Catastrophic uncertainty and regulatory impact analysis
Summary
Cost-benefit analysis embodies techniques for the analysis of possible harmful outcomes when the probability of those outcomes can be quantified with reasonable confidence. But when those probabilities cannot be quantified (“deep uncertainty”), the analytic path is more difficult. The problem is especially acute when potentially catastrophic outcomes are involved, because ignoring or marginalizing them could seriously skewing the analysis. Yet the likelihood of catastrophe is often difficult or impossible to quantify because such events may be unprecedented (runaway AI or tipping points for climate change) or extremely rare (global pandemics caused by novel viruses in the modern world). OMB’s current guidance to agencies on unquantifiable risks is now almost twenty years old and in serious need of updating. It correctly points to scenario analysis as an important tool but it fails to give guidance on the development of scenarios. It then calls for a qualitative analysis of the implications of the scenarios, but fails to alert agencies to the potential for using more rigorous analytic techniques.
Decision science does not yet provide consensus solutions to the analysis of uncertain catastrophic outcomes. But it has advanced beyond the vague guidance provided by OMB since 2003, which may not have been state-of-the-art even then. This paper surveys these developments and explains how they might best be incorporated into agency practice. Those developments include a deeper understanding of potential options and issues in constructing scenarios. They also include analytic techniques for dealing with unquantifiable risks that can supplement or replace the qualitative analysis currently suggested by OMB guidance. To provide a standard framework for discussion of uncertainty in regulatory impact analyses, the paper also proposes the use of a structure first developed for environmental impact statements as a way of framing the agency’s discussion of crucial uncertain outcomes.
Incorporating Catastrophic Uncertainty in Regulatory Impact Analysis
There are well-developed techniques for incorporating possible harmful outcomes into regulatory impact analysis when the probability of those outcomes can be quantified with reasonable confidence. But when those probabilities cannot be quantified, the analytic path is more difficult. The problem is especially acute when potentially catastrophic outcomes are involved, because ignoring or marginally them risks seriously skewing the analysis. Yet the likelihood of a catastrophic is often difficult to quantify because such events may be unprecedented (runaway AI or climate change) or extremely rare (global pandemics in the modern world).
OMB’s current guidance to agencies on this topic is now almost twenty years old and in serious need of updating. It correctly points to scenario analysis as an important tool, but it fails to give guidance on the development of scenarios. It then calls for the a qualitative analysis of the implications of the scenarios, but fails to alert agencies to the potential for using more rigorous analytic techniques.
Decision science does not yet provide consensus solutions to the analysis of uncertain catastrophic outcomes. It has, however, advanced beyond the vague guidance currently provided by OMB, or choosing the alternative with the least-bad possible outcome (maxmin). This paper surveys some of the key developments and describes how they might best be incorporated into agency practice. It is important in doing so to take into account the analytic demands that are already placed on often-overburdened agencies and the variability between agencies in economic expertise and sophistication.
Even at the time OMB’s guidance was issued, there was model language that OMB could have used that would have at least provided a general framework for agencies to use. That language is found in the Council on Environmental Quality’s guidelines for the treatment of uncertainty in environmental impact statements. Using that language as a template has several advantages. Many agencies are familiar with the language, as are courts. Judicial interpretation provides additional guidance on the use of that language. Since agency decisions requiring cost-benefit analysis often also require an environmental assessment or impact statement, creating some parallelism between the NEPA requirements and OMB guidance will also allow the relevant documents to mesh more easily.
The paper proposes modifying this template to make specific reference to some leading techniques provided by decision science. There is a plausible argument that decision makers should simply apply their own intuitions to weighing unquantifiable risks of potential catastrophe. This paper takes another tack for two reasons. First, it is difficult to form judgments about scenarios that are likely to be far outside of the decision maker’s past experience. Whatever analytic help can be offered in making those judgment calls would be beneficial. Second, to have a chance of adoption, proposals should be geared to the technical, economics-oriented perspective espoused by OIRA. Even for those who do not find formalized decision methods adequate, it is better to move the regulatory process in the direction of fuller consideration of potential catastrophic risks rather than having such possibilities swept to the margins of analysis because of a desire for “rigor.”
