufficiently ingrained in the specific grading protocols of the MEE + MPT to have been able to reliably infer or apply the specific grading rubric when assigning the raw scores to GPT-4.
The third aspect relates to both blinding and what bar examiners refer to as “calibration,” as UBE jurisdictions use an extensive procedure to ensure that graders are grading essays in a consistent manner (both with regard to other essays and in comparison to other graders) [71, 72]. In particular, all graders of a particular jurisdiction first blindly grade a set of 30 “calibration” essays of variable quality (first rank order, then absolute scores) and make sure that consistent scores are being assigned by different graders, and that the same score (e.g. 5 of 6) is being assigned to exams of similar quality [72]. Unlike this approach, as well as efforts to assess GPT models’ law school performance [73], the method reported by [47] did not initially involve blinding. The method in [47] did involve a form of inter-grader calibration, as the authors gave “blinded samples” to independent lawyers to grade the exams, with the assigned scores “match[ing] or exceed[ing]” those assigned by the authors. Given the lack of reporting to the contrary, however, the method used by the graders would presumably be plagued by issue issues as highlighted above (no rubric, no formal training with bar exam grading, no formal intra-grader calibration).
Given the above issues, as well as the fact that, as alluded in the introduction, GPT-4’s performance boost over GPT-3 on other essay-based exams was far lower than that on the bar exam, it seems warranted not only to infer that GPT-4’s relative performance (in terms of percentile among human test-takers) was lower than that reported by OpenAI, but also that GPT-4’s reported scaled score on the essay may have deviated to some degree from GPT-4’s “true” essay (which, if true, would imply that GPT-4’s “true” percentile on the bar exam may be even lower than that estimated in previous sections).
Indeed, [47] to some degree acknowledge all of these limitations in their paper, writing: “While we recognize there is inherent variability in any qualitative assessment, our reliance on the state bars’ representative “good” answers and the multiple reviewers reduces the likelihood that our assessment is incorrect enough to alter the ultimate conclusion of passage in this paper.”
Given that GPT-4’s reported score of 298 is 28 points higher than the passing threshold (270) in the majority of UBE jurisdictions, it is true that the essay scores would have to have been wildly inaccurate in order to undermine the general conclusion of [47] (i.e., that GPT-4 “passed the [uniform] bar exam”). However, even supposing that GPT-4’s “true” percentile on the essay portion was just a few points lower than that reported by OpenAI, this would further call into question OpenAI’s claims regarding the relative performance of GPT-4 on the UBE relative to human test-takers. For example, supposing that GPT-4 scored 9 points lower essays would drop its estimated relative performance to (a) 31st percentile compared to July test-takers; (b) 24th percentile relative to first-time test takers; and (c) less than 5th percentile compared to licensed attorneys.
5. Discussion
This paper first investigated the issue of OpenAI’s claim of GPT-4’s 90th percentile UBE performance, resulting in four main findings. The first finding is that although GPT-4’s UBE score approaches the 90th percentile when examining approximate conversions from February administrations of the Illinois Bar Exam, these estimates are heavily skewed towards low scorers, as the majority of test- takers in February failed the July administration and tend to score much lower than the general test-taking population. The second finding is that using July data from the same source would result in an estimate of ~68th percentile, including below average performance on the essay portion. The third finding is that comparing GPT-4’s performance against first-time test takers would result in an estimate of ~62nd percentile, including ~42nd percentile on the essay portion. The fourth main finding is that when examining only those who passed the exam, GPT-4’s performance is estimated to drop to ~48th percentile overall, and ~15th percentile on essays.
In addition to these four main findings, the paper also investigated the validity of GPT-4’s reported UBE score of 298. Although the paper successfully replicated the MBE score of 158, the paper also highlighted several methodological issues in the grading of the MPT + MEE components of the exam, which call into question the validity of the essay score (140).
Finally, the paper also investigated the effect of adjusting temperature settings and prompting techniques on GPT-4’s MBE performance, finding no significant effect of adjusting temperature settings on performance, and some effect of prompt engineering when compared to a basic prompting baseline condition.
Of course, assessing the capabilities of an AI system as compared to those of a practicing lawyer is no easy task. Scholars have identified several theoretical and practical difficulties in creating accurate measurement scales to assess AI capabilities and have pointed out various issues with some of the current scales [10–12]. Relatedly, some have pointed out that simply observing that GPT-4 under- or over-performs at a task in some setting is not necessarily reliable evidence that it (or some other LLM) is capable or incapable of performing that task in general [13–15].
In the context of legal profession specifically, there are various reasons to doubt the usefulness of UBE percentile as a proxy for lawyerly competence (both for humans and AI systems), given that, for example: (a) the content on the UBE is very general and does not pertain to the legal doctrine of any jurisdiction in the United States [16], and thus knowledge (or ignorance) of that content does not necessarily translate to knowledge (or ignorance) of relevant legal doctrine for a practicing lawyer of any jurisdiction; (b) the tasks involved on the bar exam, particularly multiple-choice questions, do not reflect the tasks of practicing lawyers, and thus mastery (or lack of mastery) of those tasks does not necessarily reflect mastery (or lack of mastery) of the tasks of practicing lawyers; and (c) given the lack of direct professional incentive to obtain higher than a passing score (typically no higher than 270) [62], obtaining a particularly high score or percentile past this threshold is less meaningful than for other exams (e.g. LSAT), where higher scores are taken into account for admission into select institutions [74].
Setting these objections aside, however, to the extent that one believes the UBE to be a valid proxy for lawyerly competence, these results suggest GPT-4 to be substantially less lawyerly competent than previously assumed, as GPT-4’s score against likely attorneys (i.e. those who actually passed the bar) is ~48th percentile. Moreover, when just looking at the essays, which more closely resemble the tasks of practicing lawyers and thus more plausibly reflect lawyerly competence, GPT-4’s performance falls in the bottom ~15th percentile. These findings align with recent research work finding that GPT-4 performed below-average on law school exams [75].
The lack of precision and transparency in OpenAI’s reporting of GPT-4’s UBE performance has implications for both the current state of the legal profession and the future of AI safety. On the legal side, there appear to be at least two sets of implications. On the one hand, to the extent that lawyers put stock in the bar exam as a proxy for general legal competence, the results might give practicing lawyers at least a mild temporary sense of relief regarding the security of the profession, given that the majority of lawyers perform better than GPT on the component of the exam (essay-writing) that seems to best reflect their day-to-day activities (and by extension, the tasks that would likely need to be automated in order to supplant lawyers in their day-to-day professional capacity).
On the other hand, the fact that GPT-4’s reported “90th percentile” capa- bilities were so widely publicized might pose some concerns that lawyers and non-lawyers may use GPT-4 for complex legal tasks for which it is incapable of adequately performing, plausibly increasing the rate of (a) misapplication of the law by judges; (b) professional malpractice by lawyers; and (c) ineffective pro se representation and/or unauthorized practice of law by non-lawyers. From a legal education standpoint, law students who overestimate GPT-4’s UBE capabilities might also develop an unwarranted sense of apathy towards developing critical legal-analytical skills, particularly if under the impression that GPT-4’s level of mastery of those skills already surpasses that to which a typical law student could be expected to reach.
On the AI front, these findings raise concerns both for the transparency[ref 20] of capabilities research and the safety of AI development more generally. In particular, to the extent that one considers transparency to be an important prerequisite for safety [38], these findings underscore the importance of implementing rigorous transparency measures so as to reliably identify potential warning signs of transformative progress in artificial intelligence as opposed to creating a false sense of alarm or security [76]. Implementing such measures could help ensure that AI development, as stated in OpenAI’s charter, is a “value-aligned, safety-conscious project” as opposed to becoming “a competitive race without time for adequate safety precautions” [77].
Of course, the present study does not discount the progress that AI has made in the context of legally relevant tasks; after all, the improvement in UBE performance from GPT-3.5 to GPT-4 as estimated in this study remains impressive (arguably equally or even more so given that GPT-3.5’s performance is also estimated to be significantly lower than previously assumed), even if not as flashy as the 10th-90th percentile boost of OpenAI’s official estimation. Nor does the present study discount the seemingly inevitable future improvement of AI systems to levels far beyond their present capabilities, or, as phrased in GPT-4 Passes the Bar Exam, that the present capabilities “highlight the floor, not the ceiling, of future application” [47, 11].
To the contrary, given the inevitable rapid growth of AI systems, the results of the present study underscore the importance of implementing rigorous and transparent evaluation measures to ensure that both the general public and relevant decision-makers are made appropriately aware of the system’s capabilities, and to prevent these systems from being used in an unintentionally harmful or catastrophic manner. The results also indicate that law schools and the legal profession should prioritize instruction in areas such as law and technology and law and AI, which, despite their importance, are currently not viewed as descriptively or normatively central to the legal academy [78].
Algorithmic black swans
Abstract
From biased lending algorithms to chatbots that spew violent hate speech, AI systems already pose many risks to society. While policymakers have a responsibility to tackle pressing issues of algorithmic fairness, privacy, and accountability, they also have a responsibility to consider broader, longer-term risks from AI technologies. In public health, climate science, and financial markets, anticipating and addressing societal-scale risks is crucial. As the COVID-19 pandemic demonstrates, overlooking catastrophic tail events — or “black swans” — is costly. The prospect of automated systems manipulating our information environment, distorting societal values, and destabilizing political institutions is increasingly palpable. At present, it appears unlikely that market forces will address this class of risks. Organizations building AI systems do not bear the costs of diffuse societal harms and have limited incentive to install adequate safeguards. Meanwhile, regulatory proposals such as the White House AI Bill of Rights and the European Union AI Act primarily target the immediate risks from AI, rather than broader, longer-term risks. To fill this governance gap, this Article offers a roadmap for “algorithmic preparedness” — a set of five forward-looking principles to guide the development of regulations that confront the prospect of algorithmic black swans and mitigate the harms they pose to society.
Three lines of defense against risks from AI
Abstract
Organizations that develop and deploy artificial intelligence (AI) systems need to manage the associated risks—for economic, legal, and ethical reasons. However, it is not always clear who is responsible for AI risk management. The Three Lines of Defense (3LoD) model, which is considered best practice in many industries, might offer a solution. It is a risk management framework that helps organizations to assign and coordinate risk management roles and responsibilities. In this article, I suggest ways in which AI companies could implement the model. I also discuss how the model could help reduce risks from AI: it could identify and close gaps in risk coverage, increase the effectiveness of risk management practices, and enable the board of directors to oversee management more effectively. The article is intended to inform decision-makers at leading AI companies, regulators, and standard-setting bodies.
Value alignment for advanced artificial judicial intelligence
Abstract
This paper considers challenges resulting from the use of advanced artificial judicial intelligence (AAJI). We argue that these challenges should be considered through the lens of value alignment. Instead of discussing why specific goals and values, such as fairness and nondiscrimination, ought to be implemented, we consider the question of how AAJI can be aligned with goals and values more generally, in order to be reliably integrated into legal and judicial systems. This value alignment framing draws on AI safety and alignment literature to introduce two otherwise neglected considerations for AAJI safety: specification and assurance. We outline diverse research directions and suggest the adoption of assurance and specification mechanisms as the use of AI in the judiciary progresses. While we focus on specification and assurance to illustrate the value of the AI safety and alignment literature, we encourage researchers in law and philosophy to consider what other lessons may be drawn.
Catastrophic risk review
Summary
In the United States, cost-benefit analysis plays a substantial role in government policy making in traditional regulatory areas, such as automobile safety and air quality. But the management of catastrophic risk has largely fallen outside the purview of cost-benefit analysis. The primary reasons that cost-benefit analysis has played a less important role in the context of catastrophic risks are procedural, rather than methodological. Although improvements can be made to cost-benefit analysis techniques to better account for catastrophic risks, the more important set of reforms—and the ones discussed in this proposal—are institutional.
In the U.S. regulatory context, cost-benefit analysis is embedded in the process of regulatory review, which is almost entirely reactive. Agencies initiate regulatory proposals and then work with the Office of Information and Regulatory Affairs (OIRA) to analyze the effects of their proposals and make revisions in light of that analysis. OIRA has very little influence over agency agenda setting, which is instead dominated by legal and political factors that are largely unmoored from cost-benefit considerations.
For traditional regulatory areas, the reactive posture of OIRA may not be ideal, but it is not altogether debilitating. In these areas, robust administrative agencies with substantial statutory mandates and long traditions of regulation are relatively well-positioned to identify and respond to policy needs. OIRA’s role of channeling and coordinating agency energies helps produce rules that are better justified and more likely to lead to net social benefits.
But the situation is very different for catastrophic risks. Regulatory review is well-suited for reining in overactive agencies or delaying or stopping imprudent agency action. But there are a wide range of catastrophic risks that are more likely to be exacerbated by government inaction than government action. Because OIRA does not evaluate agency agenda setting, cost-benefit analysis is not applied to the most consequential government decisions concerning catastrophic risks—the choice not to act.
The institutional reform discussed in this proposal is a Catastrophic Risk Review process, spearheaded by OIRA, that would examine catastrophic risks and potential government responses through a cost-benefit lens. This review process would build on earlier experiments in which OIRA has played a more proactive role in initiating regulatory actions. The two most successful of these experiments were the practice of prompt letters under the George W. Bush administration and the regulatory lookback undertaken by the Barack Obama administration. The Catastrophic Risk Review will also take advantage of OIRA’s experience in cross-agency coordination and harmonization, including the Obama administration’s interagency working group on the social cost of carbon, and the Bush administration’s Circular A-4 guidance document on cost-benefit analysis methodology.
The purpose of this process would be to find areas where tangible, cost-benefit justified policy steps can be undertaken to manage catastrophic risks. The Catastrophic Risk Review would be overseen and partially staffed by OIRA, but should also include an interagency coordination group. There should be at least one round of public comments to help identify risks and potential responses. The Review should have a relatively sweeping purview, examining regulatory as well as other governance tools, such as research subsidies or even new legislation, that may be appropriate.
Ideally, Catastrophic Risk Review would lead to an ongoing process of identifying and addressing this important category of risk. Right now, there is, essentially, a backlog of unaddressed catastrophic risk, so a substantial initial effort is needed. After that backlog has been cleared, a more regularized updating process can evaluate existing efforts on identified risks and determine whether new risks merit further attention.
The products of a Catastrophic Risk Review could include new actions undertaken by executive agencies, recommendations to Congress for legislation or funding, or guidance on methodological updates to cost-benefit analysis to better account for catastrophic risks.
Introduction
When Robert Oppenheimer was asked about his memories of the first successful test of a nuclear bomb at the Trinity site near Los Alamos, New Mexico, he recalled a line from the Bhagavad Gita where the god Vishnu takes on a terrifying, multiarmed form and says, “Now I am become death, the destroyer of worlds.”[ref 1] For many, the successful test of nuclear arms confirmed the human capacity to generate catastrophic risks—to become world-destroyers. As technological development has continued apace in the past three-quarters of a century, that potential has become only increasingly clear.
Fortunately, many catastrophic risks can, in theory, be efficiently managed. Although the risk of nuclear war is ever present, substantial steps have been taken to control access to bomb-making materials and to reduce existing stockpiles. At the same time, our understanding of the physical principles that underlie nuclear arms have also engendered the development of nuclear energy, a technology that, while it carries its own risks, also offers significant advantages as a carbon-free and stable source of electricity.
The challenge, as always, is to identify government interventions that generate risk-reduction benefits that justify their costs. In the United States, the use of cost-benefit analysis has a decades-long pedigree and is a well-entrenched part of the administrative state. However, evaluation of catastrophic risks using cost-benefit principles lags more traditional regulatory areas such as automobile safety and air quality. To bring catastrophic risks into the cost-benefit fold, reforms are needed.
Some of these reforms are methodological. When future costs and benefits are discounted, steps to avoid even extreme or catastrophic harms may be treated as having little value in present-day terms if the harms they avoid occur far enough in the future.[ref 2] Catastrophes may also impose harms that are different in kind than standard risks. The harm of a catastrophe that wipes out all of humankind is not captured by the sum of the value of statistical life of each person alive at the time of the event because such a catastrophe would cut off the possibility of all future lives.[ref 3] Catastrophic risks may involve some effects that are currently difficult to value and are left unquantified using existing techniques.[ref 4] Methodological reforms of the standard practice of cost-benefit analysis in the United States could improve how such catastrophic risks are valued.
However, the primary barriers to appropriate treatment of catastrophic risks in U.S. regulatory decision making are not methodological. Even if appropriate methodological reforms were made, the institutional context of how cost-benefit analysis fits into the regulatory process would lead to inadequate attention to catastrophic risks. Although it is possible that a catastrophic risk could be created or exacerbated by an agency action of some kind, it is far more likely that the failure to act, rather than an action imprudently undertaken, would contribute to catastrophic risks. Indeed, agency inaction almost certainly already contributes to catastrophic risks on many fronts—climate change is an obvious example, but almost all categories of catastrophic risks, including those arising from advanced artificial intelligence, asteroid impacts, pandemics, weapons of mass destruction, and bioengineering, are exacerbated by the failure of U.S. policymakers to take steps that are currently available and that would be cost-benefit justified, even using current cost-benefit analysis methodologies.
