Concepts in advanced AI governance: a literature review of key terms and definitions
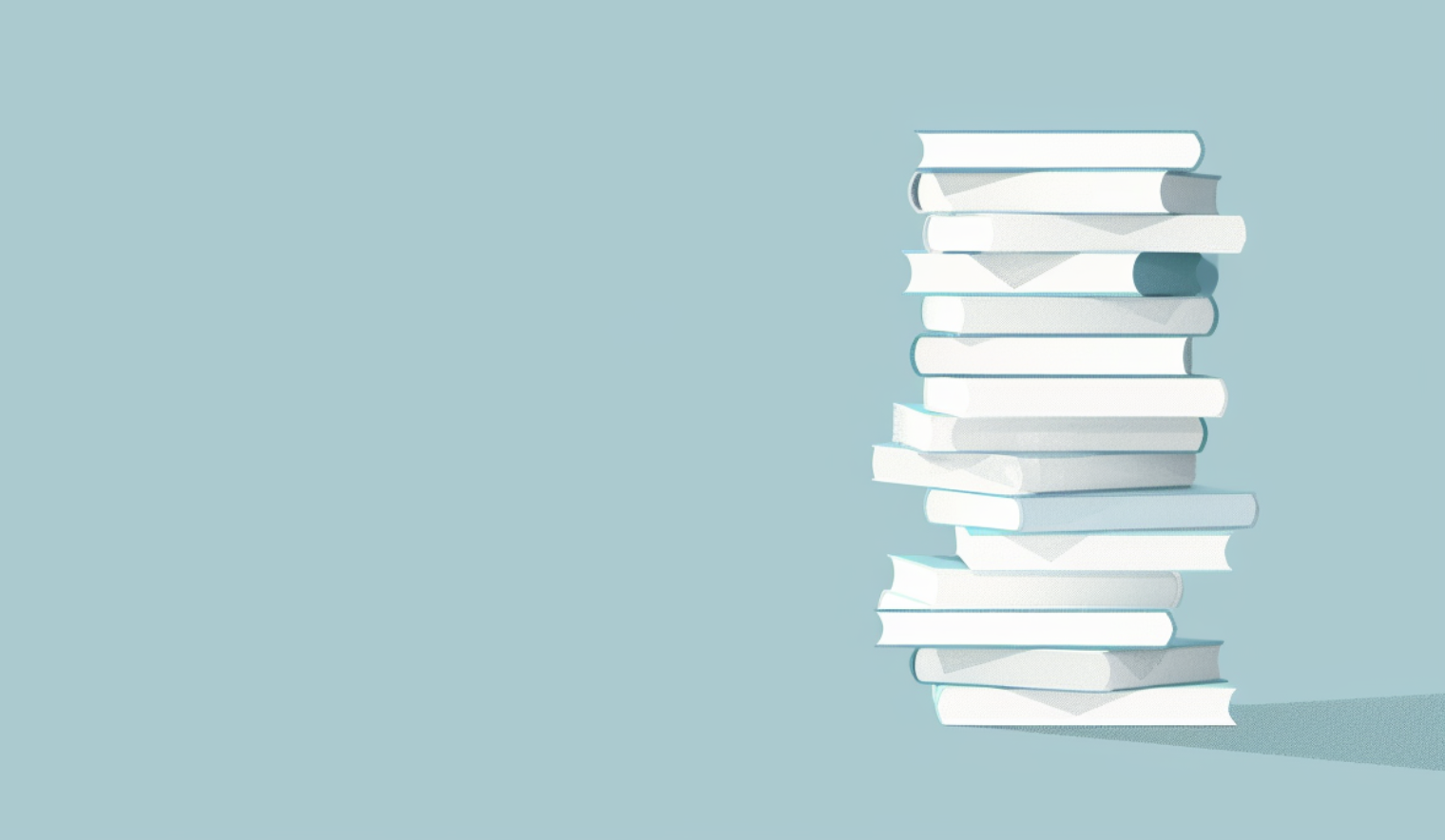
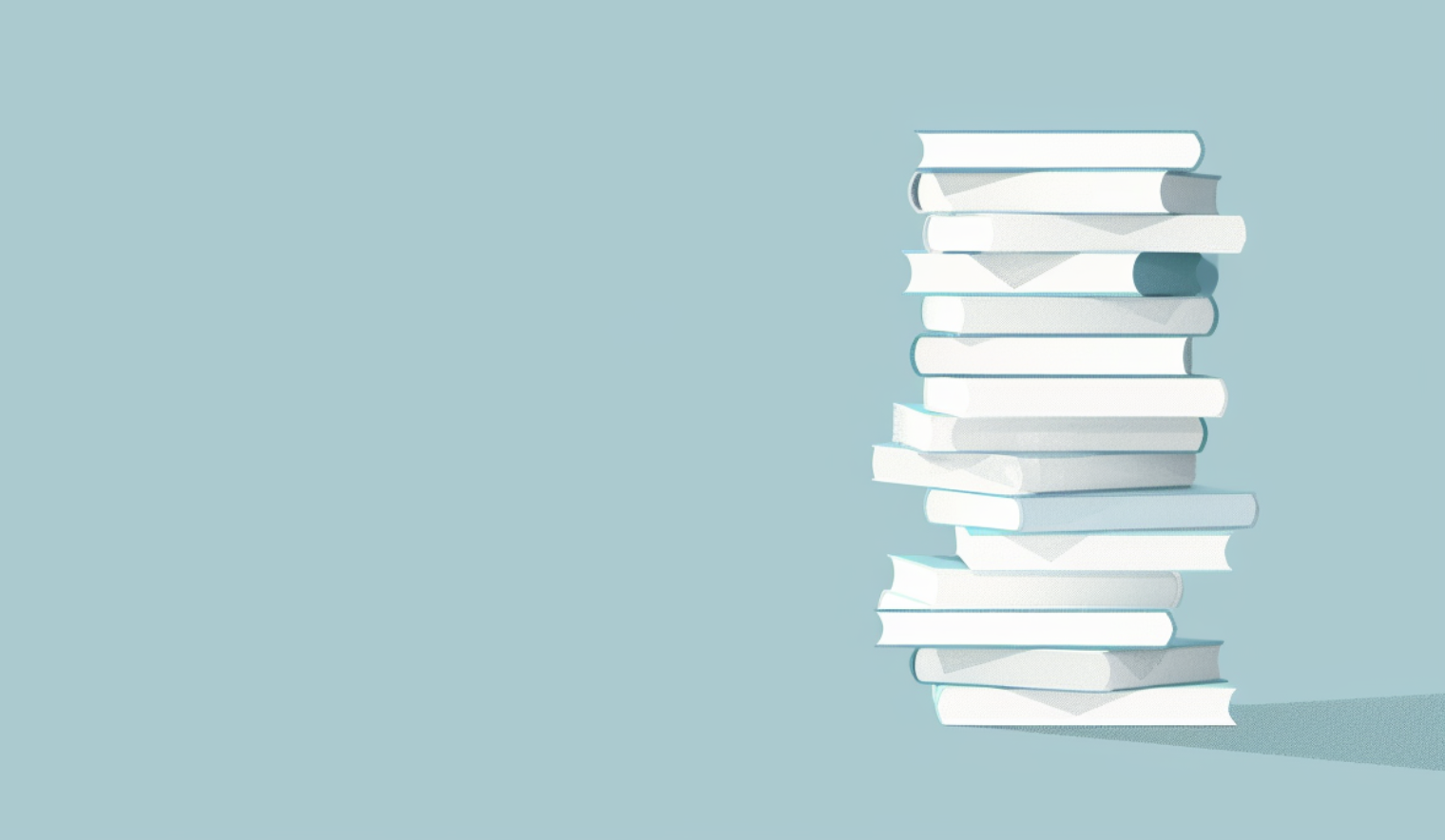
Abstract
As AI systems have become increasingly capable, policymakers, the public, and the field of AI governance have begun to consider the potential impacts and risks from these systems—and the question of how best to govern such increasingly advanced AI. Call this field “Advanced AI Governance.” However, debates within and between these communities often lack clarity over key concepts and terms. In response, this report provides an overview, taxonomy, and preliminary analysis of many cornerstone ideas and concepts within advanced AI governance.