I. Circular A-4, Uncertainty, and Later Generations
Our starting point is the current OMB guidance on regulatory impact analysis. The section of Circular A-4 begins by discussing uncertainty as a general topic regardless of quantifiability. It then has a short discussion that is specifically focused on unquantifiable uncertainty:
In some cases, the level of scientific uncertainty may be so large that you can only present discrete alternative scenarios without assessing the relative likelihood of each scenario quantitatively. For instance, in assessing the potential outcomes of an environmental effect, there may be a limited number of scientific studies with strongly divergent results. In such cases, you might present results from a range of plausible scenarios, together with any available information that might help in qualitatively determining which scenario is most likely to occur. When uncertainty has significant effects on the final conclusion about net benefits, your agency should consider additional research prior to rulemaking.1
The passage then goes on to discuss the possible benefits of obtaining additional information, “especially for cases with irreversible or large upfront investments.”2 This passage is notably lacking in guidance about how to develop scenarios or what to do with the results.
Circular A-4 also contains a discussion of future generations. It advises:
Special ethical considerations arise when comparing benefits and costs across generations. Although most people demonstrate time preference in their own consumption behavior, it may not be appropriate for society to demonstrate a similar preference when deciding between the well-being of current and future generations. Future citizens who are affected by such choices cannot take part in making them, and today’s society must act with some consideration of their interest. One way to do this would be to follow the same discounting techniques described above and supplement the analysis with an explicit discussion of the intergenerational concerns (how future generations will be affected by the regulatory decision).3
The remainder of this discussion of Circular A-4 focuses on the choice of discount rates, primarily arguing for discounting on the ground that future generations will be wealthier than the current generation. The literature on discounting over multiple generations has advanced considerably since then, with a consensus in favor of declining discount rates as a hedge against unexpectedly low economic-welfare in future generations.4
The use of discounting assumes, however, that we can quantify risks and their costs in future time periods. In the nature of things, this becomes increasingly difficult over time, as models are extended further beyond their testable results and as society diverges further from historical experience.
In the nearly 20 years since Circular A-4, the state of the art regarding analysis of unquantified uncertainty has made real progress. OMB’s guidance should be improved accordingly.
II. Case Study: Climate Change
The potentially catastrophic multigenerational risks that have been most heavily researched involve global climate change. Climate change is the poster child for the twin problems of uncertainty and impacts on future generations. Impacts on future generations are inherent in the nature of the earth system’s response to radiation forcing by greenhouse gases. Uncertainty is due to the immense complexity of the climate system and the consequent obstacles to modeling, combined with the long time spans involved and the unpredictability of human responses. Thus, climate change presses current methods of risk assessment and management to their limits and beyond.
The IPCC uses a standardized vocabulary to characterize quantifiable risks.5
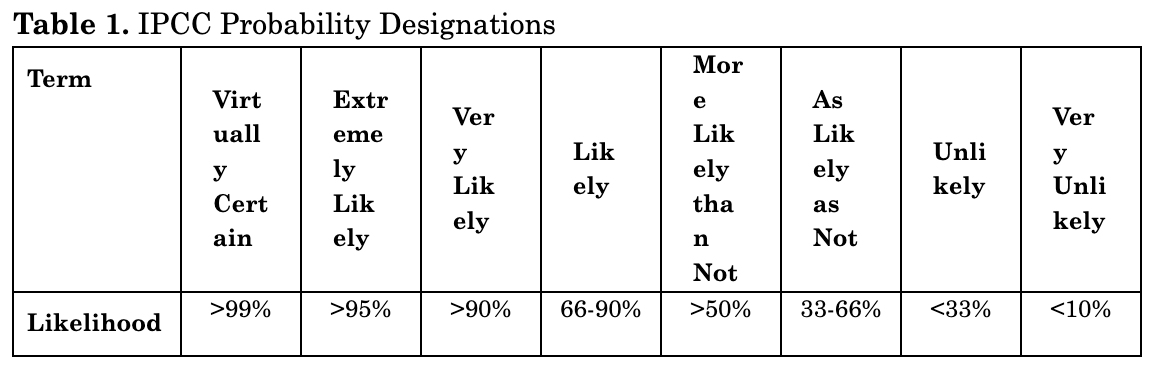
Neither lawyers nor economists have evolved anything similar. The efforts of scientists to provide systemized designations of uncertainty is an indication of the attention they have given to both quantifiable and unquantifiable risks.