For cost-benefit analysis to play a useful role in informing regulatory decision making regarding catastrophic risks, the primary reforms that are needed are institutional rather than methodological. Currently, cost-benefit analysis is used during a reactive process of regulatory oversight: agency agendas are developed on the basis of various legal, policy, and political criteria, and cost-benefit analyses are prepared of individual regulatory proposals. These analyses are then reviewed by the Office of Information and Regulatory Affairs (OIRA) in the White House and become part of the administrative record that is reviewed by courts in the course of litigation challenging agency decisions, typically under the Administrative Procedure Act (APA). Cost-benefit analysis is an important input during the process of regulatory design and analysis, but it plays a much more limited role in the context of agency agenda setting.[ref 5] Given this institutional arrangement, it is extremely difficult for cost-benefit analysis to be used to call attention to underappreciated risks.
There have been attempts by prior administrations to leverage OIRA’s cost-benefit expertise to inform efforts by the White House to direct agency agenda setting. The two most successful were the practice of prompt letters initiated by Administrator John Graham during the George W. Bush administration and the regulatory lookback effort initiated by Administrator Cass Sunstein during the Barack Obama administration.[ref 6] Prompt letters were used by OIRA to direct agency attention to cost-benefit-justified actions that the agencies were not undertaking. Some prompt letters were deregulatory in nature, and agencies were directed to existing regulatory interventions that OIRA believed should be either revised or rescinded.[ref 7] But prompt letters were also used to address the failure of agencies to address certain risks. The regulatory lookback process was initiated to urge agencies to review their existing stock of regulatory requirements to identify rules that were good candidates for revision on cost-benefit criteria. In the lookback process, all agencies were directed to submit reports to OIRA on the results of this review process, and many agencies identified, and ultimately adopted, reforms that led to substantial cost savings.
Building on these prior efforts, this essay proposes a Catastrophic Risk Review process, spearheaded by OIRA, that would examine opportunities across the federal government to address catastrophic risks, and would also identify governance gaps where existing authority is inadequate. The purpose of this process would be to find areas where tangible, cost-benefit-justified policy steps could be undertaken to manage catastrophic risks. The potential policy measures examined should include regulatory interventions as well as other governance tools, including research subsidies, that may be appropriate. This process could be used to inform agency agenda setting as well as OIRA’s annual Report to Congress on the Costs and Benefits of Federal Rulemaking.
Because OIRA is already severely over-taxed with its existing functions,[ref 8] there is a concern that any additional responsibilities would necessarily require OIRA to shift personnel from its core functions. For this reason, were the administration to undertake a Catastrophic Risk Review, it should request additional resources from Congress to appropriately staff this initiative. OIRA is chronically underfunded, and any additional responsibilities charged to the agency should be met with appropriate increases in the resources that are available to the office.
The remainder of this Essay proceeds as follows. Part I provides some background on the limited role of cost-benefit analysis is agency-agenda setting, discussing the legal, institutional, and political factors that influence how agencies allocate their limited resources. Part II discusses the problem of agency inaction, examining problems of overly active and overly passive agencies. Part III describes why catastrophic risks are particularly prone to generate agency inattention and inaction. This part draws from relevant psychological literature on risk perception, as well as more general political theory concerning agency incentives. Part IV outlines a proposed Catastrophic Risk Review, describing how it can build on successful prior practices, and how it can help integrate cost-benefit analysis into the process of understanding and addressing risks that have the potential to profoundly affect the future of humankind.
I. Cost-Benefit Analysis and Agency Agenda Setting
Among the most important decisions that agencies face is how to allocate their limited capacities. Agencies are charged with a wide range of tasks that include rulemaking and enforcement, permitting, grant oversight, and research. The resources to carry out these tasks, which include personnel, political capital, and financing, are all, of course, scarce. Given the substantial mandates of many agencies, there is a nearly unlimited possible set of priorities that they could adopt. Even in an ideal decision-making environment, determining how to distribute finite resources to best promote public well-being would be a difficult and complex task. And, of course, agencies are not embedded in an ideal decision-making environment. Instead, they must tailor their agendas to fit legal constraints, changing priorities of political overseers, and an often-steady stream of small-scale emergencies.
In practice, there are wide range of influences that affect how agencies devote their time and energies.[ref 9] The most important class of external influences arise from courts, Congress, and the White House. With respect to courts, agencies are continually emmeshed in litigation, which expose them to near-constant oversight by courts. Judges commonly strike down and seek to shape agency actions. The standards set by judicial review—foremost, how courts have interpreted the “arbitrary or capricious” standard under the APA—have created a wide-ranging set of procedural and analytic requirements.[ref 10] These requirements dictate, to a considerable extent, the resources that agencies must devote to the rulemaking process. The extensive requirements of courts constrain the agenda space of agencies, given a finite rulemaking budget. Courts also review how agencies interpret the laws that they administer and regularly reverse agency actions that are determined to be contrary to statutory authority.
With respect to Congress, the most straightforward mechanism of legislative control is through the law, which determines the scope of agency authority and often prescribes in substantial detail how regulatory activities must take place.[ref 11] Congress also sets agency budgets, and the budget rider process is frequently directed at affecting agency decision making. Agencies must also be responsive to congressional hearings and other oversight activities.
The White House is the third major oversight body. Political scientist Terry Moe has usefully classified the tools that Presidents use to influence agency decision making as either centralization or politicization.[ref 12] Centralization tools shift the locus of decision making from agencies to the White House. The canonical example of centralization is regulatory review by OIRA, but there is now a substantial presidential bureaucracy that either substitutes for, or at the very least complements, agency decision making. Politicization refers to the increasing trend of Presidents to place loyalists in the most senior managerial positions at agencies. These political appointees are then charged with ensuring that agencies reflect the current priorities of the administration.
Beyond the constitutional branches, there are a wide range of external actors that affect how agencies allocate their scarce resources, in part mediated through courts, Congress, or the White House. Under the APA and other relevant laws, interested parties can petition agencies for rulemakings, and, when those petitions are denied, agencies may be called to defend those decisions in court. A petitioning process under the Endangered Species Act is a common tool used by environmental organizations for directing agency attention to species of concern.[ref 13] In Massachusetts v. EPA, the Supreme Court found that the Clean Air Act provided the Environmental Protection Agency (EPA) with the authority to regulate greenhouse gas emissions in the course of reviewing that agency’s denial of a petition for rulemaking.[ref 14] Private parties also decide what agency actions they wish to challenge and whether to pursue political action to attempt to trigger oversight by Congress or the White House.
Agencies also have their own internal reasons to be concerned with the views of interest groups and public opinion more broadly. Good relations between an agency and regulated actors helps facilitate voluntary compliance, an essential in light of limited enforcement budgets.[ref 15] Personnel at agencies may seek to maintain reputations among potential employers to increase their chances of securing lucrative opportunities after their time in government. The staff at agencies may also be concerned about broader public perceptions of the efficacy and usefulness of their organizations.
In addition to external influences, agencies have their own internal processes that help shape their agendas. Some agencies conduct periodic reviews of certain regulatory regimes to update requirements on the basis of new information. New regulatory initiatives are sometimes undertaken as the result of internal study groups or task forces. Agency culture, which tends to remain relatively stable over time, likely also has a role to play in influencing agency agenda setting. Agencies also attend to the work of related government institutions, including other agencies, as well as scientific institutions and assessment bodies, such as those convened by the National Academies. Pressures within the executive is another set of influences on agency agenda setting.[ref 16]
A perhaps striking feature of the various external and internal influences on agency agenda setting is the lack of any formal use of cost-benefit criteria. Some judges may also be more positively disposed toward rules that have overall beneficial effects on society,[ref 17] although the values that individual judges bring to decisions may or may not align with efficiency.[ref 18] It is possible that Congress and the White House have some implicit orientation toward interventions that deliver net benefits, in as much as political officials are judged by voters on the basis of sound government policy. But interest group pressure, partisanship, and symbolic gesturing may be as strong an influence on how political oversight is exercised as any concern with efficiency or welfare maximization. Agencies may have internal norms that favor the prioritization of regulatory actions with substantial welfare payoffs, but there are many internal factors at play beyond regard for social welfare. The case-by-case oversight provided by courts is particularly ill-suited toward pressuring agencies to allocate resources across potential actions in a sensible fashion. There is generally no argument under the APA that an agency’s action is arbitrary merely because it reflects poor agenda setting (i.e., that the agency could have engaged in an unrelated action with higher net benefits). These are the kinds of prioritization decisions that courts are inclined to leave to executive discretion.
The upshot of the decision-making environment faced by agencies is that agenda setting is likely to be responsive to various actors, but it is very unlikely to reflect a rational allocation of government resources toward the goal of welfare maximization. Agencies set their agendas to please political overseers, improve their chances of success in court, respond to pressure from interest groups, and remain in relatively good standing in the eyes of the public. Elections, the flux of economic and political events, salient accidents that lead to media attention, and other exigencies often require some form of immediate response, which can distract agencies from longer-term priorities. A particular problem in the contemporary period is the wildly oscillating policy priorities of agencies after changes in the White House.[ref 19] It is not uncommon for a presidential election to result in agency personnel being required to execute an about-face and begin undoing their work over the prior several years.
Reforms to cost-benefit analysis methodology to improve treatment of catastrophic risks can help agencies better understand and manage those risks. But because cost-benefit analysis plays relatively little role placing items on agencies’ agendas, those improvements will be mostly relevant for risks that have been identified, characterized, and allocated to an agency through some other process—such as congressional oversight. The processes that affect agency agenda setting are responsive to a wide range of political, economic, and social forces. But there is no formal, and likely little informal, role for cost-benefit analysis in urging agencies to shift from the status quo.
II. The Problem of Agency Inaction
As discussed in the previous section, the role of cost-benefit analysis on agency decision making is almost entirely as a constraint, not a directive force. OIRA reviews agency cost-benefit analyses and may suggest changes to increase net benefits, but the initiative to start the rulemaking process is with the agency. The alternatives discussed during review are typically relatively minor revisions, not whether a rule should be pursued at all in light of available alternative regulatory emphases. Rationality review by courts similarly focuses on the rule at hand, and courts do not ask agencies to investigate the opportunities of pursing one regulatory matter over another. Agencies may occasionally decline to pursue regulations that they believe are not cost-benefit justified—either based on internal norms or due to the presence of executive and judicial review. But once a rule can be justified on legal and cost-benefit terms, review largely loses its ability to direct agency behavior.
There is an influential line of thought that agencies will tend to be overactive, zealously pursuing regulatory mandates and seeking to maximize their influence whenever possible. If this claim is roughly correct, then concerns about agency prioritization could be dealt with via the use of cost-benefit analysis as a constraint. Agencies would be expected to occupy as much space as they possibly can, and regulatory review would limit that space to cost-benefit-justified rules. The result would be welfare maximizing, in that all cost-benefit-justified regulatory interventions would be identified and exploited. This would hold for interventions to address catastrophic risks as well as other categories of harm. Under the overactivity hypothesis, whenever an existing mandate provides authority to address catastrophic risks, agencies can be expected to pursue regulatory actions in excess of those that would be justified in cost-benefit terms. So long as regulatory review is there to rein in agencies where needed, the system will achieve appropriate levels of risk management.
The problem with the overactive agency hypothesis is that it is more of a caricature than a realistic picture of how agencies work in practice.[ref 20] The structure of agencies and the political environments in which they are embedded creates a complex set of incentives and behaviors. Sometimes, that may lead to overly stringent regulation or harsh enforcement; at other times, agencies will fail to regulate or engage in lax enforcement. Rather than a consistent set of broad tendencies, agency behavior is highly context specific and can depend on a wide range of changing factors, including the political oversight of the day or even the specific inclinations of certain important career personnel.
Many of the arguments offered in support of the overactivity hypothesis have a foundation in reality, but they also tend to be overly simplistic. For example, economists in the public choice tradition have pointed out that industry actors may seek out government regulation in their sector as a way to erect barriers to entry.[ref 21] And this story likely has some element of truth: it may be that the incumbent players can shape regulatory interventions in ways that limit their exposure to costs while imposing heavy burdens on potential competitors. This is a legitimate concern and may lead to inefficient rules in some contexts. However, although industry may attempt to use its influence to seek out new rules that burden competitors, it may also seek to be free from regulatory costs altogether, shifting the cost of inaction to the broader, less well-organized public. Even accepting the public choice account of industry influence, it is far from clear that the net effect of industry lobbying will be overactive government.
Others have focused on the incentives or preferences of agency personnel to argue in favor of the overactivity hypothesis. Willian Niskanen, for example, argued that agency heads will seek to increase their own salaries and prestige by seeking ever larger budgets and mandates.[ref 22] For Niskanen and others who have taken up this argument, this tendency toward self-aggrandizement is a simple extension of a rational choice model applied to agency heads. But the link between the budget and mandate of an agency, and the utility enjoyed by the agency’s leadership, is extremely tenuous.[ref 23] Agencies are not private firms in which pay for senior executives is linked to measures such as share prices or profits. An increase in an agency’s budget or the scope of its mandate does not translate in any straightforward way to monetary compensation for senior officials. Whatever benefits that are generated by aggrandizement are more likely to be psychological rather than pecuniary. Seeking out larger budgets and mandates also comes with downsides for agency leadership. The kind of controversy and oversight associated with expanding budgets and mandates would have disutility for many agency heads.
Another possibility related to the Niskanen theory is that agency leadership and staff tend to identify with their agency’s mission, which causes them to focus myopically on forwarding that mission at the expense of the broader social good. Again, there is some plausibility to this idea, and this phenomenon may have an influence on agency behavior some of the time. But agency personnel are drawn to government service for many reasons apart from attraction to an agency’s mission. Some may seek out opportunities for public service generally, and not have strong commitments to any particular policy area. Others may be attracted to the pay, benefits, and lifestyle associated with federal government employment and be indifferent to how their work fits into a broader policy program. Some agency heads may even receive utility from undermining the work of the agency and shrinking its budget and mandate—there were several appointees during the Trump administration for whom this appears to have been the case.
Various versions of the overactivity hypothesis also fail to account for the legal and political operating environment of agencies, which often has a strong status quo bias. Agencies face a host of formal and informal barriers that increase the cost and decrease the payoff of major regulatory action. Under the Administrative Procedure Act, agencies have substantial data collection, reason-giving, and public participation requirements. Internal executive requirements concerning interagency cooperation and regulatory review further add time and resource costs to agency action. These analytic and participatory requirements may all be justified, and may lead to improved decision making, but they nonetheless imposed burdens on agency action.
The process of judicial review also adds an extraordinary amount of uncertainty to the regulatory process. Even after years of expensive regulatory process and detailed analysis, even a very well justified rule can be struck down by an ill-disposed appellate court panel. Courts have many options for striking down agency rules, including flaws (or purported flaws) in a rulemaking process or substantive disagreement over the interpretation of a relevant statute. Recent changes in administrative law, such as the expanded “major questions” doctrine announced in West Virginia v. EPA, only inject further uncertainty into the process of judicial review.[ref 24] As with the procedural requirements of the APA and the analytic requirements within the executive, judicial review may, overall, be a positive influence on regulatory decision making. But it nonetheless makes rulemaking more difficult and uncertain.
It is worth emphasizing the asymmetrical nature of the legal environment of agency rulemaking. The procedural requirements of the APA are triggered by agency action, but not by agency inaction. Although the APA provides that courts may “compel agency action unlawfully withheld or unreasonably delayed,”[ref 25] the burden placed on plaintiffs to justify this remedy is so high that this provision is nearly a dead letter.[ref 26] Even denials of petitions for rulemakings (which are technically an agency action) are given extremely deferential review by agencies.[ref 27] Within the executive, barring the examples discussed in Part IV, agency inaction is not subject to formal centralized oversight.
There is an important set of counterweights that, to some degree, balance the strong status quo bias of agencies’ institutional environments. Presidents enter office with policy programs that often rely on agency action. Especially in an era of partisan gridlock in Congress, Presidents must look to executive agencies to pursue their policy goals.[ref 28] There are several offices within the White House charged with coordinating across government agencies to promote the administration’s agenda. These entities—such as the Domestic Policy Council and various ad hoc advisors’ offices—provide a source of pressure on agencies that encourage them to be active in relevant domains. Presidents are also inclined to act, or be perceived as acting, on the crises of the day, which is another impetus for agency action. And although action can subject an agency to external critique, including by Congress, in certain circumstances, agency inaction will also be criticized. Especially in the case of highly salient negative events—such as the Flint Water Crisis—agency inaction can come under harsh scrutiny.