To do so, it first reviews three different purposes for seeking definitions (technological, sociotechnical, and regulatory), and discusses why and how terminology matters to both the study and the practice of AI governance. Next, the report surveys key definitions in advanced AI governance. It reviews 101 definitions across 69 terms that have been coined for advanced AI systems, within four categories: (1) essence-based concepts that focus on the anticipated form of advanced AI, (2) development-based terms that emphasize the hypothesized pathways towards advanced AI, (3) sociotechnical-change-based terms that center the societal impacts of such AI, and (4) risk-based terms that highlight specific critical capabilities of advanced AI systems. The report then reviews distinct definitions of the tools of (AI) “policy” and “governance”, different paradigms within the field of advanced AI governance, and different concepts around theories of change. By disentangling these terms and definitions, this report aims to facilitate more productive conversations between AI researchers, academics, policymakers, and the public on the key challenges of advanced AI.
Executive summary
This report provides an overview, taxonomy, and preliminary analysis of many cornerstone ideas and concepts in the emerging field of advanced AI governance.
Aim: The aim of this report is to contribute to improved analysis, debate, and policy by providing greater clarity around core terms and concepts. Any field of study or regulation can be improved by such clarity.
As such, this report reviews definitions for four categories of terms: the object of analysis (e.g., advanced AI), the tools for intervention (e.g., “governance” and “policy”), the reflexive definitions of the field of “advanced AI governance”, and its theories of change.
Summary: In sum, this report:
- Discusses three different purposes for seeking definitions for AI technology, discusses the importance of such terminology in shaping AI policy and law, and discusses potential criteria for evaluating and comparing such terms.
- Reviews concepts for advanced AI, covering a total of 101 definitions across 69 terms, including terms focused on:
- the forms of advanced AI,
- the (hypothesized) pathways towards those advanced AI systems,
- the technology’s large-scale societal impacts, and
- particular critical capabilities that advanced AI systems are expected to achieve or enable.
- Reviews concepts within “AI governance”, such as nine analytical terms used to define the tools for intervention (e.g., AI strategy, policy, and governance), four terms used to characterize different approaches within the field of study, and five terms used to describe theories of change.
The terms are summarized below in Table 1. Appendices provide detailed lists of definitions and sources for all the terms covered as well as a list of definitions for nine other auxiliary terms within the field.
Introduction
As AI systems have become increasingly capable and have had increasingly public impacts, the field that focuses on governing advanced AI systems has come into its own.
While researchers come to this issue with many different motivations, concerns, or hopes about AI—and indeed with many different perspectives on or expectations about the technology’s future trajectory and impacts—there has grown an emerging field of researchers, policy practitioners, and activists concerned with and united by what they see as the increasingly significant and pivotal societal stakes of AI. Along with significant disagreements, many in this emerging community share the belief that shaping the transformative societal impacts of advanced AI systems is a top global priority.1 However, this field still lacks clarity regarding not only many key empirical and strategic questions but also many key terms that are used.
Background: This lack of clarity matters because the recent wave of progress in AI, driven especially but not exclusively by the dramatic success of large language models (LLMs), has led to an accumulation of a wide range of new terms to describe these AI systems. Yet many of these terms—such as “foundation model”,2 “generative AI”,3 or “frontier AI”4—do not always have clear distinctions5 and are often used interchangeably.6 They moreover emerge on top of and alongside a wide range of past terms, concepts, and words that have been used in the past decades to refer to (potential) advanced AI systems, such as “strong AI”, “artificial general intelligence”, or “transformative AI”. What are we to make of all of these terms?
Rationale: Critically, debates over terminology in and for advanced AI are not just semantics—these terms matter. In a broad sense, framings, metaphors, analogies, and explicit definitions can strongly affect not just developmental pathways for technology but also policy agendas and the efficacy and enforceability of legal frameworks.7 Indeed, different terms have already become core to major AI governance initiatives—with “general-purpose AI” serving as one cornerstone category in the EU AI Act8 and “frontier AI models” anchoring the 2023 UK AI Safety Summit.9 The varying definitions and implications of such terms may lead to increasing contestation,10 as well they should: Extensive work over the past decade has shown how different terms for “AI” import different regulatory analogies11 and have implications for crafting legislation.12 We might expect the same to hold for the new generation of terms used to describe advanced AI and to center and focus its governance.13
Aim: The aim of this report is to contribute to improved analysis, debate, and policy by providing greater clarity around core terms and concepts. Any field of study or regulation can be improved by such clarity. Such literature reviews may not just contribute to a consolidation of academic work, but can also refine public and policy debates.14 Ideally, they provide foundations for a more deliberate and reflexive choice over what concepts and terms to use (and which to discard), as well as a more productive refinement of the definition and/or operationalization of cornerstone terms.
Scope: In response, this report considers four types of terms, including potential concepts and definitions for each of the following:
- the core objects of analysis—and the targets for policy (i.e., what is the “advanced AI” to be governed?),
- the tools for intervention to be used in response (i.e., what is the range of terms such as “policy”, “governance”, or “law”?),
- the field or community (i.e., what are current and emerging accounts, projects, or approaches within the broader field of advanced AI governance?), and
- the theories of change of this field (i.e., what is this field’s praxis?).
Disclaimers: This project comes with some important caveats for readers.
First, this report aims to be relatively broad and inclusive of terms, framings, definitions, and analogies for (advanced) AI. In doing so, it draws from both older and recent work and from a range of sources from academic papers to white papers and technical reports to public fora.
Second, this report is primarily concerned with mapping the conceptual landscape and with understanding the (regulatory) implications of particular terms. As such, it is less focused on policing the appropriateness or coherence of particular terms or concepts. Consequently, with regard to advanced AI it covers many terms that are still highly debated or contested or for which the meaning is unsettled. Not all the terms covered are equally widely recognized, used, or even accepted as useful in the field of AI research or within the diverse fields of the AI ethics, policy, law, and governance space. Nonetheless, this report will include many of these terms on the grounds that a broad and inclusive approach to these concepts serves best to illuminate productive future debate. After all, even if some terms are (considered to be) “outdated,” it is important to know where such terms and concepts have come from and how they have developed over time. If some terms are contested or considered “too vague,” that should precisely speak in favor of aiming to clarify their usage and relation to other terms. This will either allow the (long overdue) refinement of concepts or will at least enable an improved understanding of when certain terms are not usefully recoverable. In both cases, it will facilitate greater clarity of communication.
Third, this review is a snapshot of the state of debate at one moment. It reviews a wide range of terms, many of which have been coined recently and only some of which may have staying power. This debate has developed significantly in the last few years and will likely continue to do so.
Fourth, this review will mostly focus on analytical definitions of or for advanced AI along four approaches.15 In so doing, it will on this occasion mostly omit detailed exploration of a fifth, normative dimension to defining AI, which would focus on reviewing especially desirable types of advanced AI systems that (in the view of some) ought to be pursued or created. Such a review would cover a range of terms such as “ethical AI”,16 “responsible AI”,17 “explainable AI”,18 “friendly AI”,19 “aligned AI”,20 “trustworthy AI”,21 “provably-safe AI”,22 “human-centered AI”,23 “green AI”,24 “cooperative AI”,25 “rights-respecting AI”,26 “predictable AI”,27 “collective intelligence”,28 and “digital plurality”,29 amongst many other terms and concepts. At present, this report will not focus in depth on surveying these terms, since only some of them were articulated in the context of or in consideration of especially advanced AI systems. However, many or all of these terms are capability-agnostic and so could clearly be extended to or reformulated for more capable, impactful, or dangerous systems. Indeed, undertaking such a deepening and extension of the taxonomy presented in this report in ways that engage more with the normative dimension of advanced AI would be very valuable future work.
Fifth, this report does not aim to definitively resolve debates—or to argue that all work should adopt one or another term over others. Different terms may work best in different contexts or for different purposes and for different actors. Indeed, given the range of actors interested in AI—whether from a technical engineering, sociotechnical, or regulatory perspective—it is not surprising that there are so many terms and such diversity in definitions even for single terms. Nonetheless, to be able to communicate effectively and learn from other fields, it helps to gain greater clarity and precision in the terms we use, whether these are terms referring to our objects of analysis, our own field and community, or our theory of action. Of course, achieving clarity on terminology is not itself sufficient. Few problems, technical or social or legal, may be solved exclusively by haggling over words. Nonetheless, a shared understanding facilitates problem solving. The point here is not to achieve full or definitive consensus but to understand disagreements and assumptions. As such, this report seeks to provide background on many terms, explore how they have been used, and consider the suitability of these terms for the field.30 In doing so, this report highlights the diversity of terms in current use and provides context for more informed future study and policymaking.
Structure: Accordingly, this report now proceeds as follows.