As the IPCC explains:
In summary, while high-warming storylines—those associated with global warming levels above the upper bound of the assessed very likely range—are by definition extremely unlikely, they cannot be ruled out. For SSP1-2.6, such a high-warming storyline implies warming well above rather than well below 2°C (high confidence). Irrespective of scenario, high-warming storylines imply changes in many aspects of the climate system that exceed the patterns associated with the best estimate of GSAT [global mean near-surface air temperature] changes by up to more than 50% (high confidence).6
High climate sensitivities also increase the potential for warming above the 3 °C level. This, in turn, is linked to possible climate responses whose likelihood is very poorly understood. The IPCC reports a number of potential tipping point possibilities with largely irreversible consequences. Of these, two tipping points are associated with “deep uncertainty” for warming levels above 3°C: collapse of Western Antarctic ice sheets and shelves, and global sea level rise.7 Uncertainty is also reported as high regarding the potential for abrupt changes in Antarctic sea ice and in the Southern Ocean Meridional Overturning Circulation.8
The IPCC also indicates that damage estimates at given levels of warming are also subject to considerable uncertainty:
Projected estimates of global aggregate net economic damages generally increase non-linearly with global warming levels (high confidence). The wide range of global estimates, and the lack of comparability between methodologies, does not allow for identification of a robust range of estimates (high confidence). The existence of higher estimates than assessed in AR5 indicates that global aggregate economic impacts could be higher than previous estimates (low confidence). Significant regional variation in aggregate economic damages from climate change is projected (high confidence) with estimated economic damages per capita for developing countries often higher as a fraction of income (high confidence). Economic damages, including both those represented and those not represented in economic markets, are projected to be lower at 1.5°C than at 3°C or higher global warming levels (high confidence).9
Economists might view this assessment of the state of the art in modeling climate damages as too harsh. Nevertheless, it seems clear that we cannot assume that present estimates accurately represent the true probability distribution of possible damages.
The possibility of catastrophic tipping points looms large in economic modeling of climate change. Another key issue in terms of the economic analysis is the possibility of unexpectedly bad outcomes, such major melting of ice sheets, releases of large amounts of methane, and halting of the Gulf Stream. 10 William Nordhaus, who pioneered the economic models of climate change, has explained how these affect the analysis:
[W]e might think of the large-scale risks as a kind of planetary roulette. Every year that we inject more CO2 into the atmosphere, we spin the planetary roulette wheel. . . .
A sensible strategy would suggest an insurance premium to avoid the roulette wheel in the Climate Casino. . . . We need to incorporate a risk premium not only to cover the known uncertainties such as those involving climate sensitivity and health risks but also … uncertainties such as tipping points, including ones that are not yet discovered.11
The difficulty, as Nordhaus admits, is trying to figure out the extent of the premium. Another recent book by two leading climate economists argues that the downside risks are so great that “the appropriate price on carbon is one that will make us comfortable enough to know that we will never get to anything close to 6 °C (11 °F) and certain eventual catastrophe.”12 Although they admit that “never” is a bit of an overstatement—reducing risks to zero is impractical—they clearly think it should be kept as low as feasibly possible. Not all economists would agree with that view, but there seems to be a growing consensus that the possibility of catastrophic outcomes should play a major role in determining the price on carbon.13
Scientists are beginning to gain greater knowledge about long-term climate impacts after the end of this century. The IPCC reports advances in modeling up through 2300. Under all but the lowest emissions scenarios, global temperatures continue to rise markedly through the 22nd century.14 Moreover, “[s]ea level rise may exceed 2 m on millennial time scales even when warming is limited to 1.5°C–2°C, and tens of metres for higher warming levels.”15 Indeed, “physical and biogeochemical impacts of 21st century emissions have a potential committed legacy of at least 10,000 years.”16
To provide some perspective of the scale of the potential changes:
To place the temperature projections for the end of the 23rd century into the context of paleo temperatures, GSAT [global surface air temperature] under SSP2-4.5 (likely 2.3°C–4.6°C higher than over the period 1850–1900) has not been experienced since the Mid Pliocene, about three million years ago. GSAT projected for the end of the 23rd century under SSP5-8.5 (likely 6.6°C–14.1°C higher than over the period 1850–1900) overlaps with the range estimated for the Miocene Climatic Optimum (5°C–10°C higher) and Early Eocene Climatic Optimum (10°C–18°C higher), about 15 and 50 million years ago, respectively (medium confidence)17
The current emission pathway seems unlikely to produce the second, more severe scenario (though “unlikely” does not mean impossible). But the first scenario (SSP2-4.5) represents a middle-of-the road future in which global emissions peak around 2050 and decline through 2100.18 Even that scenario could put the world well out of the range of what homo sapiens has ever experienced, making predictions about future damages inherently uncertain.