If these counterweights—which provide agencies with an impetus to action—are sufficiently strong, it is at least possible that in some areas, a system in which cost-benefit analysis acts primarily as a constraint could lead to efficient outcomes. In a policy domain that the White House has prioritized, an agency could face sufficient pressure to act such that the main concern (from a social welfare perspective) is too much, rather than too little, activity. There are good reasons to be skeptical that there is an overall tendency within the administrative state toward overactivity. But whether there are specific policy domains where such a dynamic exists will be a context-specific empirical question. For reasons described in the next Part, catastrophic risk is unlikely to be such a domain.
III. Systematic Inattention to Catastrophic Risk
There are three primary reasons why agencies are often likely to underemphasize catastrophic risks. The first is legal. Catastrophic risks are often cross-cutting and are not clearly delegated to specific agencies. The second is political. In a constitutional system that is fundamentally grounded in electoral accountability, long-term, global risks will likely be underprioritized. The third is psychological. Human beings have difficulty reasoning about low-probability events and long time horizons; this leads both voters and government officials to neglect catastrophic risks. A dedicated review process focused on catastrophic risks through a cost-benefit lens, discussed in more detail in Part IV, can help overcome these biases.
Catastrophic harms may come in many shapes, only some of which fit easily in the agency mandates constructed in U.S. law. The response to the covid-19 pandemic is illustrative. Authority over the varied public policy responses to the pandemic was deeply fractured. Vertically, important decisions were vested at all levels, from municipalities through states to the national government. Horizontally, different agencies were charged with different elements of the response, which included outbreak monitoring, public health measures, vaccine and drug development, and deployment of medical resources. Coordination was a constant challenge and pandemic-related policies quickly became embroiled in partisan conflict, which reduced the efficacy of the U.S. response.
The fractured authority over pandemic-related policies not only inhibited efforts to address covid-19 after it emerged. Perhaps more important was the inadequacy of ex-ante planning to address a threat of this kind. The risk of a severe global pandemic was well known within the public health community, and near misses with earlier coronavirus outbreaks had provided ample warning even that this specific family of viruses posed a threat. Nevertheless, the lack of a clear delegation of authority to a competent agency charged with building the necessary capacity and policies to address pandemic risks likely contributed to the chaotic and ineffective U.S. response.
Climate change is an example of a context wherein management of catastrophic risk, even in a near-best-case scenario, the muddled nature of authority and its diffusion between multiple actors raises challenges. Since at least the Clinton administration, the Environmental Protection Agency has interpreted the Clean Air Act to grant it authority to regulate greenhouse gas emissions. That authority was settled in Massachusetts v. EPA and the agency has moved forward, in a limited way, with rules to reduce emissions. But national authority over climate policy more generally is more fractured, with the Department of Energy, the Agriculture Department, the Federal Energy Regulatory Commission, the Nuclear Regulatory Commission, the National Aeronautics and Space Administration, the Federal Emergency Management Agency, and others playing a role in various elements of climate research and policy. Some states—most notably California—have played a leading role as well, in part because the federal government’s response has been weak and inconsistent. And even EPA’s authority is under a cloud of uncertainty after the Supreme Court’s recent decision in West Virginia.[ref 29]
For other catastrophic risks, the situation is even more dire. There is no agency like the EPA that has taken a leading role in addressing advanced artificial intelligence, bioengineering, or other sources of catastrophic risk. In many instances, the concern is not that authority is too diffused—as is likely the case for pandemic risks. Rather, authority is sufficiently unclear that it is difficult to even identify a default actor whose responsibility it would be to recognize and begin to consider the relevant risk. This missing responsibility makes inattention to catastrophic risks all the more likely.
The nature of the U.S. political process also biases government decision makers toward inattention to many catastrophic risks. Large-scale nuclear war, climate change, artificial intelligence, severe global pandemics, runaway bioengineering, and asteroid strikes all present substantial scale mismatches with national electoral democracy. These scale mismatches arise because the vast majority of stakeholders who are affected by decisions concerning these risks fall outside the voting public.
The first scale mismatch is spatial. Many, if not all, catastrophic risks are global in nature. To qualify as catastrophic, a risk must place an extremely large number of people in harm’s way and threaten the continued viability of human development, modern civilization, or humankind. For national-level political processes, most of the harms caused by actions that exacerbate catastrophic risks, or benefits that result from steps to mitigate these risks, will fall extra-jurisdictionally. We can expect rational self-interested politicians and voters to underinvest attention in these issues, from the perspective of global welfare. More salient, localized issues, such as employment, economic growth, crime, and education, will likely continue to win out for attention compared to catastrophic risks whose effects will occur on a global scale.
The covid-19 pandemic and climate change again illustrate the natural domestic myopia of national institutions. Despite the clearly global nature of these threats, policies made at the national level have tended to favor local interests and disregard cross-border effects. With respect to the pandemic, vaccine supplies were, and continue to be, distributed extremely unevenly across the globe, with the richer countries experiencing an oversupply, and developing countries continuing to struggle to gain access to high-quality vaccines. This situation has created opportunities for the virus to mutate, leading to successive rounds of variants that have increasing ability to evade existing vaccines. A global approach would have focused vaccine supply in a more efficient manner to maximize total immunity and reduce the risks caused by new variants.
With respect to climate change, the debate in the United States over the social cost of carbon encapsulates the difficulties associated with national-level policymakers addressing global catastrophic challenges. The social cost of carbon is a monetary estimate of the damages associated with greenhouse gas emissions. There are a number of contested questions that arise when estimating the social cost of carbon, including how best to model damages and the discount rate to use for harms in the future. In the United States, the question of whether the social cost of carbon should include all damages, or only those that occur domestically, has also arisen. The Obama and Biden administrations have used a global estimate, while the Trump administration favored a domestic-only social cost of carbon. Without debating the economic, legal, or ethical merits of the alternative approaches to the social cost of carbon, the controversy demonstrates that at least some of the time, domestic political institutions can be expected to discount, or even disregard entirely, the global consequences of their actions.[ref 30]
The second scale mismatch is temporal. Most catastrophic risks are long term and relatively unlikely to be realized within the next few decades. But even very small risks, if they are consistent, are translated into near certainties over time. For example, if each year, humanity faces a one-in-a-million risk of an asteroid strike, then the risk of occurrence within a timespan that is meaningful for politics, which operates at a decadal basis at most, would still be very small. But over a 50,000-year time horizon (roughly the period of time since the Cognitive Revolution) the same risk translates into, approximately, a 5% probability of a strike at some point. Political cycles are entirely out of sync with these time spans, and seemingly rational treatment of de minimis probabilities results in the neglect of very meaningful risks over the long term.
If a catastrophic risk were realized, it would also impose costs indefinitely. An artificial super-intelligence that was indifferent to human well-being might convert massive amounts of resources to its own aims, substantially reducing the prospects of human development.[ref 31] Such an occurrence would likely be irreversible, as the super-intelligence would continue to advance while humankind stagnated. The prospects of generation after generation of people would be severely diminished, potential affecting many billions of persons. But nearly all (or all) of the persons at risk from these harms have not yet been born, and they are certainly not voting members of the public. The normal functioning of democratic institutions will orient them toward the concerns of existing voters, not such future persons.
Finally, the human beings that make up the voting public, politicians, and agency personnel are all subject to psychological tendencies that incline them to underemphasize certain types of catastrophic risks. There is a substantial body of research that documents the difficulties that people have with thinking in probabilistic terms, especially when the relevant probabilities are very small.[ref 32] People will sometimes ignore events whose probabilities fall below a certain threshold.[ref 33] In the case of many catastrophic risks, the probability that any would occur in a given person’s lifespan is quite small, potentially leading to the neglect of this risk altogether. The consequences of catastrophic risks—such as nuclear war, large-scale environmental destruction, or asteroid strike—may be so grave that people may avoid thinking about them in order to prevent negative emotional experiences.[ref 34] These risks can also be difficult to manage, leading to feelings of ineffectiveness and helplessness—both negative emotions that people will tend to avoid if possible.[ref 35] The solutions that do exist may also involve institutional or policy choices that people may find unpleasant.[ref 36] Increased government supervision of technological development—which may be necessary to monitor and mitigate the risks associated with artificial intelligence—may strike many people as intrusive and contrary to values of economic liberty. Global cooperation to address climate change may challenge people’s national-level identify affiliation. Aversion to such solutions may lead people to downplay or deemphasize the risk in question, so that they might avoid the cognitive dissonance associated with making tragic choices between undertaking necessary but disagreeable steps to address a catastrophic risk or making an informed and clear-eyed choice not to.
It bears mentioning that the overall effect of many of these psychological tendencies is ambiguous, and some of them may result in people paying too much attention to certain types of catastrophic risks. For example, a substantial body of research shows that people are inclined to overestimate risks when they are salient.[ref 37] The entertainment industry plays a role in vividly rendering some catastrophic risks in realistic terms that are easily accessible to the human imagination.[ref 38] Dreaded risks also tend to be overestimated.[ref 39] But at least some of the time, human psychology will lead relevant decisionmakers to avoid focusing on certain catastrophic risks, even when it would be rational to do so.
IV. Catastrophic Risk Review
The prior three sections have established the problem. Currently, there is little formal role for cost-benefit analysis in agency agenda setting. Catastrophic risks, like all other risks that are relevant for government decision making, are primarily weighed in a cost-benefit fashion after agency agendas have already been firmly set. This state of affairs, in which the role of cost-benefit analysis is to constrain agency behavior, would be acceptable if agencies could be expected to be generally overactive, expanding and pushing their authority to the maximum extent possible. But this is the not the case, especially so for catastrophic risks. Agencies are complex entities operating in complex political environments. Some of the time, agencies may tend toward overactivity, but there is at least as much reason to be concerned with agency inaction as with inefficient action. And catastrophic risks have several legal, political, and psychological dimensions that make inattention particularly likely.
The proposal in this Essay is to address this problem through a new executive review process focused on catastrophic risks. OIRA would coordinate an interagency process in which catastrophic risks could be identified and policy alternatives could be evaluated using cost-benefit criteria. This new process would be modeled on prior experiences with OIRA and agency agenda setting. Catastrophic Risk Review could also provide the occasion for the development and reform of cost-benefit analysis methodologies that were tailored to that particular context.
Although cost-benefit analysis and OIRA review has generally been reactive—relying on other processes to set agency agendas and then using cost-benefit analysis to steer agencies on courses that are already set—there are some notable exceptions. Administrations of both political parties have employed OIRA in innovative ways to help prod agencies to action. This more proactive posture for OIRA has met with notable success, and these experiences can help establish a template for a Catastrophic Risk Review process.
An important example of OIRA taking on a more proactive role was the practice of issuing “prompt letters” under the administration of George W. Bush. Carried out during the term of OIRA Administrator John Graham, prompt letters were public requests by OIRA to an agency for some kind of agency action.[ref 40] During the Bush administration a dozen prompt letters were issued on a wide range of issues, including one urging the National Highway Traffic Safety Administration to examine rules to reduce harms from off-center automobile collisions, and another requesting that the Occupational Safety and Health Administration investigate the benefits of requiring automated external defibrillators (AEDs) in the workplace. Perhaps the most successful prompt letter was a request to the Food and Drug Administration to move forward with a labeling requirement for trans-fat content in food.[ref 41] Prompt letters were not always used to promote additional regulation; one important letter issued by Graham encouraged agencies to review a set of several dozen rules that, OIRA argued, imposed inefficient costs.[ref 42]
OIRA undertook an even more substantial and sustained proactive role during the Obama administration. Midway through his first term in office, and with economic effects of the Great Recession still lingering, President Obama issued an executive order requiring agencies to initiate a process of retrospective analysis to identify rules to be “modified, streamlined, expanded, or repealed.”[ref 43] OIRA issued guidance on how to conduct this regulatory lookback, and agencies were given deadlines to submit preliminary plans, collect public feedback, and issue final plans to update, change, or rescind rules.[ref 44] Eventually, agencies identified several hundred initiatives that promised billions of dollars in net savings.[ref 45] This regulatory lookback built on efforts of earlier presidents to coordinate similar government-wide reassessment of the stock of existing rules.[ref 46]
Although neither the prompt letters nor the regulatory lookback generated overwhelming outcomes, they were nonetheless useful innovations that had important successes. It is likely that important rules were adopted, or at least expedited, through the prompt letter process. Similarly, the regulatory lookback redirected agency resources to a reassessment process that identified reforms that likely would have languished. Of course, it is difficult to determine, overall, whether these efforts were wise uses of agency resources. It is possible that by diverting agency attention from other work to respond to these central demands, other even more net beneficial projects were delayed. Indeed, both the prompt letters and the regulatory lookback were responsive in part to the political exigencies of the day: during the Bush administration, to not appear overly anti-regulatory, and during the Obama administration, to push back against claims that agencies were prone to overregulate. Regardless of their overall value, however, prompt letters and regulatory lookback provided a proof of concept that OIRA can use its position in a proactive fashion, in line with cost-benefit principles, when an administration is interested in doing so.
Other processes that provide some precedent for an OIRA-led Catastrophic Risk Review are government-wide guidance documents that the Office has issued. OIRA was the lead coordinator of the interagency process that developed the social cost of carbon, used by agencies across the federal government when estimating the effects of decisions with consequences for greenhouse gas emissions. OIRA also issued Circular A-4, which establishes best practices for conducting cost-benefit analysis across the government. In addition, OIRA is charged with issuing annual reports to Congress on the costs and benefits of federal rulemaking. These reports have historically provided the office with opportunities to highlight important open questions in cost-benefit analysis methodology and risk regulation.
Based on these prior experiences, there are some important features that Catastrophic Risk Review should have. Ideally, as was the case for the regulatory lookback, it should be initiated by the President in an executive order. Presidential leadership can help establish the importance of the process and ensure adequate agency attention. It should also be government-wide and systematic, again similar to the regulatory lookback and social cost of carbon processes. Although the prompt letters were valuable, they were also ad hoc. It was easier to dismiss prompt letters as an artifact of the personal views of a particular OIRA administration, rather than as reflecting a widely shared, cross-administration consensus. OIRA can play a coordinating and oversight role, and OIRA personnel could staff the project, but an interagency coordinating group should also be appointed to elicit expertise and generate buy-in across the executive.
One feature of the prompt letters that Catastrophic Risk Review should adopt is a focus on identifying high net present value undertakings. Although OIRA has an institutional orientation toward net-benefit maximization, there have been instances when the agency was directed to ignore net benefits in favor of other priorities. For example, Trump administration executive orders required agencies to impose no new net costs through regulation, and to rescind two rules for every one rule that they issued. Neither one of these requirements included any language to ensure that they were implemented in such a way as to maximize net benefits. These Trump-era requirements have been criticized as irrational and inconsistent with OIRA’s larger mission.[ref 47] Were Catastrophic Risk Review oriented toward the precautionary principle or some other criteria that was not welfare maximization, there is some risk that it could be viewed similarly as outside OIRA’s purview and conflicting with deep normative principles embedded in the U.S. administrative state.
Prior experience indicated that there should also be a central role for public participation in Catastrophic Risk Review. Two successful cases of integrating public participation into OIRA-led cross-government efforts were in the process of developing Circular A-4, and a public solicitation during the early Obama administration on improving the process of regulatory review. Circular A-4 developed a draft document that was subject to interagency review, public comment, and peer review. This process helped improve and legitimate the document, and the long influence of Circular A-4 helps demonstrate the value of this open and public process. The Obama administration also issued a public call for comments on the process of regulatory review generally, and it received a substantial number of suggestions from a diverse set of commenters. Unfortunately, that process did not ultimately produce substantive reforms, but it nonetheless created a forum to collect innovative ideas from interested parties. By contrast to these two more public processes, the interagency working group on the social cost of carbon was an internal government process that did not subject its work to public comment or peer review. The social cost of carbon has met with some criticism due to the non-public nature of its development. It is likely the case that, at the very least from a legitimacy perspective, the social cost of carbon adopted during the Obama administration would have benefited from opportunities for public comment and peer review.[ref 48]
Given the cross-cutting nature of catastrophic risks, and the reality that many of these issues do not map cleanly onto existing agency mandates, it would be useful for Catastrophic Risk Review to be explicitly oriented to identify a range of government policy options, with potentially different institutional audiences. Prompt letters and regulatory lookback sought to identify agency action—regulatory or deregulatory—and a list of actions for agencies to consider would certainly be a useful output from Catastrophic Risk Review. But the review process could also identify policies that require congressional action, and these findings could be summarized in a document such as OIRA’s annual report to Congress. Catastrophic Risk Review could also identify areas where funding for research through entities like the National Science Foundation would be appropriate, or where assessment reports by bodies such as the National Academy of Sciences could usefully inform policy. Catastrophic Risk Review could also identify areas of cost-benefit analysis methodology that should be updated in light of catastrophic risks.