Part I provides a background to this review by discussing three purposes to defining key terms such as AI. It also discusses why the choice for one or another term matters significantly from the perspective of AI policy and regulation, and finally discusses some criteria by which to evaluate the suitability of various terms and definitions for the specific purpose of regulation.
In Part II, this report reviews a wide range of terms for “advanced AI”, across different approaches which variably focus on (a) the anticipated forms or design of advanced AI systems, (b) the hypothesized scientific pathways towards these systems, (c) the technology’s broad societal impacts, or (d) the specific critical capabilities particular advanced AI systems are expected to achieve.
Part III turns from the object of analysis to the field and epistemic community of advanced AI governance itself. It briefly reviews three categories of concepts of use for understanding this field. First, it surveys different terms used to describe AI “strategy”, “policy”, or “governance” as this community understands the available tools for intervention in shaping advanced AI development. It then reviews different paradigms within the field of advanced AI governance as ways in which different voices within it have defined that field. Finally, it briefly reviews recent definitions for theories of change that aim to compare and prioritize interventions into AI governance.
Finally, three appendices list in detail all the terms and definitions offered, with sources, and offer a list of auxiliary definitions that can aid future work in this emerging field.31etail, with sources; and offer a list of auxiliary definitions that can aid future work in this emerging field.
I. Defining ‘advanced AI (governance)’: Background
Any quest for clarifying definitions of “advanced AI” is complicated by the already long-running, undecided debates over how to even define the more basic terms “AI” or, indeed, “intelligence”.32
To properly evaluate and understand the relevance of different terms for AI, it is useful to first set out some background. In the first place, one should start by considering the purposes for which the definition is sought. Why or how do we seek definitions of “(advanced) AI”?
1. Three purposes for definitions
For instance, rather than trying to consider a universally best definition for AI, a more appropriate approach is to consider the implications of different definitions, or—to invert the question—to ask for what purpose we seek to define AI. We can consider (at least) three different rationales for defining a term like ‘AI’.
- To build it (the technological research purpose): In the first place, AI researchers or scientists may pursue definitions of (advanced) AI by defining it from the “inside,” as a science.33 The aim of such technical definitions of AI34 is to clarify or create research-community consensus about (1) the range and disciplinary boundaries of the field—that is, what research programs and what computational techniques35 count as “AI research” (both internally and externally to research funders or users); (2) the long-range goals of the field (i.e., the technical forms of advanced AI); and/or (3) the intermediate steps the field should take or pursue (i.e., the likely pathways towards such AI). Accordingly, this definitional purpose aligns particularly closely with essence-based definitions (see Part II.1) and/or development-based definitions (see Part II.2) of advanced AI.
- To study it (the sociotechnical research purpose): In the second place, experts (in AI, but especially in other fields) may seek to primarily understand AI’s impacts on the world. In doing so, they may aim to define AI from the “outside,” as a sociotechnical system including its developers and maintainers.36 Such definitions or terms can aid researchers (or governments) who seek to understand the societal impacts and effects of this technology in order to diagnose or analyze the potential dynamics of AI development, diffusion, and application, as well as the long-term sociopolitical problems and opportunities. For instance, under this purpose researchers may aim to get to terms with understanding issues such as (1) (the geopolitics or political economy of) key AI inputs (e.g., compute, data, and labor), (2) how different AI capabilities37 give rise to a spectrum of useful applications38 in diverse domains, and (3) how these applications in turn produce or support new behaviors and societal impacts.39 Accordingly, this purpose is generally better served by sociotechnical definitions of AI systems’ impacts (see Part II.3) or risk-based definitions (see Part II.4).
- To regulate it (the regulatory purpose): Finally, regulators or academics motivated by appropriately regulating AI—either to seize the benefits or to mitigate adverse impacts—can seek to pragmatically delineate and define (advanced) AI as a legislative and regulatory target. In this approach, definitions of AI are to serve as useful handles for law, regulation, or governance.40 In principle, this purpose can be well served by many of the definitional approaches: highly technology-specific regulations for instance can gain from focusing on development-based definitions of (advanced) AI. However, in practice regulation and governance is usually better served by focusing on the sociotechnical impacts or capabilities of AI systems.
Since it is focused on the field of “advanced AI governance,” this report will primarily focus on the second and third of these purposes. However, it is useful to keep all three in mind.
2. Why terminology matters to AI governance
Whether taking a sociotechnical perspective on the societal impacts of advanced AI or a regulatory perspective on adequately governing it, the need to pick suitable concepts and terms becomes acutely clear. Significantly, the implications and connotations of key terms matter greatly for law, policy, and governance. This is because, as reviewed in a companion report,41 distinct or competing terms for AI—with their meanings and connotations—can influence all stages of the cycle from a technology’s development to its regulation. They do so in both a broad and a narrow sense.
In the broad and preceding sense, the choice of term and definition can, explicitly or implicitly, import particular analogies or metaphors into policy debates that can strongly shape the direction—and efficacy—of the resulting policy efforts.42 These framing effects can occur even if one tries to avoid explicit analogies between AI and other technologies, since apparently “neutral” definitions of AI still focus on one or another of the technology’s “features” as the most relevant, framing policymaker perceptions and responses in ways that are not neutral, natural, or obvious. For instance, Murdick and others found that the particular definition one uses for what counts as “AI” research directly affects which (industrial or academic) metrics are used to evaluate different states’ or labs’ relative achievements or competitiveness in developing the technology—framing downstream evaluations of which nation is “ahead” in AI.43 Likewise, Kraftt and colleagues found that whereas definitions of AI that emphasize “technical functionality” are more widespread among AI researchers, definitions that emphasize “human-like performance” are more prevalent among policymakers, which they suggest might prime policymaking towards future threats.44
Beyond the broad policy-framing impacts of technology metaphors and analogies, there is also a narrower sense in which terms matter. Specifically, within regulation, legislative and statutory definitions delineate the scope of a law and of the agency authorization to implement or enforce it45—such that the choice for a particular term for (advanced) AI may make or break the resulting legal regime.
Generally, within legislative texts, the inclusion of particular statutory definitions can play both communicative roles (clarifying legislative intent), and performative roles (investing groups or individuals with rights or obligations).46 More practically, one can find different types of definitions that play distinct roles within regulation: (1) delimiting definitions establish the limits or boundaries on an otherwise ordinary meaning of a term, (2) extending definitions broaden a term’s meaning to expressly include elements or components that might not normally be included in its ordinary meaning, (3) narrowing definitions aim to set limits or expressly exclude particular understandings, and (4) mixed definitions use several of these approaches to clarify components.47
Likewise, in the context of AI law, legislative definitions for key terms such as “AI” obviously affect the material scope of the resulting regulations.48 Indeed, the effects of particular definitions have impacts on regulation not only ex ante, but also ex post: in many jurisdictions, legal terms are interpreted and applied by courts based on their widely shared “ordinary meaning.”49 This means, for instance, that regulations that refer to terms such as “advanced AI”, “frontier AI”, or “transformative AI” might not necessarily be interpreted or applied in ways that are in line with how the term is understood within expert communities. All of this underscores the importance of our choice of terms—from broad and indirect metaphors to concrete and specific legislative definitions—when grappling with the impacts of this technology on society.
Indeed, the strong legal effects of different terms mean that there can be challenges for a law when it depends on a poorly or suboptimally specified regulatory term for the forms, types, or risks from AI that the legislation means to address. This creates twin challenges. On the one hand, picking suitable concepts or categories can be difficult at an early stage of a technology’s development and deployment, when its impacts and limits are not always fully understood—the so-called Collingridge dilemma.50
At the same time, the cost of picking and locking in the wrong terms within legislative texts can be significant. Beyond the opportunity costs, unreflexively establishing legal definitions for key terms can create the risk of downstream or later “governance misspecification.”51
Such governance misspecification may occur when regulation is originally targeted at a particular artifact or (technological) practice through a particular material scope and definition for those objects. The implicit assumption here is that the term in question is a meaningful proxy for the underlying societal or legal goals to be regulated. While that assumption may be appropriate and correct in many cases, there is a risk that if that assumption is wrong—either because of an initial misapprehension of the technology or because subsequent technological developments lead to that proxy term diverging from the legislative goals—the resulting technology law will less efficient, ineffective, or even counterproductive to its purposes.52
Such cases of governance misspecification can be seen in various cases of technology governance and regulation. For instance:
- The “high-performance computer” threshold in US 1990s export control regimes: In the 1990s, the US established a series of export control regimes under the Wassenaar Arrangement, which set an initial threshold for “high-performance computers” at just 195 million theoretical operations per second (MTOPS); in doing so, the regime treated that technology as far too static and could not keep pace with Moore’s Law.53 As a result, the threshold had to be updated six times within a decade,54 even as the regime became increasingly ineffective at preventing or even inhibiting US adversaries from accessing as much computing power as they needed, and it may even have become harmful to national security as it inhibited the domestic US tech industry.55
- The “in orbit” provision in the Outer Space Treaty: In the late 1960s, the Outer Space Treaty aimed to outlaw positioning weapons of mass destruction in space. It therefore (as proxy) specified a ban on placing these weapons “in orbit.”56 This definition meant that there was a loophole to be exploited by the Soviet development of fractional orbital bombardment systems (FOBS), which were able to position nuclear weapons in space (on non-ballistic trajectories) without, strictly, putting them “in orbit.”57
- Under- and overinclusive 2010s regulations on drones and self-driving cars: Calo has chronicled how, in the early 2010s, various US regulatory responses to drones or self-driving cars defined these technologies in ways that were either under- or overinclusive, leading to inefficiency or the repeal of laws.58
Thus, getting greater clarity in our concepts and terminology for advanced AI will be critical in crafting effective, resilient regulatory responses—and in avoiding brittle missteps that are easily misspecified.