III. Decisions Methods under Deep Uncertainty
It is one thing to perceive the significance of potential catastrophic risks; it is another to incorporate them into the analysis in a useful way. The precautionary principle is one effort to do so in a qualitative way, though the principle is controversial, particularly among economists. Use of precaution in the case of catastrophic risks has had some support even from Cass Sunstein, a leading critic of the precautionary principle.19 Sunstein has proposed a number of different versions of the catastrophic risk precautionary principle, in increasing order of stringency.20 The first required only that regulators take into account even highly unlikely catastrophes.21 Another version “asks for a degree of risk aversion, on the theory that people do, and sometimes should, purchase insurance against the worst kinds of harm.”22 Hence, Sunstein said, “a margin of safety is part of the Catastrophic Harm Precautionary Principle—with the degree of the margin depending on the costs of purchasing it.”23 Finally, Sunstein suggested, “it sometimes makes sense to adopt a still more aggressive form of the Catastrophic Harm Precautionary Principle, one “selecting the worst-case scenario and attempting to eliminate it.”24 More recently, Sunstein has endorsed use of maxmin in situations involving catastrophic risks, with some suggestions for possible guardrails to ensure that this test is applied sensibly.25 As Sunstein’s effort illustrates, it may be possible to clarify the areas of application for the precautionary principle sufficiently to make the principle a workable guide to decisions.
Maximin is a blunt tool, however, particularly in cases where precaution is very expensive in terms of resource utilization or foregone opportunities. There are variations on this principle and other possible tools for dealing with nonquantifiable risks.26 “Ambiguity” is a term that is often used to refer to situations in which the true probability distribution of outcomes is not known.27 There is strong empirical evidence that people are averse to ambiguity. The classic experiment involves a choice between two urns. One is known to contain half red balls and half blue; the other contains both colors but in unknown proportions. Regardless of which color they are asked to bet on, most individuals prefer to place their bet on the urn with the known composition.28 This is inconsistent with standard theories of rational decision making: if the experimental subjects prefer the known urn when asked to bet on red, that implies that they think that there are fewer than fifty percent red balls in the other urn. Consequently, they should prefer the second urn when asked to bet on blue—if it is less than half red it must be more than half blue—but they do not. Apparently, people prefer not to bet on an urn of uncertain composition.29 Such aversion to ambiguity “appears in a wide variety of contexts.”30 Ambiguity aversion may reflect a sense of lacking competence to evaluate a gamble.31
Ambiguity aversion may lead to irrational decision in some settings like laboratory experiments in which subjects know with certainty what scenarios are possible and their contents, it may be a much more reasonable attitude in practice. If we have two models of a situation available with completely different implications, that suggests that we do not understand the dynamics of the situation. That in turn means that the situation could be different from both models in some unknown way, and that whatever process the two models are trying to represent is really unknown to us. In the stylized example, perhaps there are green balls in the urn as well, or maybe the promised payoffs from our gamble won’t appear. To take an example from foreign relations, it is one thing to deal with a leader who has a track record of being capricious and unpredictable (a situation involving risk); it is another to have no idea of who is leading the country on any given day (deep uncertainty). Our ability to plan for the future is limited in such situations, and it is reasonable to want to avoid being put in that position.