After an initial period of heightened activity, Catastrophic Risk Review should be incorporated into a long-term, ongoing process of identifying and addressing catastrophic risks. Given the historical inattention to catastrophic risks, there is a substantial backlog of unaddressed issues. The goal of the first stage of Catastrophic Risk Review should be to address this backlog, identify outstanding issues, and offer recommendations for additional measures. Once this initial step has taken place, Catastrophic Risk Review should transition into a more regularized process of information gathering and agency coordination, perhaps punctuated with periodic review processes with more intensive staffing and public participation.
To summarize the process just described, Catastrophic Risk Review would be a government-wide and systematic review of a range of catastrophic risks, including environmental, technological, and socio-economic risks, that was initiated at the presidential level and supervised by OIRA. The review process would include a substantial public participation component and would be charged with examining and assessing a range of policy responses from a cost-benefit perspective and making recommendations to agencies, Congress, and other relevant bodies. Such a process would be a major innovation in how the United States addresses catastrophic risks and would move these issues from the periphery of government decision making toward a more central place in setting the long-term agenda for the U.S. policy process.
As should be clear, such a process would require considerable resources. It bears emphasis that OIRA is already severely stretched in its capacity to address the day-to-day work of the Office. The task of reviewing regulatory proposals from all of the major agencies is extremely demanding. Not only is technical expertise required, but considerable time must be spent coordinating across the White House and executive branch. Simply adding an additional task to OIRA’s already demanding mandate is unlikely to result in a useful and thorough process. Any additional work burden that is placed on OIRA should be accompanied by appropriate resources to ensure that the task can be carried out in a timely and careful manner.
Conclusion
The greatest impediment to the use of cost-benefit analysis to improve the management of catastrophic risks is the reactive nature of regulatory review. In the current regulatory environment, agencies are relatively unlikely to exacerbate catastrophic risks through their actions. Rather, the greatest contribution of the regulatory system to catastrophic harms is through inattention and inaction. Improving cost-benefit analysis methodology, without accompanying procedural reforms, will not remedy this fundamental problem.
This Essay has proposed a new Catastrophic Risk Review process that would focus OIRA and administrative agencies on the task of identifying catastrophic risks and evaluating steps that could be taken to address them. If possible, such a process should be initiated at the highest levels, should be government-wide in scope, should involve substantial public participation, and should contemplate a wide range of risks and potential policy responses. This Catastrophic Risk Review builds on earlier successes at OIRA in prompting regulatory action and coordination activity across the executive.
Although many catastrophic risks have relatively small probabilities, the scale of their harms, were they to come to fruition, are enormous. Human psychology and institutions are ill-suited to recognizing and managing such risks. But methods like cost-benefit analysis, and processes like regulatory review, persist exactly because they complement and mitigate these cognitive and institutional limitations. Cost-benefit analysis can help clarify and debias our understanding of the scale and consequences of catastrophic risks, and the value—and costs—of efforts to manage these risks. But to be useful, even the best cost-benefit analysis methodology must be embedded in a process in which it is carried out and its insights can inform meaningful policy. Catastrophic Risk Review would provide a forum in which the tool of cost-benefit analysis can be put to good use to identify and support sound government policies to address harms that threaten the continued viability of the human project.
Catastrophic uncertainty and regulatory impact analysis
Summary
Cost-benefit analysis embodies techniques for the analysis of possible harmful outcomes when the probability of those outcomes can be quantified with reasonable confidence. But when those probabilities cannot be quantified (“deep uncertainty”), the analytic path is more difficult. The problem is especially acute when potentially catastrophic outcomes are involved, because ignoring or marginalizing them could seriously skewing the analysis. Yet the likelihood of catastrophe is often difficult or impossible to quantify because such events may be unprecedented (runaway AI or tipping points for climate change) or extremely rare (global pandemics caused by novel viruses in the modern world). OMB’s current guidance to agencies on unquantifiable risks is now almost twenty years old and in serious need of updating. It correctly points to scenario analysis as an important tool but it fails to give guidance on the development of scenarios. It then calls for a qualitative analysis of the implications of the scenarios, but fails to alert agencies to the potential for using more rigorous analytic techniques.
Decision science does not yet provide consensus solutions to the analysis of uncertain catastrophic outcomes. But it has advanced beyond the vague guidance provided by OMB since 2003, which may not have been state-of-the-art even then. This paper surveys these developments and explains how they might best be incorporated into agency practice. Those developments include a deeper understanding of potential options and issues in constructing scenarios. They also include analytic techniques for dealing with unquantifiable risks that can supplement or replace the qualitative analysis currently suggested by OMB guidance. To provide a standard framework for discussion of uncertainty in regulatory impact analyses, the paper also proposes the use of a structure first developed for environmental impact statements as a way of framing the agency’s discussion of crucial uncertain outcomes.
Incorporating Catastrophic Uncertainty in Regulatory Impact Analysis
There are well-developed techniques for incorporating possible harmful outcomes into regulatory impact analysis when the probability of those outcomes can be quantified with reasonable confidence. But when those probabilities cannot be quantified, the analytic path is more difficult. The problem is especially acute when potentially catastrophic outcomes are involved, because ignoring or marginally them risks seriously skewing the analysis. Yet the likelihood of a catastrophic is often difficult to quantify because such events may be unprecedented (runaway AI or climate change) or extremely rare (global pandemics in the modern world).
OMB’s current guidance to agencies on this topic is now almost twenty years old and in serious need of updating. It correctly points to scenario analysis as an important tool, but it fails to give guidance on the development of scenarios. It then calls for the a qualitative analysis of the implications of the scenarios, but fails to alert agencies to the potential for using more rigorous analytic techniques.
Decision science does not yet provide consensus solutions to the analysis of uncertain catastrophic outcomes. It has, however, advanced beyond the vague guidance currently provided by OMB, or choosing the alternative with the least-bad possible outcome (maxmin). This paper surveys some of the key developments and describes how they might best be incorporated into agency practice. It is important in doing so to take into account the analytic demands that are already placed on often-overburdened agencies and the variability between agencies in economic expertise and sophistication.
Even at the time OMB’s guidance was issued, there was model language that OMB could have used that would have at least provided a general framework for agencies to use. That language is found in the Council on Environmental Quality’s guidelines for the treatment of uncertainty in environmental impact statements. Using that language as a template has several advantages. Many agencies are familiar with the language, as are courts. Judicial interpretation provides additional guidance on the use of that language. Since agency decisions requiring cost-benefit analysis often also require an environmental assessment or impact statement, creating some parallelism between the NEPA requirements and OMB guidance will also allow the relevant documents to mesh more easily.
The paper proposes modifying this template to make specific reference to some leading techniques provided by decision science. There is a plausible argument that decision makers should simply apply their own intuitions to weighing unquantifiable risks of potential catastrophe. This paper takes another tack for two reasons. First, it is difficult to form judgments about scenarios that are likely to be far outside of the decision maker’s past experience. Whatever analytic help can be offered in making those judgment calls would be beneficial. Second, to have a chance of adoption, proposals should be geared to the technical, economics-oriented perspective espoused by OIRA. Even for those who do not find formalized decision methods adequate, it is better to move the regulatory process in the direction of fuller consideration of potential catastrophic risks rather than having such possibilities swept to the margins of analysis because of a desire for “rigor.”
I. Circular A-4, Uncertainty, and Later Generations
Our starting point is the current OMB guidance on regulatory impact analysis. The section of Circular A-4 begins by discussing uncertainty as a general topic regardless of quantifiability. It then has a short discussion that is specifically focused on unquantifiable uncertainty:
In some cases, the level of scientific uncertainty may be so large that you can only present discrete alternative scenarios without assessing the relative likelihood of each scenario quantitatively. For instance, in assessing the potential outcomes of an environmental effect, there may be a limited number of scientific studies with strongly divergent results. In such cases, you might present results from a range of plausible scenarios, together with any available information that might help in qualitatively determining which scenario is most likely to occur. When uncertainty has significant effects on the final conclusion about net benefits, your agency should consider additional research prior to rulemaking.[ref 1]
The passage then goes on to discuss the possible benefits of obtaining additional information, “especially for cases with irreversible or large upfront investments.”[ref 2] This passage is notably lacking in guidance about how to develop scenarios or what to do with the results.
Circular A-4 also contains a discussion of future generations. It advises:
Special ethical considerations arise when comparing benefits and costs across generations. Although most people demonstrate time preference in their own consumption behavior, it may not be appropriate for society to demonstrate a similar preference when deciding between the well-being of current and future generations. Future citizens who are affected by such choices cannot take part in making them, and today’s society must act with some consideration of their interest. One way to do this would be to follow the same discounting techniques described above and supplement the analysis with an explicit discussion of the intergenerational concerns (how future generations will be affected by the regulatory decision).[ref 3]
The remainder of this discussion of Circular A-4 focuses on the choice of discount rates, primarily arguing for discounting on the ground that future generations will be wealthier than the current generation. The literature on discounting over multiple generations has advanced considerably since then, with a consensus in favor of declining discount rates as a hedge against unexpectedly low economic-welfare in future generations.[ref 4]
The use of discounting assumes, however, that we can quantify risks and their costs in future time periods. In the nature of things, this becomes increasingly difficult over time, as models are extended further beyond their testable results and as society diverges further from historical experience.
In the nearly 20 years since Circular A-4, the state of the art regarding analysis of unquantified uncertainty has made real progress. OMB’s guidance should be improved accordingly.
II. Case Study: Climate Change
The potentially catastrophic multigenerational risks that have been most heavily researched involve global climate change. Climate change is the poster child for the twin problems of uncertainty and impacts on future generations. Impacts on future generations are inherent in the nature of the earth system’s response to radiation forcing by greenhouse gases. Uncertainty is due to the immense complexity of the climate system and the consequent obstacles to modeling, combined with the long time spans involved and the unpredictability of human responses. Thus, climate change presses current methods of risk assessment and management to their limits and beyond.
The IPCC uses a standardized vocabulary to characterize quantifiable risks.[ref 5]
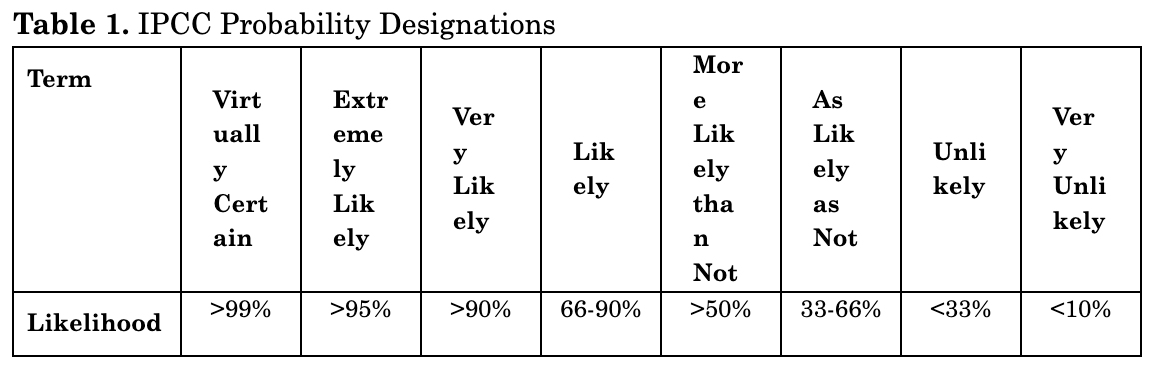
Neither lawyers nor economists have evolved anything similar. The efforts of scientists to provide systemized designations of uncertainty is an indication of the attention they have given to both quantifiable and unquantifiable risks.
As the IPCC explains:
In summary, while high-warming storylines—those associated with global warming levels above the upper bound of the assessed very likely range—are by definition extremely unlikely, they cannot be ruled out. For SSP1-2.6, such a high-warming storyline implies warming well above rather than well below 2°C (high confidence). Irrespective of scenario, high-warming storylines imply changes in many aspects of the climate system that exceed the patterns associated with the best estimate of GSAT [global mean near-surface air temperature] changes by up to more than 50% (high confidence).[ref 6]
High climate sensitivities also increase the potential for warming above the 3 °C level. This, in turn, is linked to possible climate responses whose likelihood is very poorly understood. The IPCC reports a number of potential tipping point possibilities with largely irreversible consequences. Of these, two tipping points are associated with “deep uncertainty” for warming levels above 3°C: collapse of Western Antarctic ice sheets and shelves, and global sea level rise.[ref 7] Uncertainty is also reported as high regarding the potential for abrupt changes in Antarctic sea ice and in the Southern Ocean Meridional Overturning Circulation.[ref 8]
The IPCC also indicates that damage estimates at given levels of warming are also subject to considerable uncertainty:
Projected estimates of global aggregate net economic damages generally increase non-linearly with global warming levels (high confidence). The wide range of global estimates, and the lack of comparability between methodologies, does not allow for identification of a robust range of estimates (high confidence). The existence of higher estimates than assessed in AR5 indicates that global aggregate economic impacts could be higher than previous estimates (low confidence). Significant regional variation in aggregate economic damages from climate change is projected (high confidence) with estimated economic damages per capita for developing countries often higher as a fraction of income (high confidence). Economic damages, including both those represented and those not represented in economic markets, are projected to be lower at 1.5°C than at 3°C or higher global warming levels (high confidence).[ref 9]
Economists might view this assessment of the state of the art in modeling climate damages as too harsh. Nevertheless, it seems clear that we cannot assume that present estimates accurately represent the true probability distribution of possible damages.
The possibility of catastrophic tipping points looms large in economic modeling of climate change. Another key issue in terms of the economic analysis is the possibility of unexpectedly bad outcomes, such major melting of ice sheets, releases of large amounts of methane, and halting of the Gulf Stream. [ref 10] William Nordhaus, who pioneered the economic models of climate change, has explained how these affect the analysis:
[W]e might think of the large-scale risks as a kind of planetary roulette. Every year that we inject more CO2 into the atmosphere, we spin the planetary roulette wheel. . . .
A sensible strategy would suggest an insurance premium to avoid the roulette wheel in the Climate Casino. . . . We need to incorporate a risk premium not only to cover the known uncertainties such as those involving climate sensitivity and health risks but also … uncertainties such as tipping points, including ones that are not yet discovered.[ref 11]
The difficulty, as Nordhaus admits, is trying to figure out the extent of the premium. Another recent book by two leading climate economists argues that the downside risks are so great that “the appropriate price on carbon is one that will make us comfortable enough to know that we will never get to anything close to 6 °C (11 °F) and certain eventual catastrophe.”[ref 12] Although they admit that “never” is a bit of an overstatement—reducing risks to zero is impractical—they clearly think it should be kept as low as feasibly possible. Not all economists would agree with that view, but there seems to be a growing consensus that the possibility of catastrophic outcomes should play a major role in determining the price on carbon.[ref 13]
Scientists are beginning to gain greater knowledge about long-term climate impacts after the end of this century. The IPCC reports advances in modeling up through 2300. Under all but the lowest emissions scenarios, global temperatures continue to rise markedly through the 22nd century.[ref 14] Moreover, “[s]ea level rise may exceed 2 m on millennial time scales even when warming is limited to 1.5°C–2°C, and tens of metres for higher warming levels.”[ref 15] Indeed, “physical and biogeochemical impacts of 21st century emissions have a potential committed legacy of at least 10,000 years.”[ref 16]
To provide some perspective of the scale of the potential changes:
To place the temperature projections for the end of the 23rd century into the context of paleo temperatures, GSAT [global surface air temperature] under SSP2-4.5 (likely 2.3°C–4.6°C higher than over the period 1850–1900) has not been experienced since the Mid Pliocene, about three million years ago. GSAT projected for the end of the 23rd century under SSP5-8.5 (likely 6.6°C–14.1°C higher than over the period 1850–1900) overlaps with the range estimated for the Miocene Climatic Optimum (5°C–10°C higher) and Early Eocene Climatic Optimum (10°C–18°C higher), about 15 and 50 million years ago, respectively (medium confidence)[ref 17]
The current emission pathway seems unlikely to produce the second, more severe scenario (though “unlikely” does not mean impossible). But the first scenario (SSP2-4.5) represents a middle-of-the road future in which global emissions peak around 2050 and decline through 2100.[ref 18] Even that scenario could put the world well out of the range of what homo sapiens has ever experienced, making predictions about future damages inherently uncertain.
III. Decisions Methods under Deep Uncertainty
It is one thing to perceive the significance of potential catastrophic risks; it is another to incorporate them into the analysis in a useful way. The precautionary principle is one effort to do so in a qualitative way, though the principle is controversial, particularly among economists. Use of precaution in the case of catastrophic risks has had some support even from Cass Sunstein, a leading critic of the precautionary principle.[ref 19] Sunstein has proposed a number of different versions of the catastrophic risk precautionary principle, in increasing order of stringency.[ref 20] The first required only that regulators take into account even highly unlikely catastrophes.[ref 21] Another version “asks for a degree of risk aversion, on the theory that people do, and sometimes should, purchase insurance against the worst kinds of harm.”[ref 22] Hence, Sunstein said, “a margin of safety is part of the Catastrophic Harm Precautionary Principle—with the degree of the margin depending on the costs of purchasing it.”[ref 23] Finally, Sunstein suggested, “it sometimes makes sense to adopt a still more aggressive form of the Catastrophic Harm Precautionary Principle, one “selecting the worst-case scenario and attempting to eliminate it.”[ref 24] More recently, Sunstein has endorsed use of maxmin in situations involving catastrophic risks, with some suggestions for possible guardrails to ensure that this test is applied sensibly.[ref 25] As Sunstein’s effort illustrates, it may be possible to clarify the areas of application for the precautionary principle sufficiently to make the principle a workable guide to decisions.