Given all the above, the aim in this report is not to find the “correct” definition or frame for advanced AI. Rather, it considers that different frames and definitions can be more useful for specific purposes or for particular actors and/or (regulatory) agencies. In that light, we can explore a series of broad starting questions, such as:
- What different definitions have been proposed for advanced AI? What other terms could we choose?
- What aspects of advanced AI (e.g., its form and design, the expected scientific principles of its development pathways, its societal impacts, or its critical capabilities) do these different terms focus on?
- What are the regulatory implications of different definitions?
In sum, this report is premised on the idea that exploring definitions of AI (and related terms) matters, whether we are trying to understand AI, understand its impacts, or govern them effectively.
3. Criteria for definitions
Finally, we have the question of how to formulate relevant criteria for suitable terms and definitions for advanced AI. In the first place, as discussed above, this depends on one’s definitional purpose.
Nonetheless, from the specific perspective of regulation and policymaking, what are some good criteria for evaluating suitable and operable definitions for advanced AI? Notably, Jonas Schuett has previously explored legal approaches to defining the basic term “AI”. He emphasizes that to be suitable for the purpose of governance, the choice of terms for AI should meet a series of requirements for all good legal definitions—namely that terms are neither (1) overinclusive nor (2) underinclusive and that they are (3) precise, (4) understandable, (5) practicable, and (6) flexible.59 Other criteria have been proposed: for instance, it has been suggested that an additional desiderata for a useful regulatory definition for advanced AI might include something like ex ante clarity—in the sense that the definition should allow one to assess, for a given AI model, whether it will meet the criteria for that definition (i.e., whether it will be regulated within some regime), and ideally allow this to be assessed in advance of deployment (or even development) of that model.60 Certainly, these criteria remain contested and are likely incomplete. In addition, there may be trade-offs between the criteria, such that even if they are individually acceptable, one must still strike a workable balance between them.61
II. Defining the object of analysis: Terms for advanced AI
Having briefly discussed the different definitional purposes, the relevance of terms for regulation, and potential criteria for evaluating definitions, this report now turns to survey the actual terminology for advanced AI.
Within the literature and public debate, there are many terms used to refer to the conceptual cluster of AI systems that are advanced—i.e., that are sophisticated and/or are highly capable and/or could have transformative impacts on society.62 However, because of this diversity of terms, not all have featured equally strongly in governance or policy discussions. To understand and situate these terms, it is useful to compare their definitions with others and to review different approaches to defining advanced AI.
In Schuett’s model for “legal” definitions for AI, he has distinguished four types of definitions, which focus variably on (1) the overarching term “AI”, (2) particular technical approaches in machine learning, (3) specific applications of AI, and (4) specific capabilities of AI systems (e.g., physical interaction, ability to make automated decisions, ability to make legally significant decisions).63
Drawing on Schuett’s framework, this report draws a similar taxonomy for common definitions for advanced AI. In doing so, it compares between different approaches that focus on one of four features or aspects of advanced AI.
- The anticipated technical form or design of AI systems (essence-based approaches);
- The proposed scientific pathways and paradigms towards creating advanced AI (development-based approaches);
- The broad societal impacts of AI systems, whatever their cognitive abilities (sociotechnical-change-based approach);
- The specific critical capabilities64 that could potentially enable extreme impacts in particular domains (risk-based approaches).
Each of these approaches has a different focus, object, and motivating question (Table 2).
This report will now review these categories of approaches in turn. For each, it will broadly (1) discuss that approach’s core definitional focus and background, (2) list the terms and concepts that are characteristic of it, (3) provide some brief discussion of common themes and patterns in definitions given to these terms,65 and (4) then provide some preliminary reflections on the suitability of particular terms within this approach, as well as of the approach as a whole, to provide usable analytical or regulatory definitions for the field of advanced AI governance.66
1. Essence-based definitions: Forms of advanced AI
Focus of approach: Classically, many definitions of advanced AI focus on the anticipated form, architecture, or design of future advanced AI systems.67 These definitions as such focus on AI systems that instantiate particular forms of advanced intelligence,68 for instance by instantiating an “actual mind” (that “really thinks”); by displaying a degree of autonomy; or by being human-like, general-purpose, or both in the ability to think, reason, or achieve goals across domains (see Table 3).
Terms: The form-centric approach to defining advanced AI accordingly encompasses a variety of terms, including strong AI, autonomous machine (/ artificial) intelligence, general artificial intelligence, human-level AI, foundation model, general-purpose AI system, comprehensive AI services, artificial general intelligence, robust artificial intelligence, AI+, (machine/artificial) superintelligence, superhuman general-purpose AI, and highly-capable foundation models.69
Definitions and themes: While many of these terms are subject to a wide range of different definitions (see Appendix 1A), they combine a range of common themes or patterns (see Table 3).
Suitability of overall definitional approach: In the context of analyzing advanced AI governance, there are both advantages and drawbacks to working with form-centric terms. First, we review five potential benefits.
Benefit (1): Well-established and recognized terms: In the first place, using form-centric terms has the advantage that many of these terms are relatively well established and familiar.72 Out of all the terms surveyed in this report, many form-centric definitions for advanced AI, like strong AI, superintelligence, or AGI, have both the longest track record and the greatest visibility in academic and public debates around advanced AI. Moreover, while some of these terms are relatively niche to philosophical (“AI+”) or technical subcommunities (“CAIS”), many of these terms are in fact the ones used prominently by the main labs developing the most disruptive, cutting-edge AI systems.73 Prima facie, reusing these terms could avoid the problem of having to reinvent the wheel and achieve widespread awareness of and buy-in on newer, more niche terms.
Benefit (2): Readily intuitive concepts: Secondly, form-centric terms evoke certain properties—such as autonomy, adaptability, and human-likeness—which, while certainly not uncontested, may be concepts that are more readily understood or intuited by the public or policymakers than would be more scientifically niche concepts. At the same time, this may also be a drawback, if the ambiguity of many of these terms opens up greater scope for misunderstanding or flawed assumptions to creep into governance debates.
Benefit (3): Enables more forward-looking and anticipatory policymaking towards advanced AI systems and their impacts. Thirdly, because some (though not all) form-centric definitions of advanced AI relate to systems that are perceived (or argued) to appear in the future, using these terms could help extend public attention, debate, and scrutiny to the future impacts of yet more general AI systems which, while their arrival might be uncertain, would likely be enormously impactful. This could help such debates and policies to be less reactive to the impacts of each latest AI model release or incident and start laying the foundations for major policy initiatives. Indeed, centering governance analysis on form-centric terms, even if they are (seen as) futuristic or speculative, can help inform more forward-looking, anticipatory, and participatory policymaking towards the kind of AI systems (and the kind of capabilities and impacts) that may be on the horizon.74
One caveat here is that to consider this a benefit, one has to strongly assume that these futuristic forms of advanced AI systems are in fact feasible and likely near in development. At the same time, this approach need not presume absolute certainty over which of these forms of advanced AI can or will be developed, or on what timelines; rather, well-established risk management approaches75 can warrant some engagement with these scenarios even under uncertainty. To be clear, this need not (and should not) mean neglecting or diminishing policy attention for the impacts of existing AI systems,76 especially as these impacts are already severe and may continue to scale up as AI systems both become more widely implemented and create hazards for existing communities.