There are a number of different approaches to modeling uncertainty about the true probability distribution.32 One is the Klibanoff-Marinacci-Mukerji model.33 This approach assumes that decision makers are unsure about the correct probability. Their decision is based on (a) the likelihood that the decision maker attaches to different probability distributions, (b) the degree to which the decision maker is averse to taking chances about which probability distribution is right, and (c) the expected utility of a decision under each of the possible probability distributions. In simpler terms, the decision maker combines the expected outcome under each probability distribution according to the decision maker’s beliefs about the distributions and attitude toward uncertainty regarding the true probability distribution. The shape of the function used to create the overall assessment determines in a straightforward way whether the decision maker is uncertainty averse, uncertainty neutral, or uncertainty seeking.34
The Klibanoff-Marinacci-Mukerji model has an appealing degree of generality. But this model is not easily applied because the decision maker needs to be able to attach numerical weights to the specific probability distributions, which may not be possible in cases of true uncertainty where the possible distributions are themselves unknown.35 The model fits best with situations where our uncertainty is fairly tightly bounded: we know all of the possible models and how they behave, although we are unsure which one is correct.
Other models of ambiguity are more tractable and apply in situations where uncertainty may run deeper. As economist Sir Nicholas Stern explained, in these models of uncertainty, “the decision maker, who is trying to choose which action to take, does not know which of [several probability] distributions is more or less likely for any given action.”36 He explains that it can be shown the decision maker would “act as if she chooses the action that maximises a weighted average of the worst expected utility and the best expected utility …. The weight placed on the worst outcome would be influenced by concern of the individual about the magnitude of associated threats, or pessimism, and possibly any hunch about which probability might be more or less plausible.”37
These models are sometimes called α-maxmin models, with α representing the weighting factor between best and worst cases.38 One way to understand these models is that we might want to minimize our regret for making the wrong decision, where we regret not only disastrous outcomes that lead to the worst case scenario, but also we regret having missed the opportunity to achieve the best case scenario. Alternatively, a can be a measure of the balance between our hopes (for the best case) and our fears (of the worst case).
Applying these α-maxmin models as a guide to action leads to what we might call the α-precautionary principle. Unlike most formulations of the precautionary principle, α-precaution is not only aimed at avoiding the worst case scenario; it also involves precautions against losing the possible benefits of the best case scenario.391 In some situations, the best case scenario is more or less neutral, so that α-maxmin is not much different from pure loss avoidance, unless the decision maker is optimistic and uses an especially low alpha. But where the best case scenario is potentially extremely beneficial, unless the decision maker’s alpha is very high, α-precaution will suggest a more neutral attitude toward uncertainty in order to take advantage of potential upside gains.
For example, suppose we have two models about what will happen if a certain decision is made. We assume that each one provides us enough information to allow the use of conventional risk assessment techniques if we were to assume that the model is correct. For instance, one model might have an expected harm of $1 billion and a variance of $0.2 billion; the other an expected harm of $10 billion and a variance of $3 billion. If we know the degree of risk aversion of the decision maker, we can translate each outcome into an expected utility figure for each model. The trouble is that we do not know which model is right, or even the probability of correctness. Hence, the situation is characterized by uncertainty. To assess the consequences associated with the decision, we then use a weighted average of these two figures based on our degree of pessimism and ambiguity aversion. This averaging between models allows us to compare the proposed course of action with other options.
An interesting variant of α-maxmin uses a weighted average that includes not only the best case and worst case scenarios, but also the expected value of the better understood, intermediate part of the probability distribution.40 This approach “is a combination between the mathematical expectation of all the possible outcomes and the most extreme ones.”41 This tri-factor approach may be “suitable for useful implementations in situations that entangle both more reliable (‘risky’) consequences and less known (‘uncertain’), extreme outcomes.”42 However, this approach requires a better understanding of the mid-range outcomes and their probabilities than does α-maxmin.
We seem to be suffering from an embarrassment of riches, in the sense of having too many different method for making decisions in situations in which extreme outcomes weigh heavily. At present, it is not clear that any one method will emerge as the most useful for all situations. For that reason, the ambiguity models should be seen as providing decision makers with a collection of tools for clarifying their analysis rather than providing a clearly defined path to the “right” decision.