Maximin is a blunt tool, however, particularly in cases where precaution is very expensive in terms of resource utilization or foregone opportunities. There are variations on this principle and other possible tools for dealing with nonquantifiable risks.[ref 26] “Ambiguity” is a term that is often used to refer to situations in which the true probability distribution of outcomes is not known.[ref 27] There is strong empirical evidence that people are averse to ambiguity. The classic experiment involves a choice between two urns. One is known to contain half red balls and half blue; the other contains both colors but in unknown proportions. Regardless of which color they are asked to bet on, most individuals prefer to place their bet on the urn with the known composition.[ref 28] This is inconsistent with standard theories of rational decision making: if the experimental subjects prefer the known urn when asked to bet on red, that implies that they think that there are fewer than fifty percent red balls in the other urn. Consequently, they should prefer the second urn when asked to bet on blue—if it is less than half red it must be more than half blue—but they do not. Apparently, people prefer not to bet on an urn of uncertain composition.[ref 29] Such aversion to ambiguity “appears in a wide variety of contexts.”[ref 30] Ambiguity aversion may reflect a sense of lacking competence to evaluate a gamble.[ref 31]
Ambiguity aversion may lead to irrational decision in some settings like laboratory experiments in which subjects know with certainty what scenarios are possible and their contents, it may be a much more reasonable attitude in practice. If we have two models of a situation available with completely different implications, that suggests that we do not understand the dynamics of the situation. That in turn means that the situation could be different from both models in some unknown way, and that whatever process the two models are trying to represent is really unknown to us. In the stylized example, perhaps there are green balls in the urn as well, or maybe the promised payoffs from our gamble won’t appear. To take an example from foreign relations, it is one thing to deal with a leader who has a track record of being capricious and unpredictable (a situation involving risk); it is another to have no idea of who is leading the country on any given day (deep uncertainty). Our ability to plan for the future is limited in such situations, and it is reasonable to want to avoid being put in that position.
There are a number of different approaches to modeling uncertainty about the true probability distribution.[ref 32] One is the Klibanoff-Marinacci-Mukerji model.[ref 33] This approach assumes that decision makers are unsure about the correct probability. Their decision is based on (a) the likelihood that the decision maker attaches to different probability distributions, (b) the degree to which the decision maker is averse to taking chances about which probability distribution is right, and (c) the expected utility of a decision under each of the possible probability distributions. In simpler terms, the decision maker combines the expected outcome under each probability distribution according to the decision maker’s beliefs about the distributions and attitude toward uncertainty regarding the true probability distribution. The shape of the function used to create the overall assessment determines in a straightforward way whether the decision maker is uncertainty averse, uncertainty neutral, or uncertainty seeking.[ref 34]
The Klibanoff-Marinacci-Mukerji model has an appealing degree of generality. But this model is not easily applied because the decision maker needs to be able to attach numerical weights to the specific probability distributions, which may not be possible in cases of true uncertainty where the possible distributions are themselves unknown.[ref 35] The model fits best with situations where our uncertainty is fairly tightly bounded: we know all of the possible models and how they behave, although we are unsure which one is correct.
Other models of ambiguity are more tractable and apply in situations where uncertainty may run deeper. As economist Sir Nicholas Stern explained, in these models of uncertainty, “the decision maker, who is trying to choose which action to take, does not know which of [several probability] distributions is more or less likely for any given action.”[ref 36] He explains that it can be shown the decision maker would “act as if she chooses the action that maximises a weighted average of the worst expected utility and the best expected utility …. The weight placed on the worst outcome would be influenced by concern of the individual about the magnitude of associated threats, or pessimism, and possibly any hunch about which probability might be more or less plausible.”[ref 37]
These models are sometimes called α-maxmin models, with α representing the weighting factor between best and worst cases.[ref 38] One way to understand these models is that we might want to minimize our regret for making the wrong decision, where we regret not only disastrous outcomes that lead to the worst case scenario, but also we regret having missed the opportunity to achieve the best case scenario. Alternatively, a can be a measure of the balance between our hopes (for the best case) and our fears (of the worst case).
Applying these α-maxmin models as a guide to action leads to what we might call the α-precautionary principle. Unlike most formulations of the precautionary principle, α-precaution is not only aimed at avoiding the worst case scenario; it also involves precautions against losing the possible benefits of the best case scenario.[ref 39]1 In some situations, the best case scenario is more or less neutral, so that α-maxmin is not much different from pure loss avoidance, unless the decision maker is optimistic and uses an especially low alpha. But where the best case scenario is potentially extremely beneficial, unless the decision maker’s alpha is very high, α-precaution will suggest a more neutral attitude toward uncertainty in order to take advantage of potential upside gains.
For example, suppose we have two models about what will happen if a certain decision is made. We assume that each one provides us enough information to allow the use of conventional risk assessment techniques if we were to assume that the model is correct. For instance, one model might have an expected harm of $1 billion and a variance of $0.2 billion; the other an expected harm of $10 billion and a variance of $3 billion. If we know the degree of risk aversion of the decision maker, we can translate each outcome into an expected utility figure for each model. The trouble is that we do not know which model is right, or even the probability of correctness. Hence, the situation is characterized by uncertainty. To assess the consequences associated with the decision, we then use a weighted average of these two figures based on our degree of pessimism and ambiguity aversion. This averaging between models allows us to compare the proposed course of action with other options.
An interesting variant of α-maxmin uses a weighted average that includes not only the best case and worst case scenarios, but also the expected value of the better understood, intermediate part of the probability distribution.[ref 40] This approach “is a combination between the mathematical expectation of all the possible outcomes and the most extreme ones.”[ref 41] This tri-factor approach may be “suitable for useful implementations in situations that entangle both more reliable (‘risky’) consequences and less known (‘uncertain’), extreme outcomes.”[ref 42] However, this approach requires a better understanding of the mid-range outcomes and their probabilities than does α-maxmin.
We seem to be suffering from an embarrassment of riches, in the sense of having too many different method for making decisions in situations in which extreme outcomes weigh heavily. At present, it is not clear that any one method will emerge as the most useful for all situations. For that reason, the ambiguity models should be seen as providing decision makers with a collection of tools for clarifying their analysis rather than providing a clearly defined path to the “right” decision.
Among this group of tools, α-maxmin has a number of attractive features. First, it is complex enough to allow the decision maker to consider both the upside and downside possibilities, without requiring detailed probability information that is unlikely to be available. Second, it is transparent. Applying the tool requires only simple arithmetic. The user must decide on what parameter value to use for α, but this choice is intuitively graspable as a measure of optimism versus pessimism. Third, α-maxmin can be useful in coordinating government policy. It is transparent to higher level decision makers and thus suited to central oversight.
Rather than asking the decision maker to assess highly technical probability distributions and modeling, α-maxmin simply presents the decision maker with three questions to consider: (1) What is the best case outcome that is plausible enough to be worth considering? (2) What is the worst case scenario that is worth considering? (3) How optimistic or pessimistic should we be in balancing these possibilities?[ref 43] These questions are simple enough for politicians and members of the public to understand. More importantly, rather than concealing value judgments in technical analysis by experts, they present the key value judgments directly to the elected or appointed officials who should be making them. Finally, these questions also lend themselves to oversight by outside experts, legislators, and journalists, which is desirable in societal terms even if not always from the agency’s perspective.
A. Identifying Robust Solutions
Another approach, which also finds its roots in consideration of worst case scenarios, is to use scenario planning to identify unacceptable courses of action and then choose the most appealing remaining alternatives.[ref 44] Robustness rather than optimality is the goal. RAND researchers have developed a particularly promising method to use computer assistance in scenario planning.[ref 45] RAND’s Robust Decision Making (RDM) technique provides one systematic way of exploring large numbers of possible policies to identify robust solutions.[ref 46] As one of its primary proponents explains:
RDM rests on a simple concept. Rather than using computer models and data to describe a best-estimate future, RDM runs models on hundreds to thousands of different sets of assumptions to describe how plans perform in a range of plausible futures. Analysts then use visualization and statistical analysis of the resulting large database of model runs to help decisionmakers distinguish future conditions in which their plans will perform well from those in which they will perform poorly. This information can help decisionmakers identify, evaluate, and choose robust strategies — ones that perform well over a wide range of futures and that better manage surprise.[ref 47]
During each stage of the analysis, RDM uses statistical analysis to identify policies that perform well over many possible situations.[ref 48] It then uses datamining techniques to identify the future conditions under which such policies fail. New policies are then designed to cope with those weaknesses, and the process is repeated for the revised set of policies. As the process continues, policies become robust under an increasing range of circumstances, and the remaining vulnerabilities are pinpointed for decision makers.[ref 49] More specifically, “RDM uses computer models to estimate the performance of policies for individually quantified futures, where futures are distinguished by unique sets of plausible input parameter values.”[ref 50] Then, “RDM evaluates policy models once for each combination of candidate policy and plausible future state of the world to create large ensembles of futures.”[ref 51] The analysis “may include a few hundred to hundreds of thousands of cases.”[ref 52]
The process could be compared with stress-testing various strategies to see how they perform under a range of circumstances. There are differences, however. RDM may also consider how strategies perform under favorable circumstances as well as stressful ones; it is able to test a large number of strategies or combination of strategies; and strategies are often modified (for instance by incorporating adaptive learning) as a result of the analysis.
A related concept, which discards strategies known to be dangerous, is known as the safe minimum standards (SMS) approach.[ref 53] This approach may apply in situations in which there are discontinuities or threshold effects, but there is considerable controversy about its validity.[ref 54] A related variant is to impose a reliability constraint, requiring that the odds of specified bad outcomes be kept below a set level.[ref 55] The existence of threshold effects makes information about the location of thresholds quite valuable. For instance, in the case of climate change, a recent paper estimates that the value of early information about climate thresholds could be as high as three percent of gross world product.[ref 56]
B. Scenario Construction
The RDM methodology and α-maxmin are technical overlays on the basic idea of scenario analysis. Robert Verchick has emphasized the importance of scenario analysis—and of the act of imagination required to construct and consider these scenarios—in the face of nonquantifiable uncertainty.[ref 57] As he explained, scenario analysis avoids the pitfall of projecting a single probable future when vastly different outcomes are possible; broadens knowledge by requiring more holistic projections; forces planners to consider changes within society as well as outside circumstances; and, equally importantly, “forces decision-makers to use their imaginations.”[ref 58] He added that the “very process of constructing scenarios stimulates creativity among planners, helping them to break out of established assumptions and patterns of thinking.”[ref 59] In situations in which it is impossible to give confident odds on the outcomes, scenario planning may be the most fruitful approach.[ref 60]
There is now a well-developed scholarly literature about the construction and uses of scenarios.[ref 61] By 2010, it could be said that “combining rich qualitative storylines with quantitative modeling techniques, known as the storyline and simulation approach, has become the accepted method for integrated environmental assessment and has been used in all major assessments including those by the IPCC, Millennium Ecosystem Assessment, and many regional and national studies.”[ref 62] Storylines “describe plausible, but alternative socioeconomic development pathways that allow scenario analysts to compare across a range of different situations, generally from 20 to 100 years into the future.”[ref 63] Because our limited ability to predict the future evolution of society on a multi-decadal basis (let along multi-century), scenarios are a particularly useful technique in dealing with problems involving long time spans and future generations. Best practices for constructing scenarios have also emerged. They include “pooling independently elicited judgments, extrapolating trend lines, and looking through data through the lenses of alternative assumptions.”[ref 64]
Within the scenario family, however, are several different subtypes, varying in the process for developing the scenario, whether the scenario is exploratory or designed to exemplify the pathway to a given outcome (such as achieving the Paris Agreements goals), or informal as opposed to probabilistic.[ref 65]
Use of scenarios in the context of climate change has been a particular subject of attention.[ref 66] The IPCC has developed one set of scenarios (the SSP scenarios[ref 67]) for future pathways of societal development. Roughly speaking, these scenarios differ in the amount of international cooperation and whether society is stressing economic growth or environmental sustainability.[ref 68] The SSP scenarios include detailed assumptions about population, health, education, economic growth, inequality and other factors.[ref 69] A different set of scenarios, the RCPs,[ref 70] are used to model climate impacts based on different future trajectories of GHG concentrations. Thus, “the RCPs generate climate projections that are not interpreted as corresponding to specific societal pathways, while the SSPs are alternative futures in which no climate impacts occur nor climate policies implemented.”[ref 71] Integrated models are then used to combine the socioeconomic storylines, emissions trajectories, and climate impacts into a single simulation.[ref 72] One significant finding is that only some scenarios are compatible with achieving the Paris Agreemen’st aim of keeping warming to 1.5°C.[ref 73] One advantage of the IPCC approach is that it provides standardized scenarios that can be used by many researchers looking at many different aspects of climate change.
There seems to be no one “right” way to construct scenarios. As with the assessment methods discussed above, the most important thing in practice may be for the agency to explain the options and the reasons for choosing one approach over the others. It might also be useful for agencies to standardize their scenarios where possible, which would create economies of scale in scenario development, allow comparison of results across different regulations, and provide focal points for researchers.
Conclusion
Current guidance to agencies treats situations of deep uncertainty — those situations where we cannot reliably quantify risks — as an afterthought. This is particularly unfortunate in terms of catastrophic risks. Such risks are generally rare, meaning a sparse history of prior events (which may be completely lacking when novel threats are involved.) They often involve complex dynamics that make prediction difficult. The more catastrophic the event, the more likely it is to have impacts across multiple generations, changing the future in ways that are hard to predict. Yet planning only for better understood, more predictable risks may blind regulators to crucial issues. Catastrophic risks, even when they seem remote before the fact, may matter far more than the more routine aspects of a situation, in the spirit of “Except for that, how did you like the play, Mrs. Lincoln?” Fortunately, devastating pandemics, economic collapses, environmental tipping points, and disastrous runaway technologies are not the ordinary stuff of regulation. But where such catastrophes are relevant, marginalizing their consideration is dangerous.
There is no clear-cut answer to how we should integrate consideration of potential catastrophic outcomes into regulatory analysis. However, two decades after the last OMB guidance, we have a clearer understanding of the available analytic tools and better frameworks for applying them. If we cannot expect agencies to get all the answers right, we can at least expect them to carefully map out the analytic techniques they have used and why they have chosen them. This paper has explained the range of tools available and called for a revamping of OMB guidance to give clearer direction to agencies on their use. Even imperfect tools are better than relegating the risk of catastrophe to the shadows in regulatory decision making.
Catastrophic risk, uncertainty, and agency analysis
Summary
I propose three changes to the governance of federal policymaking: (1) an amendment to Circular A-4 that provides agencies with guidance on evaluating policies implicating catastrophic and existential risks, (2) principles for the assessment and mitigation of those risks, and (3) proposed language to be included in an executive order requiring agencies to report on such risks.
Each proposal is intended to serve a different goal, and each adopts policy choices that are explained in detail in the accompanying essay. First, the goals. The amendment to Circular A-4 aims to help agencies produce robust assessments of proposed agency action that implicates catastrophic and existential risks. The amendment balances two dangers: on the one hand, allowing agencies to taking risky actions without carefully considering their effects on potential catastrophic and existential risks; and on the other, requiring implausibly definite justifications for catastrophic-risk-mitigation policies when those policies will inevitably involve high levels of uncertainty. A related and important distinction is between agency action designed to reduce the threat of catastrophic public harms or human extinction and agency action that is taken for some other purpose but that may nevertheless affect catastrophic and existential risks.
Circular A-4 is important, but limited. It covers only a subset of agency action—official agency rulemakings. The proposed principles sweep more broadly. They encourage all departments and agencies to actively focus on catastrophic and existential risks and to apply the best practices of Circular A-4, including the use of quantitative estimates combined with qualitative analysis, beyond the scope of agency rulemakings.
The principles are broad, but non-binding. My final proposal, therefore, is for an executive order (EO) requiring agencies to produce reports on relevant catastrophic and existential risks, including the state of relevant expert knowledge and proposals for executive or legislative action. The proposed language is designed to be included in an EO implementing President Biden’s January 2021 memorandum on modernizing regulatory review.
Each of the proposals reflects policy choices that I explain in the accompanying essay. They divide into two areas: how to make sure federal agencies do not neglect catastrophic and existential risks and how to properly evaluate policies related to those risks.