Benefit (4): Enables public debate and scrutiny of overarching (professed) direction and destination for AI development. Fourthly, and relatedly, this above advantage to using form-centric terms could still hold, even if one is very skeptical of these types of futuristic AI, because they afford the democratic value of allowing the public and policymakers to chime in on the actual professed long-term goals and aspirations of many (though not all) leading AI labs.77
In this way, the cautious, clear, and reflexive use of terms such as AGI in policy debates could be useful even if one is very skeptical of the actual feasibility of these forms of AI (or believes they are possible but remains skeptical that they will be built anytime soon using extant approaches). This is because there is democratic and procedural value in the public and policymakers being able to hold labs to account for the goals that they in fact espouse and pursue—even if those labs may turn out mistaken about the ability to execute on those plans (in the near term).78 This is especially the case when these are goals that the public might not (currently) agree with or condone.79
Using these “futuristic” terms could therefore help ground public debate over whether the development of these particular systems is even a societal goal they condone, whether society might prefer for labs or society to pursue a different visions for society’s relation to AI technology,80 or (if these systems are indeed considered desirable and legitimate goals) what additional policies or guarantees the world should demand.81
Benefit (5): Technology neutrality: Fifthly, the use of form-centric terms in debates can build in a degree of technology neutrality82 in policy responses, since debates need not focus on the specific engineering or scientific pathways by which one or another highly capable and impactful AI system is pursued or developed. This could make the resulting regulatory frameworks more scalable and future-proof.
At the same time, there are a range of general drawbacks to using (any of these) form-focused definitions in advanced AI governance.
Drawback (1): Connotations and baggage around terms: In the first place, the greater familiarity of some of these terms means that many form-focused terms have become loaded with cultural baggage, associations, or connotations which may mislead, derail, or unduly politicize effective policymaking processes. In particular, many of these terms are contested and have become associated (whether or not necessarily) with particular views or agendas towards building these systems.83 This is a problem because, as discussed previously, the use of different metaphors, frames, and analogies may be irreducible in (and potentially even essential to) the ways that the public and policymakers make sense of regulatory responses. Yet different analogies—and especially the unreflexive use of terms—also have limits and drawbacks and create risks of inappropriate regulatory responses.84
Drawback (2): Significant variance in prominence of terms and constant turnover: In the second place, while some of these terms have held currency at different times in the last decades, many do not see equally common use or recognition in modern debates. For instance, terms such as “strong AI” which dominated early philosophical debates, appear to have fallen slightly out of favor in recent years85 as the emergence and impact of foundation models generally, and generative AI systems specifically, has revived significantly greater attention to terms such as “AGI”. This churn or turnover in definitions may mean that it may not be wise to attempt to pin down a single term or definition right now, since analyses that focus on one particular anticipated form of advanced AI may be more likely to be rendered obsolete. At the same time, this is likely to be a general problem with any concepts or terminology chosen.
Drawback (3): Contested terms, seen as speculative or futuristic: In the third place, while some form-centric terms (such as “GPAIS” or “foundation model”) have been well established in AI policy debates or processes, others, such as “AGI”, “strong AI”, or “superintelligence”, are more future-oriented, referring to advanced AI systems that do not (yet) exist.86 Consequently, many of these terms are contested and seen as futuristic and speculative. This perception may be a challenge, because even if it is incorrect (e.g., such that particular systems like “AGI” will in fact be developed within short timelines or are even in some sense “already here”87), the mere perception that a technology or term is far-off or “speculative” can serve to inhibit and delay effective regulatory or policy action.88
A related but converse risk of using future-oriented terms for advanced AI policy is that it may inadvertently import a degree of technological determinism89 in public and policy discussions, as it could imply that one or another particular forms or architectures of advanced AI (“AGI”, “strong AI”) are not just possible but inevitable—thereby shifting public and policy discussions away from the question of whether we should (or can safely) develop these systems (rather than other, more beneficial architectures)90 towards less ambitious questions over how we should best (safely) reckon with the arrival or development of these technologies.
In response, this drawback could be somewhat mitigated by relying on terms for the forms of advanced AI—such as GPAIS or highly-capable foundation models—that are (a) more present-focused, while (b) not putting any strong presumed ceilings on the capabilities of the systems.
Drawback (4): Definitional ambiguity: In the fourth place, many of these terms, and especially future-oriented terms such as “strong AI”, “AGI”, and “human-level AI”, suffer from definitional ambiguity in that they are used both inconsistently and interchangeably with one another.91
Of course, just because there is no settled or uncontested definition for a term such as “AGI” does not make it prima facie unsuitable for policy or public debate. By analogy, the fact that there can be definitional ambiguity over the content or boundaries of concepts such as “the environment” or “energy” does not render “environmental policy” or “energy policy” meaningless categories or irrelevant frameworks for regulation.92 Nor indeed does outstanding definitional debate mean that any given term, such as AGI, is “meaningless.”93
Nonetheless, the sheer range of contesting definitions for many of these concepts may reflect an underlying degree of disciplinary or philosophical confusion, or at least suggest that, barring greater conceptual clarification and operationalization,94 these terms will lead to continued disagreement. Accordingly, anchoring advanced AI governance to broad terms such as “AGI” may make it harder to articulate appropriately scoped legal obligations for specific actors that will not end up being over- or underinclusive.95
Drawback (5): Challenges in measurement and evaluation: In the fifth place, an underlying and related challenge for the form-centric approach is that (in part due to these definitional disagreements and in part due to deeper reasons) it faces challenges around how to measure or operationalize (progress towards) advanced AI systems.
This matters because effective regulation or governance—especially at the international level96—often requires (scientific and political) consensus around key empirical questions, such as when and how we can know that a certain AI system truly achieves some of the core features (e.g., autonomy, agency, generality, and human-likeness) that are crucial to a given term or concept. In practice, AI researchers often attempt to measure such traits by evaluating an AI system’s ability to pass one or more specific benchmark tests (e.g., the Turing test, the Employment test, the SAT, etc.).97
However, such testing approaches have many flaws or challenges.98 At the practical level, there have been problems with how tests are applied and scored99 and how their results are reported.100 Underlying this is a challenge that the way in which some common AI performance tests are constructed may emphasize nonlinear or discontinuous metrics, which can lead to an overtly strong impression that some model skills are “suddenly” emergent properties (rather than smoothly improving capabilities).101 More fundamentally, there have been challenges to the meaningfulness of applying human-centric tests (such as the bar exam) to AI systems102 and indeed deeper critiques of the construct validity of leading benchmark tests in terms of whether they actually are indicative of progress towards flexible and generalizable AI systems.103
Of course, that does not mean that there may not be further scientific progress towards the operationalization of useful tests for understanding when particular forms of advanced AI such as AGI have been achieved.104 Nor is it to suggest that benchmark and evaluation challenges are unique to form-centric definitions of AI—indeed, they may also challenge many approaches focused on specific capabilities of advanced AIs.105 However, the extant challenges over the operationalization of useful tests mean that overreliance on these terms could muddle debates and inhibit consensus over whether a particular advanced system is within reach (or already being deployed).
Drawback (6): Overt focus on technical achievement of particular forms may make this approach underinclusive of societal impacts or capabilities: In the sixth place, the focus of future-oriented form-centric approaches on the realization of one or another type of advanced AI system (“AGI”, “human-level AI”), might be adequate if the purpose for our definitions is for technical research.106 However, for those whose definitional purpose is to understand AI’s societal impacts (sociotechnical research) or to appropriately regulate AI (regulatory), many form-centric terms may miss the point.
This is because what matters from the perspective of human and societal safety, welfare, and well-being—and from the perspective of law and regulation107—is not the achievement of some fully general capacity in any individual system but rather overall sociotechnical impacts or the emergence of key dangerous capabilities—even if they derive from systems that are not yet (fully) general108 or that develop dangerous emergent capabilities that are not human-like.109 Given all this, there is a risk that taking a solely form-centric approach leaves advanced AI governance vulnerable to a version of the “AI effect,” whereby “real AGI” is always conceived of as being around the corner but rarely as a system already in production.