Among this group of tools, α-maxmin has a number of attractive features. First, it is complex enough to allow the decision maker to consider both the upside and downside possibilities, without requiring detailed probability information that is unlikely to be available. Second, it is transparent. Applying the tool requires only simple arithmetic. The user must decide on what parameter value to use for α, but this choice is intuitively graspable as a measure of optimism versus pessimism. Third, α-maxmin can be useful in coordinating government policy. It is transparent to higher level decision makers and thus suited to central oversight.
Rather than asking the decision maker to assess highly technical probability distributions and modeling, α-maxmin simply presents the decision maker with three questions to consider: (1) What is the best case outcome that is plausible enough to be worth considering? (2) What is the worst case scenario that is worth considering? (3) How optimistic or pessimistic should we be in balancing these possibilities?43 These questions are simple enough for politicians and members of the public to understand. More importantly, rather than concealing value judgments in technical analysis by experts, they present the key value judgments directly to the elected or appointed officials who should be making them. Finally, these questions also lend themselves to oversight by outside experts, legislators, and journalists, which is desirable in societal terms even if not always from the agency’s perspective.
A. Identifying Robust Solutions
Another approach, which also finds its roots in consideration of worst case scenarios, is to use scenario planning to identify unacceptable courses of action and then choose the most appealing remaining alternatives.44 Robustness rather than optimality is the goal. RAND researchers have developed a particularly promising method to use computer assistance in scenario planning.45 RAND’s Robust Decision Making (RDM) technique provides one systematic way of exploring large numbers of possible policies to identify robust solutions.46 As one of its primary proponents explains:
RDM rests on a simple concept. Rather than using computer models and data to describe a best-estimate future, RDM runs models on hundreds to thousands of different sets of assumptions to describe how plans perform in a range of plausible futures. Analysts then use visualization and statistical analysis of the resulting large database of model runs to help decisionmakers distinguish future conditions in which their plans will perform well from those in which they will perform poorly. This information can help decisionmakers identify, evaluate, and choose robust strategies — ones that perform well over a wide range of futures and that better manage surprise.47
During each stage of the analysis, RDM uses statistical analysis to identify policies that perform well over many possible situations.48 It then uses datamining techniques to identify the future conditions under which such policies fail. New policies are then designed to cope with those weaknesses, and the process is repeated for the revised set of policies. As the process continues, policies become robust under an increasing range of circumstances, and the remaining vulnerabilities are pinpointed for decision makers.49 More specifically, “RDM uses computer models to estimate the performance of policies for individually quantified futures, where futures are distinguished by unique sets of plausible input parameter values.”50 Then, “RDM evaluates policy models once for each combination of candidate policy and plausible future state of the world to create large ensembles of futures.”51 The analysis “may include a few hundred to hundreds of thousands of cases.”52
The process could be compared with stress-testing various strategies to see how they perform under a range of circumstances. There are differences, however. RDM may also consider how strategies perform under favorable circumstances as well as stressful ones; it is able to test a large number of strategies or combination of strategies; and strategies are often modified (for instance by incorporating adaptive learning) as a result of the analysis.