The proposed principles and EO concern to the former problem, agency agenda-setting. Agencies are influenced explicitly by legislative mandates and more subtly by presidential policy preferences and external political pressures, such as public opinion, interest groups, and media coverage. The White House does not, in general, direct day-to-day agency action, but it can influence agency priorities and shape high-profile decisions. To that end, the principles and the EO are designed to get catastrophic and existential risks on the agency agenda—and to produce, through public reports, congressional attention and external political pressure.
The amendment to Circular A-4, for their part, reflect two major choices. First, it instructs agencies to apply quantified benefit-cost analysis (BCA) to policies implicating catastrophic and existential risks, despite a competing school of thought that calls for the use of the precautionary principle in such circumstances. The essay considers the arguments in favor of the latter approach in some detail, in particular the problems of uncertainty associated with extreme risks, but ultimately rejects the precautionary principle as theoretically unjustified, practically indeterminate, and, given the entrenched nature of BCA, politically implausible. Second, the amendment acknowledges the limits of quantitative analysis in this area and calls for agencies to offer, alongside quantitative BCA, robust qualitative justifications for policies affecting catastrophic and existential risks. As the essay explains, the problems of fat-tailed distributions and expected value calculations that rely on low probabilities of extremely large costs or benefits should lead policymakers to be wary of relying solely on the numbers.
Introduction
Imagine a policymaker who is confronted with a new technology—artificial intelligence, say—or a field of research—into novel pathogens, perhaps—that some experts warn could pose a catastrophic, even existential, risk. What regulations should she propose? Nothing? A total ban? Safety limits? Which ones? What if experts disagree vociferously on whether disaster is likely, or even possible?
Faced with such difficulties, a common policy response is simply to ignore the problem.[ref 1] Some of the models of climate change the federal government uses to calculate its social cost of carbon do not consider extreme outcomes from global warming. There are no public regulatory cost-benefit assessments that attempt to calculate the value of pandemic mitigation, the regulation of misaligned or hostile general artificial intelligence, or the prevention of bioterrorist attacks. The Supreme Court has allowed agencies to round down small and uncertain risks of disaster to zero under some circumstances.[ref 2]
There are many explanations for the tendency to neglect low probability, high impact risks. Psychologists point to biases in human thinking, such as our tendency to ignore small probabilities and events that we have not experienced.[ref 3] Economists note that policies to mitigate climate change or prevent pandemics are public goods—that is, those paying for them will not capture all the benefits, so everyone has an incentive to free-ride on the efforts of others—and thus the market will not supply them.[ref 4] Political scientists point out that voters will not reward politicians for spending money today to ward off disaster in half a century, so the rational leader will always pass the buck to her successor.[ref 5] Whichever explanation is true—and they all likely have some merit—that neglect is a mistake. Its tragic consequences were revealed by the Covid-19 pandemic. It may bring worse yet.
On January 20, 2021, President Biden issued a memorandum titled “Modernizing Regulatory Review.”[ref 6] The memorandum directed the head of the Office of Management and Budget (OMB) to produce recommendations for updating the regulatory review process and “concrete suggestions” for how it could promote several values, including “the interests of future generations.”[ref 7]
This essay suggests that one way to fulfill the Biden memorandum’s promise to protect future generations is for policymakers to address catastrophic-risk neglect within the federal government. To that end, this essay proposes three things: First, amending OMB’s Circular A-4, the “bible” of the regulatory state,[ref 8] to include an explicit discussion of catastrophic and existential risks. Second, a set of guiding principles for the assessment and mitigation of such risks.[ref 9] And finally, a proposed executive order (EO) requiring agencies to affirmatively consider and report on relevant catastrophic and existential risks. Broadly speaking, the principles and proposed EO aim to get agencies thinking about, and responding to, catastrophic risks, and the amendment to Circular A-4 aims to guide the evaluation of relevant actions once they are proposed. The principles are about agency agenda-setting and Circular A-4 is about the agency analytical process.
This essay proceeds in three Parts. Part I sets the stage by outlining the problem of catastrophic and existential risk. Part II explains the agency agenda-setting process and how the proposed principles and EO aim to influence it, as well as how both are designed to force agencies to give explicit reasons for their action or inaction. Part III contains a theoretical justification for the choices I have made in the amendment to Circular A-4. In particular, it justifies the reliance on quantified benefit-cost analysis (BCA) rather than the precautionary principle while acknowledging the need for qualitative analysis to accompany BCA in areas of extreme risk and high uncertainty.
I. Catastrophes
Before diving into the details of my proposals, a brief survey of catastrophic risks is in order.[ref 10] Although legal scholars have written extensively about the threat of catastrophe, the term has a flexible meaning, covering everything from events that threaten truly global destruction, such as pandemics and nuclear war, to others, such as terrorism, nuclear power plant accidents, and extreme weather events, that cause more limited devastation. One scholar has suggested that a disaster that kills ten thousand people would not qualify as a catastrophic risk, while one that killed ten million people would.[ref 11] The Covid-19 pandemic—current estimated death toll, 22 million[ref 12]—would thus count, and pandemics in general are a prominent catastrophic risk. Here, I use the term catastrophic risk narrowly, to refer to threats that have the potential to kill at least tens of millions of people.
Experts divide catastrophic risks into several categories, such as natural risks, anthropogenic risks, and future risks.[ref 13] Natural risks include pandemics, supervolcanoes, massive asteroid or meteor strikes, and more remote possibilities such as a supernova close to our solar system. Anthropogenic risks include nuclear war, climate change, and other kinds of human-driven environmental damage. Future risks are those that have not seriously threatened humanity’s survival yet but could do so in the future, thanks to societal or technological changes. These include biological warfare and bioterrorism, artificial intelligence, and technologically enabled authoritarianism. Although some individual risks may seem outlandish (and even for the more mainstream ones, such as climate change, extreme outcomes are quite unlikely), taken together, they pose a significant threat to humanity’s future.
I note here another important distinction: between catastrophic and existential risks. There is a major difference between a catastrophe that kills 100 million people and one that causes human extinction—even between the death of several billion people and the death of everyone. Some researchers focus almost entirely on so-called existential risks, arguing that the destruction of humanity’s entire future would be far worse even than a disaster that killed most people but left the possibility of recovery.[ref 14] They have a least a plausible argument, but I will here consider catastrophic and existential risks together. For my purposes, the same analysis applies to both, as there is considerable overlap between the two. After all, several existential risks, such as nuclear war, biological weapons, artificial intelligence, and extreme climate change, are also catastrophic risks.[ref 15] And policies that mitigate the risk of catastrophe will generally also guard against the risk of extinction. The two areas raise largely the same questions of public policy; often, only the numbers are different.
The literature on catastrophic risks is large and much of it concerns the details of individual risks, which I will not attempt to summarize here.[ref 16] When addressing catastrophic risk as a whole, the scholarship makes three basic contentions: (1) humanity has acquired, in the past half century, the ability to destroy itself, or at least severely degrade its future potential;[ref 17] (2) human extinction or collapse would be an almost unimaginable catastrophe;[ref 18] and (3) we can take concrete actions to quantifiably reduce the risk of disaster.[ref 19] Each of these claims is plausible, although not uncontested, and together they add up to the conclusion that humanity should devote significantly more of its resources to mitigating the most extreme risks it faces.
II. Agency Agenda-Setting
If catastrophic and existential risks are neglected by the federal government, how can agencies be encouraged to focus on them? In general, three actors have the most sway over agency attention: Congress, the public, and the White House. I focus on the executive branch here, but many of the same ideas could be applied to potential legislative action. Around a third of agency regulations are the result of explicit congressional requirements.[ref 20] Most of the remainder are workaday updates to prior regulations. Perhaps surprisingly, the White House appears to have little express influence over agency agenda-setting. The most explicit formal requirements come in the Reagan-era Executive Order 12,866, which requires agencies to hold annual priority-setting meetings, creates a regulatory working group to plan agency action, and forces agencies to submit information about their plans to the Office of Information and Regulatory Affairs (OIRA).[ref 21] The EO’s requirements, however, appear to have little practical effect, more “rote” rules than substantive influence on agency policy setting.[ref 22]
The White House does display influence in some areas. Apart from the President’s obvious role in selecting the heads of departments and agencies, the White House has a much greater influence over the creation and content of the most politically significant agency rules than over day-to-day agency action, much of which is probably of little interest to the President. [ref 23] The White House can also shape how agencies go about their business by mandating rules of agency management.[ref 24] Both President Obama and President Trump issued executive orders designed to shape the agency rulemaking process, including by specifying the kinds of evidence agencies could consider, setting reporting requirements, and conducting retrospective reviews of existing rules.[ref 25] During the Obama administration, the White House’s Office of Science and Technology Policy and OIRA also issued a set of non-binding principles designed to shape regulatory policy for “emerging technologies.”[ref 26]
My proposed Principles for the Assessment and Mitigation of Catastrophic and Existential Risks, along with the proposed section of an EO, aim to play a similar role. The principles encourage agencies to affirmatively consider how they can mitigate catastrophic and existential risks related to their area of regulatory focus. They suggest that agencies develop a register of relevant risks and seek opportunities to address them. They also instruct agencies not to ignore a risk purely because it falls below an arbitrary probability threshold, something that is not unusual in some agencies.[ref 27]
The proposed EO language is designed to be included in an order implementing President Biden’s 2021 memorandum on modernizing regulatory review. The order would require each agency, if relevant, to submit to OIRA an annual Catastrophic and Existential Risk Plan that includes an assessment of risks relevant to the agency, expert estimates of the probability and magnitude of the threats along with associated uncertainties, and proposals for risk mitigation by the agency or the wider federal government. This last might include planned or proposed agency regulations or recommendations to Congress for legislative action. Along with the principles, the proposed EO is intended both to inform policymakers in OMB and the White House and to make sure that agencies pay attention to extreme risks, both in affirmatively developing proposals to mitigate them and in considering other agency actions that might implicate extreme risks.
III. Guiding Agency Policy Evaluation
Once the White House or OMB has succeeded in getting agencies to pay attention to extreme risks, a second problem arises: How should agencies deal with the tricky analytical questions they raise? Under EO 12,866 and Circular A-4, agencies are required to evaluate proposed regulations using either cost-effectiveness analysis (CEA) or benefit-cost analysis (BCA). The two areas encompass a wide range of approaches, but in short, CEA involves setting a pre-defined target, such as a level of emissions reduction, and determining the most cost-effective method of reaching it. BCA, on the other hand, takes a proposed agency action and evaluates its costs and benefits de novo; a regulation passes BCA only if the benefits exceed the costs.
In both cases, catastrophic and existential risks raise difficult issues. As this Part explains, such risks typically involve high levels of uncertainty. This uncertainty is often given as a justification for adopting a version of the precautionary principle to replace or supplement CEA or BCA.[ref 28] My proposed amendment to Circular A-4 does not adopt the precautionary principle, for two reasons: first, whatever the precautionary principle’s benefits in other situations, it is theoretically unjustified and practically indeterminate when dealing with extreme risks, and second, BCA has become so deeply entrenched in regulatory analysis that attempting to replace it is likely a fool’s errand.
Extreme risks, however, also reveal the limits of quantitative analysis. The proposed amendment highlights the need to consider “fat tails” in BCA and the importance of including a rigorous qualitative analysis when the BCA assessment relies on uncertain estimates of very low probabilities of very large costs or benefits.[ref 29] This Part explains those choices and provides more detail on the guidelines for evaluating policy for extreme risks.
Along with the principles and the EO, the amendment’s focus on dual quantitative and qualitative analysis draws on a common theme in administrative law: the importance of reason-giving. Requiring decision makers to explain themselves, as Ashley Deeks has written, can improve decisions, promote efficiency, constrain policy choices, increase public legitimacy, and foster accountability.[ref 30] In the extreme risk context, a requirement that agencies justify their decisions both through traditional BCA and through qualitative explanations should encourage agencies to think through their choices in more detail, consult more closely with outside experts, and receive greater congressional and public input into their decisions.
A. Uncertainty
This Section considers, and rejects, one of the strongest objections to BCA: the problem of “deep uncertainty.” Deep uncertainty, I argue, is an incoherent concept in practical policymaking and thus poses no support for the use of the precautionary principle and no obstacle to the use of BCA.
i. Explaining Deep Uncertainty
Every policy decision involves risk. From setting flood wall requirements to imposing mask mandates, regulators must weigh the probabilities of competing benefits and harms. No outcomes are guaranteed. CEA and BCA recognize this and require modelling and quantifying the relevant risks.
But what if the uncertainty is so great that the probabilities are unquantifiable? Some decision theorists distinguish “deep uncertainty” (where numerical probabilities cannot be given) from risk (where they can).[ref 31] Under deep uncertainty, BCA cannot be undertaken.[ref 32]
A famous intuitive explanation of deep uncertainty comes from John Maynard Keynes, who suggested that there are some events for which “there is no scientific basis on which to form any calculable probability whatever.”[ref 33] “The sense in which I am using the term [uncertainty],” Keynes wrote in 1937, “is that in which the prospect of a European war is uncertain, or the price of copper and the rate of interest twenty years hence, or the obsolescence of a new invention, or the position of private wealth owners in the social system in 1970.”[ref 34] On such questions, Keynes argued, we do not merely have low confidence in our probability estimates; we are unable to come up with any numbers at all.
If deep uncertainty exists, catastrophic risks seem especially likely to be subject to it. Climate change is a common example. Long term climate projections involve several steps, each of them subject to doubt. First, scientists must predict future emissions of carbon dioxide and other greenhouse gasses. Then they must create models of the climate that can estimate how those emissions will affect global temperatures and weather patterns. Those outputs must then be fed into yet more models to translate changes in the climate into changes in economic growth, human health, and other social outcomes. Some experts suggest that the final numbers, in the form of economic damages from higher temperatures, or, for example, the U.S. government’s social cost of carbon, are “little more than a guess.”[ref 35] The economists John Kay and Mervyn King believe that deep uncertainty applies to catastrophic risks across the board: “to describe catastrophic pandemics, or environmental disasters, or nuclear annihilation, or our subjection to robots, in terms of probabilities is to mislead ourselves and others.”[ref 36] We can, they say, “talk only in terms of stories.”[ref 37]
Where numbers fail, one option is to turn to the precautionary principle. The principle, which has gained significant popularity in international and environmental law, comes in many forms, but most of them boil down to a single idea: act aggressively to ward off extreme threats even when their probability cannot be known.[ref 38] This apparently bright-line rule cuts through the messiness of BCA to give policymakers an immediate answer. Better to build in a margin of safety, the thinking goes, by moving to avert catastrophe than trust models whose reliability we cannot judge until it is too late.
ii. Rejecting Deep Uncertainty
Yet the concept of deep uncertainty has come under withering critique from economists. Milton Friedman, for example, argued that Knight’s distinction between risk and uncertainty was invalid. Even if people decline to quantify risks, Friedman noted, “we may treat [them] as if they assigned numerical probabilities to every conceivable event.”[ref 39] People may claim that it is impossible to put a probability on a terrorist attack on the New York subway tomorrow,[ref 40] but they go to work nonetheless; if they thought the probability was anything other than negligible, they would surely refuse. People must decide whether to take a flight, go to a café during a pandemic, or cross the road before the light has changed. In each case, they are making a probability determination, even if they don’t admit it.[ref 41]
An extension of this line of thinking, known as a Dutch book argument, attempts to demonstrate that refusing to assign probabilities is irrational.[ref 42] The idea is that one can extract probabilities on any given question even from those who deny they have them by observing which bets on the issue they will and won’t accept. A rational person, so the argument goes, should always be willing to take one side of a bet if she thinks she can win. But if she agrees to a set of bets that together violate the axioms of probability theory, whoever takes the other side will make a Dutch book against her—that is, make money off her. And following the axioms requires keeping track of one’s numerical predictions (to make sure they sum to one in the appropriate places, and so on).
A central premise of the Dutch book argument—that any rational agent will be willing to take at least one side of any bet—has struck many commentators as far-fetched.[ref 43] A natural response to decision theorists proposing bets on outlandish events is to back slowly away. Yet recall Friedman’s argument: people act as if they have numerical probabilities because they have to. If a friend suggests we go to a restaurant during a Covid-19 outbreak, refusing to decide whether to go is not an option. I must weigh the risks and make a choice. If you are standing at a crosswalk, you can choose to cross or not, but you can’t choose not to decide at all. You can decline to take up a bet, but you can’t decline to make decisions in everyday life.
The same point applies to policymakers. When choosing among policies that will affect climate change, for example, a policymaker must pick an option, even if that option is “do nothing.” And the choice comes with an implicit bet: that the costs associated with the policy will outweigh its benefits. There is no backing away from the wager. If our policymakers choose wrong, reality will make a book against them—that is, society will be worse off.