Suitability of different terms within approach: Given the above, if one does aim to draw on this approach, it may be worth considering which terms manage to gain from the strengths of this approach while reducing some of the pitfalls. In this view, the terms “GPAIS” or “foundation model” may be more suitable in many contexts, as they are recognized as categories of (increasingly) general and competent AI systems of which some versions already exist today. In particular, because (versions) of these terms are already used in ongoing policy debates, they could provide better regulatory handles for governing the development of advanced AI—for instance by their relation to the complex supply chain of modern AI development that contains both upstream and downstream developers and users.110 Moreover, these terms do not presume a ceiling in the system’s capability; accordingly, concepts such as “highly-capable foundation model”,111 “extremely capable foundation model”, or “threshold foundation model” could help policy debates be cognizant of the growing capabilities of these systems while still being more easily understandable for policymakers.112
2. Development-based definitions: Pathways towards advanced AI
Focus of approach: A second cluster of terms focuses on the anticipated or hypothesized scientific pathways or paradigms that could be used to create advanced AI systems. Notably, the goal or target of these pathways is often to build “AGI”-like systems.113
Notes and caveats: Any discussion of proposed pathways towards advanced AI has a number of important caveats. In the first place, many of these proposed paradigms have long been controversial, with pervasive and ongoing disagreement about their scientific foundations and feasibility as paths towards advanced AI (or in particular as paths towards particular forms of advanced AI, such as AGI).114 Secondly, these approaches are not necessarily mutually exclusive, and indeed many labs combine elements from several in their research.115 Thirdly, because the relative and absolute prominence and popularity of many of these paradigms have fluctuated over time and because there are often, as in any scientific field, significant disciplinary gulfs between paradigms, there is highly unequal treatment of these pathways and terms. As such, whereas some paradigms (such as the scaling, reinforcement-learning, and, to some extent, brain-inspired approaches) are reasonably widely known, many of the other approaches and terms listed (such as “seed AI”) may be relatively unknown or even very obscure within the modern mainstream machine learning (ML) community.116
Other taxonomies: There have been various other such attempts to create taxonomies of the main theorized pathways that have been proposed to build or implement advanced AI. For instance, Goertzel and Pennachin have defined four different approaches to creating “AGI”, which to different degrees draw on lessons from the (human) brain or mind.117 More recently, Hannas and others have drawn on this framework and extended it to five theoretical pathways towards “general AI”.118
Further extending such frameworks, one can distinguish between at least 11 proposed pathways towards advanced AI (See Table 4).
Terms: Many of these paradigms or proposed pathways towards advanced AI come with their own assorted terms and definitions (see Appendix 1B). These terms include amongst others de novo AGI, prosaic AGI, frontier (AI) model [compute threshold], [AGI] from evolution, [AGI] from powerful reinforcement learning agents, powerful deep learning models, seed AI, neuroAI, brain-like AGI, neuromorphic AGI, whole-brain emulation, brain-computer interface, [advanced AI based on] a sophisticated embodied agent, or hybrid AI (see Table 4).
Definitions: As noted, these terms can be mapped on 11 proposed pathways towards advanced AI, with their own terms for the resulting advanced AI systems.
Notably, there are significant differences in the prominence of these approaches—and the resources dedicated to them—at different frontier AI labs today. For instance, while some early work on the governance of advanced AI systems focused on AI systems that would (presumably) be built from first principles, bootstrapping,121 or neuro-emulated approaches (see Table 4), much of such work has more recently shifted to focus on understanding the risks from and pathways to aligning and governing advanced AI systems created through computational scaling.
This follows high-profile trends in leading AI labs. While (as discussed above) many research labs are not dedicated to a single paradigm, the last few years (and 2023 in particular) have seen a significant share of resources going towards computational scaling approaches, which have yielded remarkably robust (though not uncontested) performance improvements.122 As a result, the scaling approach has been prominent in informing the approaches of labs such as OpenAI,123 Anthropic,124 DeepMind,125 and Google Brain (now merged into Google DeepMind).126 This approach has also been prominent (though somewhat lagging) in some Chinese labs such as Baidu, Alibaba, Tencent, and the Beijing Institute for General Artificial Intelligence.127 Nonetheless, other approaches continue to be in use. For instance, neuro-inspired approaches have been prominent in DeepMind,128 Meta AI Research,129 and some Chinese130 and Japanese labs,131 and modular cognitive architecture approaches have informed the work by Goertzel’s OpenCog project,132 amongst others.
Suitability of overall definitional approach: In the context of analyzing advanced AI governance, there are both advantages and drawbacks to using concepts that focus on pathways of development.
Amongst the advantages of this approach are:
Benefit (1): Close(r) grounding in actual technical research agendas aimed at advanced AI: Defining advanced AI systems according to their (envisioned) development pathways has the benefit of keeping advanced AI governance debates more closely grounded in existing technical research agendas and programs, rather than the often more philosophical or ambiguous debates over the expected forms of advanced AI systems.
Benefit (2): Technological specificity allowing scoping of regulation to approaches of concern: Relatedly, this also allows better regulatory scoping of the systems of concern. After all, the past decade has seen a huge variety amongst AI techniques and approaches, not just in terms of their efficacy but also in terms of the issues they raise, with particular technical approaches raising distinct (safety, interpretability, robustness) issues.133 At the same time, these correlations might be less relevant in the last few years given the success of scaling-based approaches at creating remarkably versatile and general-purpose systems.
However, taking the pathways-focused approach to defining advanced AI has its own challenges:
Drawback (1): Brittleness as technological specificity imports assumptions about pathways towards advanced AI: The pathway-centric approach may import strong assumptions about what the relevant pathways towards advanced AI are. As such, governance on this basis may not be robust to ongoing changes or shifts in the field.
Drawback (2): Suitability of terms within this approach: Given this, development-based definitions of pathways towards advanced AI seem particularly valuable if the purpose of definition is technical research but may be less relevant if the purpose is sociotechnical analysis or regulation. Technical definitions of AI might therefore provide an important baseline or touchstone for analysis in many other disciplines, but they may not be fully sufficient or analytically enlightening to many fields of study dealing with the societal consequences of the technology’s application or with avenues for governing these.
At any rate, one interesting feature of development-based definitions of advanced AI is that the choice of approach (and term) to focus on has significant and obvious downstream implications for framing the policy agendas for advanced AI—in terms of the policy issues to address, the regulatory “surface” of advanced AI (e.g., the necessary inputs or resources to pursue research along a certain pathway), and the most feasible or appropriate tools. For instance, a focus on neuro-integrationist-produced brain-computer interfaces suggests that policy issues for advanced AI will focus less on questions of value alignment134 and rather around (biomedical) questions of human consent, liability, privacy, (employer) neurosurveillance,135 and/ormorphological freedom.136 A focus on embodiment-based approaches towards robotic agents raises more established debates from robot law.137 Conversely, if one expects that the pathway towards advanced AI still requires underlying scientific breakthroughs, either from first principles or through a hybrid approach, this would imply that very powerful AI systems could be developed suddenly by small teams or labs, which lack large compute budgets.
Similarly, focusing on scaling–based approaches—which seems most suitable given the prominence and success of this approach in driving the recent wave of AI progress—leads to a “compute-based” perspective on the impacts of advanced AI.138 This suggests that the key tools and levers for effective governance should focus on compute governance—provided we assume that this will remain a relevant or feasible precondition for developing frontier AI. For instance, such an approach underpins the compute-threshold definition for frontier AI, which defines advanced AI with reference to particular technical elements or inputs (such as a compute usage or FLOP threshold, dataset size, or parameter count) used in its development.139 While a useful referent, this may be an unstable proxy given that it may not reliably or stably correspond to the particular capabilities of concern.
3. Sociotechnical-change based definitions: Societal impacts of advanced AI
Focus of approach: A third cluster of definitions in advanced AI governance mostly brackets out philosophical questions of the precise form of AI systems or engineering questions of the scientific pathways towards their development. Rather, it aims at defining advanced AI in terms of different levels of societal impacts.
Many concepts in this approach have emerged from scholarship that aimed to abstract away from these architectural questions and rather explore the aggregate societal impacts of advanced AI. This includes work on AI technology’s international, geopolitical impacts140 as well as work on identifying relevant historical precedents for the technology’s societal impacts, strategic stakes, and political economy.141 Examples of this work are those that identified novel categories of unintended “structural” risks from AI as distinct from “misuse” or “accident” risks,142 or taxonomies of the different “problem logics” created by AI systems.143
Terms: The societal-impact-centric approach to defining advanced AI includes a variety of terms, including: (strategic) general-purpose technology, general-purpose military transformation, transformative AI, radically transformative AI, AGI (economic competitiveness definition), and machine superintelligence.
Definitions and themes: While many of these terms are subject to a wide range of different definitions (see Appendix 1C), they again feature a range of common themes or patterns (see Table 5).