A related concept, which discards strategies known to be dangerous, is known as the safe minimum standards (SMS) approach.53 This approach may apply in situations in which there are discontinuities or threshold effects, but there is considerable controversy about its validity.54 A related variant is to impose a reliability constraint, requiring that the odds of specified bad outcomes be kept below a set level.55 The existence of threshold effects makes information about the location of thresholds quite valuable. For instance, in the case of climate change, a recent paper estimates that the value of early information about climate thresholds could be as high as three percent of gross world product.56
B. Scenario Construction
The RDM methodology and α-maxmin are technical overlays on the basic idea of scenario analysis. Robert Verchick has emphasized the importance of scenario analysis—and of the act of imagination required to construct and consider these scenarios—in the face of nonquantifiable uncertainty.57 As he explained, scenario analysis avoids the pitfall of projecting a single probable future when vastly different outcomes are possible; broadens knowledge by requiring more holistic projections; forces planners to consider changes within society as well as outside circumstances; and, equally importantly, “forces decision-makers to use their imaginations.”58 He added that the “very process of constructing scenarios stimulates creativity among planners, helping them to break out of established assumptions and patterns of thinking.”59 In situations in which it is impossible to give confident odds on the outcomes, scenario planning may be the most fruitful approach.60
There is now a well-developed scholarly literature about the construction and uses of scenarios.61 By 2010, it could be said that “combining rich qualitative storylines with quantitative modeling techniques, known as the storyline and simulation approach, has become the accepted method for integrated environmental assessment and has been used in all major assessments including those by the IPCC, Millennium Ecosystem Assessment, and many regional and national studies.”62 Storylines “describe plausible, but alternative socioeconomic development pathways that allow scenario analysts to compare across a range of different situations, generally from 20 to 100 years into the future.”63 Because our limited ability to predict the future evolution of society on a multi-decadal basis (let along multi-century), scenarios are a particularly useful technique in dealing with problems involving long time spans and future generations. Best practices for constructing scenarios have also emerged. They include “pooling independently elicited judgments, extrapolating trend lines, and looking through data through the lenses of alternative assumptions.”64
Within the scenario family, however, are several different subtypes, varying in the process for developing the scenario, whether the scenario is exploratory or designed to exemplify the pathway to a given outcome (such as achieving the Paris Agreements goals), or informal as opposed to probabilistic.65
Use of scenarios in the context of climate change has been a particular subject of attention.66 The IPCC has developed one set of scenarios (the SSP scenarios67) for future pathways of societal development. Roughly speaking, these scenarios differ in the amount of international cooperation and whether society is stressing economic growth or environmental sustainability.68 The SSP scenarios include detailed assumptions about population, health, education, economic growth, inequality and other factors.69 A different set of scenarios, the RCPs,70 are used to model climate impacts based on different future trajectories of GHG concentrations. Thus, “the RCPs generate climate projections that are not interpreted as corresponding to specific societal pathways, while the SSPs are alternative futures in which no climate impacts occur nor climate policies implemented.”71 Integrated models are then used to combine the socioeconomic storylines, emissions trajectories, and climate impacts into a single simulation.72 One significant finding is that only some scenarios are compatible with achieving the Paris Agreemen’st aim of keeping warming to 1.5°C.73 One advantage of the IPCC approach is that it provides standardized scenarios that can be used by many researchers looking at many different aspects of climate change.
There seems to be no one “right” way to construct scenarios. As with the assessment methods discussed above, the most important thing in practice may be for the agency to explain the options and the reasons for choosing one approach over the others. It might also be useful for agencies to standardize their scenarios where possible, which would create economies of scale in scenario development, allow comparison of results across different regulations, and provide focal points for researchers.
Conclusion
Current guidance to agencies treats situations of deep uncertainty — those situations where we cannot reliably quantify risks — as an afterthought. This is particularly unfortunate in terms of catastrophic risks. Such risks are generally rare, meaning a sparse history of prior events (which may be completely lacking when novel threats are involved.) They often involve complex dynamics that make prediction difficult. The more catastrophic the event, the more likely it is to have impacts across multiple generations, changing the future in ways that are hard to predict. Yet planning only for better understood, more predictable risks may blind regulators to crucial issues. Catastrophic risks, even when they seem remote before the fact, may matter far more than the more routine aspects of a situation, in the spirit of “Except for that, how did you like the play, Mrs. Lincoln?” Fortunately, devastating pandemics, economic collapses, environmental tipping points, and disastrous runaway technologies are not the ordinary stuff of regulation. But where such catastrophes are relevant, marginalizing their consideration is dangerous.
There is no clear-cut answer to how we should integrate consideration of potential catastrophic outcomes into regulatory analysis. However, two decades after the last OMB guidance, we have a clearer understanding of the available analytic tools and better frameworks for applying them. If we cannot expect agencies to get all the answers right, we can at least expect them to carefully map out the analytic techniques they have used and why they have chosen them. This paper has explained the range of tools available and called for a revamping of OMB guidance to give clearer direction to agencies on their use. Even imperfect tools are better than relegating the risk of catastrophe to the shadows in regulatory decision making.