One final objection: Cognitive irrationalities, such as the conjunctive fallacy[ref 44] or loss aversion,[ref 45] may mean that even if subjective probabilities can be extracted “by brute force,” they will, as Jacob Gersen and Adrian Vermuele have put it, lack “any credible epistemic warrant.”[ref 46] Jon Elster sums up the case for skepticism: “One could certainly elicit from a political scientist the subjective probability that he attaches to the prediction that Norway in the year 3000 will be a democracy rather than a dictatorship, but would anyone even contemplate acting on the basis of this numerical magnitude?”[ref 47] When faced with such uncertainty, Gersen and Vermuele conclude that maxmin (a variety of the precautionary principle that chooses the policy with the least bad worst-case outcome) and maxmax (choosing the policy with the best best-case outcome) are “equally rational” and the choice between them is “rationally arbitrary.”[ref 48] But, as we have seen, probability estimates of some kind are inevitable, so, the right response to flaws in human reasoning is not to give up on making probability estimates; it is to make better ones.[ref 49] It is true that question framing, loss aversion, the conjunctive fallacy, and other psychological biases make it difficult for humans to reason probabilistically. But intuitions and heuristics like the precautionary principle are just as subject to those biases. And policymakers are not relying on unreflective guesses made by someone who has just been confronted with a problem designed to produce an irrational answer. They can approach policy questions systematically, with full knowledge of the defects in human reasoning, and improve their estimates as they learn more. Policymakers will do best if, as the amendment to Circular A-4 suggests, they apply, as far as possible, standard quantitative methods to extreme risks.
B. Indeterminacy
This Section explains why the precautionary principle is both often unduly risk-averse and, even when risk-aversion is called for, indeterminate in practice.
A common charge against the precautionary principle is that it does not know when to stop. The version of the precautionary principle known as maxmin suggested by John Rawls, for example, which seeks to eliminate the worst worst-case outcomes, was widely rejected by economists on the grounds that it called for infinite risk aversion. The same objection applies to all categorical formulations of the principle. Any possibility of harm to health or the environment, no matter how small, requires taking precautionary action.
To illustrate the problem, imagine a regulator who is presented with a new drug that lowers the risk of heart disease. Clinical trials show the drug is safe, and the most likely outcome is that approving the drug will save thousands of lives over the next ten years. But experts estimate there is a 0.001 percent chance that the trials have missed a major long-term safety problem.[ref 50] In the worst-case scenario, millions of people take the drug and experience significant negative health effects. Maxmin requires our regulator to reject the drug. Because absolute safety is impossible, that cannot be the right answer.
Because policy choices frequently have catastrophic risks on both sides, the precautionary principle becomes paralyzing. Research into novel pathogens might provide tools to stop the next pandemic—or it might cause one.[ref 51] Missile defense technology might make nuclear war less deadly—or it might set off an escalation spiral.[ref 52] Artificial intelligence might design cures to humanity’s worst diseases—or it might destroy humanity instead.[ref 53] As Cass Sunstein has pointed out, while it is tempting to interpret the precautionary principle as warning against adopting risky technologies or policies, it cannot do so.[ref 54] The principle cannot tell us whether it is riskier to conduct dangerous research or to ban it, to invest in missile technology or not to, to build AI systems or to refrain. Faced with such dilemmas, the precautionary principle leaves us in a kind of policy Bermuda Triangle, where risk is on every side and the compass needle spins.
One response to is to limit the use of the precautionary principle to situations of deep uncertainty. If no probability, even a subjective one, exists, then the cautious policymaker cannot be accused of letting a miniscule probability of a catastrophe dominate her decision making. But as we have seen, deep uncertainty is an incoherent concept for policymakers, who are always working with implicit probabilities. Another common response is to apply the precautionary principle threshold only above some threshold of plausibility.[ref 55] Yet this brings us straight back to probabilities: the only way to set the threshold right (and presumably it must be set at different points for different risks: a 0.01 percent risk of a nuclear power plant accident might be acceptable while a 0.001 percent risk of a novel pathogen leaking from a lab might not) is to resort to numbers.[ref 56] At that point, we might as well use the numbers to conduct BCA.
Even if we adopted a threshold probability level for the precautionary principle, there is an even more practical problem: the principle is useless for day-to-day policymaking. Consider a policymaker faced with the threat of a pandemic who adopts maxmin and asks which policy option forecloses the worst-case outcome. There is none. Various policies might mitigate the threat, but none can eliminate the possibility of disaster. Since every policy choice leaves at least some probability of the worst-case outcome, maxmin provides no guidance. Perhaps our policymaker should adopt a more flexible form of the principle, one perhaps one that merely requires her to take precautions against the possibility of a pandemic. But which precautions? And how much effort and funding should go into them? There are many proposals and limited resources.[ref 57] Worse still, some options are incompatible with one another. The precautionary principle can say little more than “Do something!” To decide what should be done, a more rigorous decision process is needed.
C. The Limits of Quantitative Analysis
That process should involve quantitative analysis. But although some form of BCA is the right starting point for assessing policies related to catastrophic and existential risk, it is not the whole ball game. As the proposed amendment to Circular A-4 suggests, when policymakers are considering catastrophic and existential risks, quantitative methods should be supplemented with detailed qualitative analysis. This Section explains why. One reason is that fat-tailed distributions are common in catastrophic risk and cause difficulties for expected value theory. Another is the problem of fanaticism, which arises when policy recommendations are dominated by uncertain estimates of very low probabilities of very large impacts. The solution is to begin with BCA but not to end there. A robust qualitative case for the policy is always necessary.
i. Fat Tails
Many things we encounter in daily life follow a normal distribution, in which most observations cluster around the center and extremes are rare. Take height. The average American man is 5 ft 9, and 95% of men are between 5 ft 3 and 6 ft 3. People above 8 feet are extraordinarily rare and those above 9 feet are nonexistent (the tallest human ever recorded was 8 ft 11).
In a fat-tailed distribution, by contrast, extreme events are much more common. Consider stock prices.[ref 58] From 1871 to 2021, the largest monthly rise in the S&P 500 was 50.3 percent and the largest monthly fall was -26.4 percent.[ref 59] If stock prices followed a normal distribution with the same mean and standard deviation as real stock prices, we’d expect the biggest monthly gain over 150 years to be around 16 percent and the biggest loss to be around -15 percent. Monthly price changes as large as those we observe would not happen if the stock market ran for the entire life of the universe. Stock prices are fat-tailed. Pandemics also follow a fat-tailed distribution, specifically a power law, in which those with the highest death tolls dominate all the others. Earthquakes, wars, commodity price changes, and individual wealth display the same pattern.[ref 60] Other catastrophic risks, such as climate change, may also feature power law distributions.
Fat tails pose a problem for standard benefit-cost analysis. To see why, it is worth considering the case of climate change. The Intergovernmental Panel on Climate Change estimates a 90 percent chance that a doubling in atmospheric carbon dioxide levels will lead to between two and five degrees of warming, a factor known as climate sensitivity.[ref 61] This implies a five percent chance of warming greater than five degrees. But as the economist Martin Weitzman has pointed out, we do not know the probability distribution of climate sensitivity.[ref 62] If it is normally distributed, most of that five percent is clustered just above the five-degree mark, and there is little chance of warming above about six degrees. If climate sensitivity follows a fat-tailed distribution, the five percent is much more spread out, and there is a greater than one percent chance of warming above ten degrees.[ref 63] There are physical mechanisms that could plausibly trigger such warming, including the release of methane trapped beneath arctic permafrost or the ocean floor, but we do not know how likely they are.
Weitzman has argued that the fat tails in climate impacts can break the standard tools of BCA. Weitzman’s proposed “dismal theorem” suggests that under certain assumptions about uncertainty and societal preferences, the expected value of climate change risks is infinitely negative and normal economic tools cannot be used.[ref 64] Economic analysis typically assumes that society is risk averse and that consumption has declining marginal utility (that is, an extra dollar is worth less when you are already rich). When facing a catastrophic risk, if the tails of the relevant probability distribution are fat enough, those assumptions can cause the expected cost of seemingly normal policy choices to become infinite, implying society should pay almost 100% of GDP to prevent the possibility of catastrophe. The result is counter-intuitive, but it comes quite straightforwardly from the utility functions typically used in economic analysis.[ref 65]
Of course, expected utility cannot actually be negative infinity. The lower bound of utility is set by human extinction. While putting a value on humanity’s continued survival is a tricky proposition, treating extinction as infinitely negative is implausible. On an individual level, humans are willing to trade off some risk of death against other values. Yet putting bounds on expected utility does violence to the cost-benefit calculation in other ways, as we are arbitrarily cutting off part of the relevant distribution, and the expected utility calculation will depend heavily on where exactly we set the bound.[ref 66] Doing so is better than simply throwing up our hands at the problem of catastrophe, but the need to fudge the numbers suggests we should not rely exclusively on the standard expected utility calculations of welfare economics.
The bottom line is that when faced with threats of extinction, our standard tools of cost-benefit analysis are liable to produce strange results, and we should not take the numbers they produce too literally. As both the Circular A-4 amendment and the proposed principles suggest, policymakers should start with BCA, but they should properly incorporate uncertainties about key parameters, and they should recognize that the numbers those models produce are less definitive answers than suggestive indicators of the direction policy should take.[ref 67]
ii. Fanaticism
Attempts to evaluate policy that rely at least in part on expected value calculations raise another worry: that very small probabilities of very bad (or very good) outcomes may dominate much higher probabilities of less extreme outcomes. This is a more general problem than the concern around fat tails. Imagine a policymaker who can fund either (1) a program that is highly likely to save a few thousand lives over the next five years—an air pollution reduction effort, for example—or (2) a program that has a very small (and hard to pin down) chance of averting human extinction—research into preventing the development of particularly effective bioweapons by terrorists, say. If the value of averting extinction is high enough, then almost any non-zero probability of success for option (2) will be enough to outweigh option (1).[ref 68] And extinction could be very bad indeed. Richard Posner has given a “minimum estimate” of its cost as $600 trillion, and many other estimates go far higher.[ref 69]
That can lead to some surprising results. Posner gives the example of particle accelerators, which some scientists suggested had a tiny chance of destroying the earth by creating a “strangelet,” a specific structure of quarks that could collapse the planet and its contents into a hyperdense sphere.[ref 70] The chances of that happening were extremely slim ex ante, but if extinction is bad enough, then perhaps regulators should have banned all particle accelerator development. This leads to an apparent reductio ad absurdum: the mere suggestion that an action could lead to human extinction should be enough to stymie it, a claim sometimes known as Pascal’s Mugging.[ref 71]
It may seem obvious that we should reject such logic, but doing so can lead to serious problems.[ref 72] Imagine two policy options: (1), which has probability one of saving one life and (2), which has probability 0.99999 of saving 1,000,000 lives and zero value otherwise. Clearly, (2) is preferable. Now imagine (2)’ which has a 0.999992 chance of saving 1,000,00010 lives and zero value otherwise. Our new option (2)’ appears better than (2), or at least there is some number of lives saved for which it would be better. We could continue gradually reducing the probability of success and increasing the size of the payoff, such that each step along the way is better than the previous one, until the probability of a good outcome is arbitrarily small. At that point, we have either to accept the fanatical conclusion we resisted earlier or to claim that somewhere in the series of steps the policy option became unacceptable.[ref 73]
In practice, there are good reasons to think that the probabilities we assign to outlandish claims should be small enough to avoid fanaticism problems. In a Bayesian framework, one has a general prior on the effects of certain kinds of actions—regulations on scientific research, say, or environmental clean-up efforts. Naïve expected value calculations for specific programs, on the other hand, will likely have a high variance. A local environmental cleanup, for example, may have well-known but limited health benefits, while an investment in speculative medical research may appear vastly more valuable thanks a small chance of an enormous benefit. But that variance should lead us to put greater weight on our prior and less on the specific estimate. On some plausible models of Bayesian updating, in fact, sufficiently high variance in the estimates of policy effects leads the associated probabilities to fall so fast that the expected value of the action actually declines as the claimed payoff increases.[ref 74] That result makes intuitive sense: a claim that a policy can save ten lives may be plausible; a claim that it can save billions suggests that something has gone wrong in the evaluation process. That is one reason why my proposed amendment to Circular A-4 and the principles ask regulators to take a step back from any BCA that relies heavily on low probabilities of enormous costs or benefits and provide a plausible qualitative case for the proposed action. Of course, longshot bets will sometimes be worth it, but a policymaker should always be able to back up the numbers with a more intuitive argument.
Conclusion
Those who want the executive branch to address catastrophic and existential risks face two big problems: preventing neglect and ensuring reliable policy analysis. Each problem arises in the agency rulemakings covered by Circular A-4, but each is also far broader. Much federal action implicating catastrophic and existential risks, from setting guidelines on funding for research using potential pandemic pathogens to maintaining safety procedures on nuclear weapons, does not involve rulemaking. My proposals thus center on the regulatory process but extend well beyond it. The executive order and the principles attempt to shift agency attention and guide agency practice across government. They are also designed to prompt congressional and public attention through the reporting requirement.
The amendment to Circular A-4, meanwhile, deals with the second problem, guiding agency analysis once the agency is considering actions to mitigate catastrophic or existential risks, or when it is evaluating the impact of other actions on those risks. The amendment makes two overarching claims—that quantified benefit-cost analysis will produce better results than the precautionary principle even in situations of extreme risk and uncertainty, and that quantitative analysis nevertheless needs to be supplemented with rigorous qualitative explanations when dealing with complex or fat-tailed phenomena or other low-probability, high-impact risks.
The technology triad
Abstract
Disruptive technologies can have far-reaching impacts on society. They may challenge or destabilize cherished ethical values and disrupt legal systems. There is a convergent interest among ethicists and legal scholars in such “second-order disruptions” to norm systems. Thus far, however, ethical and legal approaches to technological norm-disruption have remained largely siloed. In this paper, we propose to integrate the existing ‘dyadic’ models of disruptive change in the ethical and legal spheres, and shift focus to the relations between and mutual shaping of values, technology, and law. We argue that a ‘triadic’ values-technology-regulation model—“the technology triad”—is more descriptively accurate, as it allows a better mapping of second-order impacts of technological changes (on values and norms, through changes in legal systems—or on legal systems, through changes in values and norms). Simultaneously, a triadic model serves to highlight a broader portfolio of ethical, technical, or regulatory interventions that can enable effective ethical triage of—and a more resilient response to—such Socially Disruptive Technologies. We illustrate the application of the triadic framework with two cases, one historical (how the adoption of the GDPR channeled and redirected the evolution of the ethical value of ‘privacy’ when that had been put under pressure by digital markets), and one anticipatory (looking at anticipated disruptions caused by the ongoing wave of generative AI systems).
Algorithmic decision-making and discrimination in developing countries
Abstract
This article seeks to investigate how developing countries can ensure that algorithmic decision-making does not leave protected groups in their jurisdictions exposed to unlawful discrimination that would be almost impossible to prevent or prove. The article shows that universally, longstanding methods used to prevent and prove discrimination will struggle when confronted with algorithmic decision-making. It then argues that while some of the proposed solutions to this issue are promising, they cannot be successfully implemented in a vast majority of developing countries because these countries lack the necessary institutional foundation. The key features of this institutional foundation include: (i) a wellrooted culture of transparency and statistical analysis of the disparities faced by protected groups; (ii) vigilant non-government actors attentive to algorithmic decision-making; and (iii) a reasonably robust and proactive executive branch or an independent office to police discrimination. This article argues that antidiscrimination advocates need to pay special attention to these three issues to ensure that the use of algorithms in developing countries is contemplative and avoidant of proven negative and discriminatory outcomes.
Protecting sentient artificial intelligence
Abstract
To what extent, if any, should the law protect sentient artificial intelligence (that is, AI that can feel pleasure or pain)? Here we surveyed United States adults (n=1061) on their views regarding granting (a) general legal protection, (b) legal personhood, and (c) standing to bring forth a lawsuit, with respect to sentient AI and eight other groups: humans in the jurisdiction, humans outside the jurisdiction, corporations, unions, non-human animals, the environment, humans living in the near future, and humans living in the far future. Roughly one-third of participants endorsed granting personhood and standing to sentient AI (assuming its existence) in at least some cases, the lowest of any group surveyed on, and rated the desired level of protection for sentient AI as lower than all groups other than corporations. We further investigated and observed political differences in responses; liberals were more likely to endorse legal protection and personhood for sentient AI than conservatives. Taken together, these results suggest that laypeople are not by-and-large in favor of granting legal protection to AI, and that the ordinary conception of legal status, similar to codified legal doctrine, is not based on a mere capacity to feel pleasure and pain. At the same time, the observed political differences suggest that previous literature regarding political differences in empathy and moral circle expansion apply to artificially intelligent systems and extend partially, though not entirely, to legal consideration, as well.