Suitability of approach: Concepts within the sociotechnical-change-based approach may be unsuitable iFocus of approach: Finally, a fourth cluster of terms follows a risk-based approach and focuses on critical capabilities, which certain types of advanced AI systems (whatever their underlying form or scientific architecture) might achieve or enable for human users. The development of such capabilities could then mark key thresholds or inflection points in the trajectory of society.
Other taxonomies: Work focused on the significant potential impacts or risks of advanced AI systems is of course hardly new.150 Yet in the past years, as AI capabilities have progressed, there has been renewed and growing concern that these advances are beginning to create key threshold moments where sophisticated AI systems develop capabilities that allow them to achieve or enable highly disruptive impacts in particular domains, resulting in significant societal risks. These risks may be as diverse as the capabilities in question—and indeed discussions of these risks do not always or even mostly presume (as do many form-centric approaches) the development of general capabilities in AI.151 For instance, many argue that existing AI systems may already contribute to catastrophic risks in various domains:152 large language models (LLMs) and automated biological design tools (BDTs) may already be used to enable weaponization and misuse of biological agents,153 the military use of AI systems in diverse roles may inadvertently affect strategic stability and contribute to the risk of nuclear escalation,154 and existing AI systems’ use in enabling granular and at-scale monitoring and surveillance155 may already be sufficient to contribute to the rise of “digital authoritarianism”156 or “AI-tocracy”157, to give a few examples.
As AI systems become increasingly advanced, they may steadily and increasingly achieve or enable further critical capabilities in different domains that could be of special significance. Indeed, as leading LLM-based AI systems have advanced in their general-purpose abilities, they have frequently demonstrated emergent abilities that are surprising even to their developers.158 This has led to growing concern that as these models continue to be scaled up159 some next generation of these systems could develop unexpected but highly dangerous capabilities if not cautiously evaluated.160
What are these critical capabilities?161 In some existing taxonomies, critical capabilities could include AI systems reaching key levels of performance in domains such as cyber-offense, deception, persuasion and manipulation, political strategy, building or gaining access to weapons, long-horizon planning, building new AI systems, situational awareness, self-proliferation, censorship, or surveillance,162 amongst others. Other experts have been concerned about cases where AI systems display increasing tendencies and aptitudes towards controlling or power-seeking behavior.163 Other overviews identify other sets of hazardous capabilities.164 In all these cases, the concern is that advanced AI systems that achieve these capabilities (regardless of whether they are fully general, autonomous, etc.) could enable catastrophic misuse by human owners, or could demonstrate unexpected extreme—even hazardous—behavior, even against the intentions of their human principals.
Terms: Within the risk-based approach, there are a range of domains that could be upset by critical capabilities. A brief survey (see Table 6) can identify at least eight such capability domains—moral/philosophical, economic, legal, scientific, strategic or military, political, exponential, and (extremely) dangerous.165 Namely, these include:166
- Concepts relating to AI systems that achieve or enable critical moral and/or philosophical capabilities include artificial/machine consciousness, digital minds, digital people, sentient artificial intelligence, robot rights catastrophe, (negative) synthetic phenomenology, suffering risks, and adversarial technological maturity.
- Concepts relating to AI systems that achieve or enable critical economic capabilities include high-level machine intelligence, tech company singularity, and artificial capable intelligence (ACI).
- Concepts relating to AI systems that achieve or enable critical legal capabilities include advanced artificial judicial intelligence, technological-legal lock-in, and legal singularity.
- Concepts relating to AI systems that achieve or enable critical scientific capabilities include process-automating science and technology and scientist model.
- Concepts relating to AI systems that achieve or enable critical strategic and/or military capabilities include decisive strategic advantage and singleton.
- Concepts relating to AI systems that achieve or enable critical political capabilities include stable totalitarianism, value lock-in, and actually existing AI.
- Concepts related to AI systems that achieve or enable critical exponential capabilities include intelligence explosion, autonomous replication in the real world, autonomous AI research, and duplicator.
- Concepts relating to AI systems that achieve or enable (extremely) hazardous capabilities include advanced AI, high-risk AI systems, AI systems of concern, prepotent AI, APS systems, WIDGET, rogue AI, runaway AI, frontier (AI) model (under two definitional thresholds), and highly-capable systems of concern.
Definitions and themes: As noted, many of these terms have different definitions (see Appendix 1D). Nonetheless, a range of common themes and patterns can be distilled (see Table 6).
Suitability of approach: There are a range of benefits and drawbacks to defining advanced AI systems by their (critical) capabilities. These include (in no particular order):
Benefit (1): Focuses on key capability development points of most concern: A first benefit of adopting the risk-based definitional approach is that these concepts can be used, alone or in combination, to focus on the key thresholds or transition points in AI development that we most care about—not just the aggregate eventual, long-range societal outcomes nor the (eventual) “final” form of advanced AI, but rather the key intermediate (technical) capabilities that would suffice to create (or enable actors to achieve) significant societal impacts: the points of no return.
Benefit (2): Highlighting risks and capabilities can more precisely inform the public understanding: Ensuring that terms for advanced AI systems clearly center on particular risks or capabilities can help the public and policymakers understand the risks or challenges to be avoided, in a way that is far clearer than terms that focus on very general abilities or which are highly technical (i.e., terms within essence- or development-based approaches, respectively). Such terms may also assist the public in comparing the risks of one model to those posed by another.169
Benefit (3): Generally (but not universally) clearer or more concrete: While some terms within this approach are quite vague (e.g., “singleton”) or potentially difficult to operationalize or test for (e.g., “artificial consciousness”), some of the more specific and narrow terms within this approach could offer more clarity, and less definitional drift, to regulation. While many of them would need significant further clarification before they could be suitable for use in legislative texts (whether domestic laws or international treaties), they may offer the basis for more circumscribed, tightly defined professional cornerstone concepts for such regulation.170
However, there are also a number of potential drawbacks to risk-based definitions.
Drawback (1): Epistemic challenges around “unknown unknown” critical capabilities: One general challenge to this risk-based approach for characterizing advanced AI is that, in the absence of more specific and empirical work, it can be hard to identify and enumerate all relevant risk capabilities in advance (or to know that we have done so). Indeed, aiming to exhaustively list out all key capabilities to watch for could be a futile exercise to undertake.171 At the same time, this is a challenge that is arguably faced in any domain of (technology) risk mitigation, and it does not mean that doing such analysis to the best of our abilities is void. However, this challenge does create an additional hurdle for regulation, as it heightens the chance that if the risk profile of the technology rapidly changes, regulators or existing legal frameworks will be unsure of how or where to classify that model.
Drawback (2): Challenges around comparing or prioritizing between risk capabilities: A related challenge lies in the difficulty of knowing which (potential) capabilities to prioritize for regulation and policy. However, that need not be a general argument against this approach. Instead, it may simply help us make explicit the normative and ethical debates over what challenges to avoid and prioritize.
Drawback (3): Utilizing many parallel terms focused on different risks can increase confusion: One risk for this approach is that while the use of many different terms for advanced AI systems, depending on their specific critical capabilities in particular domains, can make more for appropriate and context-sensitive discussions (and regulation) within those domains, at an aggregate level this may increase the range of terms that regulators and the public have to reckon with and compare between—with the risk that these actors simply drown in the range of terms.
Drawback (5): Outstanding disagreements over appropriate operationalization of capabilities: One further challenge with these terms may lie in the way that some key terms remain contested or debated—and that even clearer terms are not without challenge. For instance, in 2023, the concept of “frontier model” has become subject to increasing debate over its potential adequacy for regulation.172 Notably, there are at least three ways of operationalizing this concept. The first, computational threshold, has been discussed above.173
However, a second operationalization for frontier AI focuses on some relative-capabilities threshold. This approach includes recent proposals to define “frontier AI models” in terms of capabilities relative to other AI systems,174 as models that “exceed the capabilities currently present in the most advanced existing models” or as “models that are both (a) close to, or exceeding, the average capabilities of the most capable existing models.”175 Taking such a comparative approach to defining advanced AI may be useful in combating the easy tendency of observers to normalize or become used to the rapid pace of AI capability progress.176 Yet there may be risks with such a comparative approach, especially when tied to a moving wavefront of “the most capable” existing models, as this could easily impose a need on regulators to engage in constant regulatory updating, as well as creating risks of underinclusivity of some foundation models that did not display hazardous capabilities in their initial evaluations, but which once deployed or shared might be reused or recombined in ways that could create or enable significant harms.177 The risk of embedding this definition of frontier AI in regulation, would be to leave a regulatory gap around significantly harmful capabilities, especially those that are no longer at the technical “frontier,” but which remain unaddressed even so. Indeed, for similar reasons, Seger and others have advocated using the concept “highly-capable foundation models” instead.178
A third approach to defining frontier AI models has instead focused more on identifying a set of static and absolute criteria grounded in particular dangerous capabilities (i.e., a dangerous-capabilities threshold). Such definitions might be useful insofar as they help regulators or consumers identify better when a model crosses a safety threshold and in a way that is less susceptible to slippage or change over time. This could make such concepts more suitable (and resulting regulations less at risk of obsolescence or governance misspecification) than operationalizations of “frontier AI model” that rely on indirect technological metrics (such as compute thresholds) as proxies for these capabilities. Even so, as discussed above, anchoring the “frontier AI model” concept on particular dangerous capabilities leaves open questions around how to best operationalize and create evaluation suites that are able to identify or predict such capabilities ex ante.