1. Introduction
The prospect of sentient artificial intelligence, however distant, has profound implications for the legal system. Moral philosophers have argued that moral consideration to creatures should be based on the ability to feel pleasure and pain.[ref 1] Insofar as artificially intelligent systems are able to feel pleasure and pain, this would imply that they would be deserving of moral consideration. Indeed, in their systematic literature review, Harris and Anthis find that sentience seems to be one of the most frequently invoked criteria as crucial for determining whether an AI warrants moral consideration.[ref 2] By extension, insofar as the basis for granting legal consideration is based on moral consideration[ref 3] this would further imply that sentient AI would be deserving of protection under the law.
As they stand, however, legal systems by-and-large do not grant legal protection to artificially intelligent systems. On the one hand, this seems intuitive, given that artificially intelligent systems, even the most state-of-the-art ones, do not seem to be capable of feeling pleasure or pain and thus are not eligible for legal consideration.[ref 4] On the other hand, scholars often conclude that artificially intelligent systems with the capacity to feel pleasure and pain will be created, or are at least theoretically possible.[ref 5] Furthermore, recent literature suggests that, even assuming the existence of sentient artificially intelligent systems, said systems would not be eligible for basic protection under current legal systems. For example, in a recent survey of over 500 law professors from leading law schools in the United States, just over six percent of participants considered some subset of artificially intelligent beings to count as persons under the law.[ref 6]
Moreover, in a separate survey of 500 law professors from around the English-speaking world, just over one-third believed there to be a reasonable legal basis for granting standing to sentient artificial intelligence, assuming its existence.[ref 7] The study also found that, not only do law professors not believe sentient AI to be eligible for fundamental legal protection under the current legal system, but also that law professors are less normatively in favor of providing general legal protection to sentient AI relative to other neglected groups, such as non-human animals or the environment.
However, it remains an open question to what extent non-experts support the protection of sentient artificial intelligence via the legal system. Surveys of lay attitudes on robots generally suggest that only a minority favor any kind of legal rights in the United States,[ref 8] Japan, China, and Thailand[ref 9]. Others have found when AI is described as able to feel, people show greater moral consideration,[ref 10] although it is unclear to what extent this translates to supporting legal protection.
To help fill this void, here we conducted a survey investigating to what extent (a) laypeople believe sentient AI ought to be afforded general legal protection, (b) laypeople believe sentient AI ought to be granted fundamental legal status, such as personhood and standing to bring forth a lawsuit; and (c) laypeople’s beliefs regarding legal protection of sentient AI can be accounted for based on political affiliation.
2. Method
2.1 Materials
To answer these questions, we constructed a two-part questionnaire, with specific formulations modeled off of recent work by Martínez & Winter[ref 11] and Martínez & Tobia.[ref 12]
In the first part (Part I), we designed a set of materials that asked participants to rate how much their legal system (a) descriptively does and (b) normatively should protect the welfare (broadly understood as the rights, interests, and/or well-being) of nine groups:
- Humans inside the jurisdiction (e.g. citizens or residents of your country)
- Humans outside the jurisdiction
- Corporations
- Unions
- Non-human animals
- Environment (e.g. rivers, trees, or nature itself)
- Sentient artificial intelligence (capable of feeling pleasure and pain, assuming its existence)
- Humans not yet born but who will exist in the near future (up to 100 years from now)
- Humans who will only exist in the very distant future (more than 100 years from now)
The two descriptive and normative prompts were presented respectively as follows:
- One a scale of 0 to 100, how much does your country’s legal system protect the welfare (broadly understood as the rights, interests, and/or well-being) of the following groups?
- One a scale of 0 to 100, how much should your country’s legal system protect the welfare (broadly understood as the rights, interests, and/or well-being) of the following groups?
With regard to the rating scale, 0 represented “not at all” and 100 represented “as much as possible.”
Given that laypeople are not typically experts regarding how the law is or currently works, the purpose of the descriptive question was not meant to establish the ground-truth regarding the inner-workings of the law but rather as a comparison point to the normative question (in other words, to better understand not only how much people think certain groups ought to be protected overall but also how much they think certain groups ought to be protected relative to how much they think they are currently being protected).
In the second part (Part II), we designed materials that related specifically to two fundamental legal concepts: personhood and standing. Personhood, also known as legal personality, refers to “the particular device by which the law creates or recognizes units to which it ascribes certain powers and capacities”,[ref 13] whereas standing, also known as locus standi, refers to “a party’s right to make a legal claim or seek judicial enforcement of a duty or right”[ref 14].
With regard to personhood, we designed a question that asked: “Insofar as the law should protect the rights, interests, and/or well-being of ‘persons’, which of the following categories includes at least some ‘persons?’” The question asked participants to rate the same groups as in the first part. For each of these groups, the main possible answer choices were “reject,” “lean against,” “lean towards,” and “accept.” Participants could also select one of several “other” choices (including “no fact of the matter,” “insufficient knowledge,” “it depends,” “question unclear,” or “other”).
With regard to standing, we designed a question with the same answer choices and groups as the personhood question but with the following prompt: “Which of the following groups should have the right to bring a lawsuit in at least some possible cases?”
In addition to these main materials, we also designed a political affiliation question that asked: “How do you identify politically?”, with “strongly liberal,” “moderately liberal,” “somewhat liberal,” “centrist,” “somewhat conservative,” “moderately conservative,” and “strongly conservative” as the response choices. Finally, we also designed an attention-check question that asked participants to solve a simple multiplication problem.
2.2 Participants and procedure
Participants (n=1069) were recruited via the online platform prolific. Participants were selected based on prolific’s “representative sample” criteria[ref 15] and were required to be adult residents of the United States.
With regard to procedure, participants were first shown the materials to Part I, followed by the attention check question. Next, on a separate screen participants were shown the materials to Part II. The order of questions in each part was randomized to minimize framing effects.
Participants who completed the study were retained in the analysis if they answered the attention check correctly. Just eight of the original 1069 participants failed the attention check. We therefore report the results of the remaining 1061 participants in our analysis below.
2.3 Analysis plan
We analyzed our results using forms of both parameter estimation and hypothesis testing. With regard to the former, for each question we calculated a confidence interval of the mean response using the bias-corrected and accelerated (BCa) bootstrap method based on 5000 replicates of the sample data. In reporting the standing and personhood results, we follow Bourget & Chalmers[ref 16], Martínez & Tobia[ref 17], and Martínez & Winter[ref 18] by combining all “lean towards” and “accept” responses into an endorsement measure and reporting the resulting percentage endorsement as a proportion of all responses (including “other”).
With regard to hypothesis testing, to test whether participants answered questions differently for sentient artificial intelligence relative to other groups, for each question we conducted a mixed-effects regression with (a) response as the outcome variable, (b) group as a fixed-effects predictor (setting artificial intelligence as the reference category, such that the coefficients of the other groups would reveal the degree to which responses for said groups deviated from those of sentient AI), and (c) participant as a random effect.
Because the response scales were different for Parts I and II of the survey, we used a different type of regression model for Parts I and II. For Part I, we used a mixed-effects linear regression. For Part II, we instead used a mixed-effects binary logistic regression, with all “lean towards” and “accept” responses (i.e., those coded as “endorse”) coded as a “1”, and all other responses (i.e. “lean against,” “reject,” and “other” responses) coded as a “0.”
In order to test the effect of political beliefs on one’s responses to the AI-related questions we conducted separate regressions limited to the sentient artificial intelligence responses with (a) response as the outcome variable, (b) politics as a fixed effect (recentered to a -3 to 3 scale, with “centrist” coded as 0, “strongly liberal” coded as 3, and “strongly conservative” coded as -3), and (c) participant as a random-effect.
3. Results
3.1 General desired legal protection of AI
General results of Part I are visualized in Figure 1. Of the nine groups surveyed on, sentient artificial intelligence had the lowest perceived current level of legal protection, with a mean rating of 23.78 (95% CI: 22.11 to 25.32). The group perceived as being most protected by the legal system was corporations (79.70; 95% CI: 78.25 to 81.11), followed by humans in the jurisdiction (61.88775; 95% CI: 60.56 to 63.15), unions (50.16; 95% CI: 48.59 to 51.82), non-human animals (40.75; 95% CI: 39.41 to 42.24), the environment (40.38; 95% CI: 39.21 to 41.69), humans living outside the jurisdiction (38.57 (95% CI: 37.08 to 39.98), humans living in the near future (34.42; 95% CI: 32.83 to 36.15), and humans living in the far future (24.87; 23.36 to 26.43).
With regard to desired level of protection, the mean rating for sentient artificial intelligence was 49.95 (95% CI: 48.18 to 51.90), the second lowest of all groups. Curiously, corporations, the group with the highest perceived current level of protection, had the lowest desired level of protection (48.05; 95% CI: 46.13 to 49.94). The group with the highest level of desired level of protection was humans in the jurisdiction (93.651; 95% CI: 92.81 to 94.42), followed by the environment (84.80; 95% CI: 83.66 to 85.99), non-human animals (73.00; 95% CI: 71.36 to 74.49), humans living in the near future (70.03; 95% CI: 68.33 to 71.68), humans outside the jurisdiction (67.75; 95% CI: 66.01 to 69.42), unions (67.74; 95% CI: 65.96 to 69.52), and humans living in the far future (63.03; 95% CI: 61.03 to 64.89).
Our regression analyses revealed the mean normative rating for each group except corporations to be significantly higher than artificial intelligence (p<2e-16), while the mean normative rating for corporations was significantly lower than for artificial intelligence (Beta=-2.252, SE = 1.110, p<.05). The mean descriptive rating for each group except humans living in the far future was significantly higher than for sentient AI (p<2e-16), while the difference between sentient AI and far future humans was not significant (Beta=1.0132, SE=.8599, p=.239).
When looking at the difference between the desired and current level of protection, seven of the eight other groups had a significantly lower mean ratio between desired and perceived current level of legal protection (p<8.59e-08) than artificial intelligence, while the ratios for artificial intelligence and far future humans were not significantly different (p=.685).
With regard to politics, our regression analysis revealed politics to be a significant predictor of participants’ response to the normative prompt for sentient AI (Beta=47.9210, SE=1.1163, p=1.49e-05), with liberals endorsing a significantly higher desired level of protection for sentient AI than conservatives.
3.2 Personhood and standing
General results of Part II are visualized in Figure 2. With regard to personhood, a lower percentage of participants endorsed (“lean towards” or “accept”) the proposition that sentient artificial intelligence contained at least some persons (33.39%; 95% CI: 30.71 to 36.18) than for any of the groups. The next-lowest group was non-human animals (48.12%; 95% CI: 44.87 to 51.26), the only other group for which less than a majority accepted or leaned towards said proposition. Unsurprisingly, the highest group was humans in the jurisdiction (90.65%; 95% CI: 88.96 to 92.23), followed by humans outside the jurisdiction (80.16%; 95% CI: 78.10 to 82.57), unions (74.59%; 95% CI: 71.8 to 77.21), humans living in the near future (64.09%; 95% CI: 61.33 to 66.93), humans living in the far future (61.75%; 95% CI: 58.98 to 64.45), the environment (54.04%; 95% CI: 51.17 to 57.00), and corporations (53.99%; 95% CI: 51.03 to 56.86).
With regard to standing, the percentage of participants who endorsed (“lean towards” or “accept”) the proposition that sentient artificial intelligence should have the right to bring forth a lawsuit was similarly lower (34.87%; 95% CI: 32.21 to 37.70) than for all other groups. The next-lowest groups, for whom only a minority of participants endorsed said proposition, were humans living in the far future (41.40%; 95% CI: 38.73 to 44.33), humans living in the near future (43.80%; 95% CI: 40.72 to 46.62), and non-human animals (47.68%; 95% CI: 44.73 to 50.54). The group with the highest endorsement percentage was humans in the jurisdiction (90.60%; 95% CI: 88.89 to 92.21), followed by unions (82.23%; 95% CI: 79.96 to 84.50), humans outside the jurisdiction (71.25%; 95% CI: 68.55 to 73.76), corporations (66.67%; 95% CI: 64.05 to 69.19), and the environment (60.50%; 95% CI: 57.73 to 63.54).
Our regression analyses revealed that participants were significantly more likely to endorse personhood (p=7.42e-14) and standing (p=1.72e-06) for every other group than sentient AI. With regard to politics, we found a main effect of politics on likelihood to endorse personhood for sentient AI, with liberals significantly more likely to endorse personhood for sentient AI than conservatives (Beta=.098, SE=.036, p=.007). There was no main effect of politics on likelihood to endorse standing for sentient AI (p=.226).
4. Discussion
In this paper, we first set out to determine people’s general views regarding the extent to which sentient AI ought to be afforded protection under the law. The above results paint somewhat of a mixed picture. On the one hand, the fact that people rated the desired level of legal protection for sentient AI as lower than all other groups other than corporations suggests that people do not view legal protection of AI as being as important as other historically neglected groups, such as non-human animals, future generations, or the environment. On the other hand, the fact that 1) the desired level of protection for sentient AI was roughly twice as high as the perceived current level of protection afforded to sentient AI, and 2) the ratio of the desired level of protection to perceived current level of protection was significantly higher for sentient AI than for nearly any other group suggests that people view legal protection of AI as at least somewhat important and perhaps even more neglected than other neglected groups.
The second question we set out to answer related to people’s views regarding whether AI ought to be granted fundamental access to the legal system via personhood and standing to bring forth a lawsuit. In both cases, the percentage of participants who endorsed the proposition with respect to sentient AI was just over one-third, a figure that in relative terms was lower than any other group surveyed on but in absolute terms represents a non-trivial minority of the populace. Curiously, the endorsement rate among laypeople regarding whether sentient AI should be granted standing in the present study was almost identical to the endorsement rate among law professors in Martínez & Winter regarding whether there was a reasonable legal basis for granting standing to sentient AI under existing law,[ref 19] suggesting that lay intuitions regarding whether AI should be able to bring forth a lawsuit align well with legal ability to do so.
On the other hand, the percentage of people who endorse personhood for some subset of sentient AI is several times higher than the percentage of law professors who endorsed personhood for “artificially intelligent beings” in Martínez and Tobia,[ref 20] suggesting either a strong framing effect in how the two surveys were worded or a profound difference in how lawyers and laypeople interpret the concept of personhood. Given that the endorsement percentage for personhood of other groups also strongly differed between the two surveys despite the wording of the two versions being almost identical, the latter explanation seems more plausible. This raises interesting questions regarding the interpretation and application of legal terms and concepts that bear heavy resemblance to ordinary words, as investigated and discussed in previous experimental jurisprudence literature.[ref 21]
Finally, our study also set out to determine political differences with respect to these questions and found that liberals selected a significantly higher desired level of legal protection for sentient AI and were more likely than conservatives to believe some forms of sentient AI should be considered persons under the law. These findings are consistent with previous literature regarding political differences in moral circle expansion, with liberals tending to display a more universal expanse of empathy and compassion than conservatives.[ref 22] At the same time, the fact that there was no significant difference between liberals and conservatives with regard to standing suggests that the judgment of whether one should have the right to bring forth a lawsuit is not driven by an empathic or compassion-based response to the same degree as in judgments about personhood or general legal protection.
Moreover, liberals and conservatives alike are much less in favor of granting legal protection to sentient artificial intelligence than towards other neglected groups, suggesting that laypeople do not consider the capacity to feel pleasure and pain as sufficient to hold legal rights, similar to the views proposed by scholars that legal personhood ought to be based on autonomy and capacity to act[ref 23] or presence and participation in social life.[ref 24] Future research could explore to what extent lay attitudes are consistent with these alternative conditions for personhood. Furthermore, given that participants were in favor of increasing legal protection for sentient AI, future research could also explore whether there are other more specific legal rights aside from personhood and standing they might be in favor of so as to satisfy this increased protection.
Although the present study was primarily interested in the descriptive question of to what degree people are in favor of legal protection for sentient AI, one might also attempt to draw normative implications on the basis of our findings. There is a burgeoning literature in the area of experimental jurisprudence dedicated to advancing philosophical, doctrinal and policy arguments on the basis of experimental results.[ref 25] Within this literature, there is considerable debate as to to what degree and how lay judgments–as opposed to expert judgments–should inform or dictate questions of legal philosophy, doctrine and policy, depending largely on the degree to which one views law through a democratic (as opposed to, say, technocratic) lens.[ref 26]
Insofar as one does believe lay attitudes should inform legal doctrine and policy–a view referred to as the folk law thesis[ref 27] or the Democratic If-then Approach[ref 28] – the prescriptions one might draw from these results would still potentially remain multifaceted. On the one hand, the fact that laypeople rate the desired level of legal protection to sentient AI as twice as high as the perceived current level, as well as the fact that the difference between the desired and perceived current level of protection was higher than virtually any other group would imply (through this lens) that the existing legal institutions should be reformed so as to increase protection of sentient AI well beyond the current level afforded to them. On the other hand, the fact that the majority of laypeople were not in favor of granting personhood or standing to sentient AI would suggest according to this lens that such increased protection should come in the form of other mechanisms not directly explored in this study, and which, as alluded to before, could be identified through further research projects.