Given this, while the risk-based approach may be the most promising ground for defining advanced AI systems from a regulatory perspective, it is clear that not all terms in use in this approach are equally suitable, and many require further operationalization and clarification.
III. Defining the advanced AI governance epistemic community
Beyond the object of concern of “advanced AI” (in all its diverse forms), researchers in the emerging field concerned with the impacts and risks of advanced AI systems have begun to specify a range of other terms and concepts, relating to the tools for intervening in and on the development of advanced AI systems in socially beneficial ways, terms by which this community’s members conceive of the overarching approach or constitution of their field, and theories of change.
1. Defining the tools for policy intervention
First off, those writing about the risks and regulation of AI have proposed a range of terms in describing the tools, practices, or nature of governance interventions that could be used in response (see Table 7).
Like the term “advanced AI”, these terms set out objects of study in scoping the practices or tools of AI governance. They matter insofar as they link these terms to tools for intervention.
Nonetheless, these terms do not capture the methodological dimension of how different approaches to advanced AI governance have approached these issues—nor the normative question of why different research communities have been driven to focus on the challenges from advanced AI in the first place.180
2. Defining the field of practice: Paradigms
Thus, we can next consider different ways that practitioners have defined the field of advanced AI governance.181 Researchers have used a range of terms to describe the field of study that focuses on understanding the trajectory to forms of, or impacts of advanced AI and how to shape these. While these have significant overlaps in practice, it is useful to distinguish some key terms or framings of the overall project (Table 8).
However, while these terms show some different focus and emphasis, and different normative commitments, this need not preclude an overall holistic approach. To be sure, work and researchers in this space often hold diverse expectations about the trajectory, form, or risks of future AI technologies; diverse normative commitments and motivations for studying these; and distinct research methodologies given their varied disciplinary backgrounds and epistemic precommitments.184 However, even so, many of these communities remain united by a shared perception of the technology’s stakes—the shared view that shaping the impacts of AI is and should be a significant global priority.185
As such, one takeaway here is not that scholars or researchers need pick any one of these approaches or conceptions of the field. Rather, there is a significant need for any advanced AI governance field to maintain a holistic approach, which includes many distinct motivations and methodologies. As suggested by Dafoe,
“AI governance would do well to emphasize scalable governance: work and solutions to pressing challenges which will also be relevant to future extreme challenges. Given all this potential common interest, the field of AI governance should be inclusive to heterogenous motivations and perspectives. A holistic sensibility is more likely to appreciate that the missing puzzle pieces for any particular challenge could be found scattered throughout many disciplinary domains and policy areas.”186
In this light, one might consider and frame advanced AI governance as an inclusive and holistic field, concerned with, broadly, “the study and shaping of local and global governance systems—including norms, policies, laws, processes, and institutions—that affect the research, development, deployment, and use of existing and future AI systems, in ways that help the world choose the role of advanced AI systems in its future, and navigate the transition to that world.”
3. Defining theories of change
Finally, researchers in this field have been concerned not just with studying and understanding the strategic parameters of the development of advanced AI systems,187 but also with considering ways to intervene upon it, given particular assumptions or views about the form, trajectory, societal impacts, or risky capabilities of this technology.
Thus, various researchers have defined terms that aim to capture the connection between immediate interventions or policy proposals, and the eventual goals they are meant to secure (see Table 9).
Drawing on these terms, one might also articulate new terms that incorporate elements from the above.196 For instance, one could define a “strategic approach” as a cluster of correlated views on advanced AI governance, encompassing (1) broadly shared assumptions about the key technical and governance parameters of the challenge; (2) a broad theory of victory and impact story about what solving this problem would look like; (3) a broadly shared view of history, with historical analogies to provide comparison, grounding, inspiration, or guidance; and (4) a set of intermediate strategic goals to be pursued, giving rise to near-term interventions that would contribute to reaching these.
Conclusion
The community focused on governing advanced AI systems has developed a rich and growing body of work. However, it has often lacked clarity, not only regarding many key empirical and strategic questions, but also regarding many of its fundamental terms. This includes different definitions for the relevant object of analysis—that is, species of “advanced AI”—as well as different framings for the instruments of policy, different paradigms or approaches to the field itself, and distinct understandings of what it means to have a theory of change to guide action.
This report has reviewed a range of terms for different analytical categories in the field. It has discussed three different purposes for seeking definitions for core terms, and why and how (under a “regulatory” purpose) the choice of terms matters to both the study and practice of AI governance. It then reviewed analytical definitions of advanced AI used across different clusters which focus on the forms or design of advanced AI systems, the (hypothesized) scientific pathways towards developing these systems, the technology’s broad societal impacts, and the specific critical capabilities achieved by particular AI systems. The report then briefly reviewed analytical definitions of the tools for intervention, such as “policy” and governance”, before discussing definitions of the field and community itself and definitions for theories of change by which to prioritize interventions.
This field of advanced AI governance has shown a penchant for generating many concepts, with many contesting definitions. Of course, while any emerging field will necessarily engage in a struggle to define itself, this field has seen a particularly broad range of terms, perhaps reflecting the disciplinary range. Eventually, the community may need to more intentionally and deliberately commit to some terms. In the meantime, those who engage in debate within and beyond the field should at least have greater clarity about the ways that these concepts are used and understood, and about the (regulatory) implications of some of these terms. This report has aimed to provide such greater clarity in order to help provide greater context for more informed and clear discussions about questions in and around the field.
Appendix 1: Lists of definitions for advanced AI terms
This appendix provides a detailed list of definitions for advanced AI systems, with sources. These may be helpful for readers to explore work in this field in more detail; to understand the longer history and evolution of many terms; and to consider the strengths and drawbacks of particular terms, and of specific language, for use in public debate, policy formulation, or even in direct legislative texts.
1.A. Definitions focused on the form of advanced AI
Different definitional approaches emphasize distinct aspects or traits that would characterize the form of advanced AI systems—such as that it is ‘mind-like’, performs ‘autonomously’, ‘is general-purpose’, ‘performs like a human’, ‘performs general-purpose like a human’, etc. However, it should be noted that there is significant overlap, and many of these terms are often (whether or not correctly) used interchangeably.
Advanced AI is mind-like & really thinks
- Strong AI
- An “appropriately programmed computer [that] really is a mind, in the sense that computers given the right programs can be literally said to understand and have other cognitive states.”198
- “The assertion that machines could possibly act intelligently (or, perhaps better, act as if they were intelligent) is called the ‘weak AI’ hypothesis by philosophers, and the assertion that machines that do so are actually thinking (as opposed to simulating thinking) is called the ‘strong AI’ hypothesis.”199
- “the combination of Artificial General Intelligence/Human-Level AI and Superintelligence.”200
Advanced AI is autonomous
- Autonomous machine intelligence: “intelligent machines that learn more like animals and humans, that can reason and plan, and whose behavior is driven by intrinsic objectives, rather than by hard-wired programs, external supervision, or external rewards.”201
- Autonomous artificial intelligence: “artificial intelligence that can adapt to external environmental challenges. Autonomous artificial intelligence can be similar to animal intelligence, called (specific) animal-level autonomous artificial intelligence, or unrelated to animal intelligence, called non-biological autonomous artificial intelligence.”202
General artificial intelligence: “broadly capable AI that functions autonomously in novel circumstances”.203
Advanced AI is human-like
- Human-level AI (HLAI)
- “systems that operate successfully in the common sense informatic situation [defined as the situation where] the known facts are incomplete, and there is no a priori limitation on what facts are relevant. It may not even be decided in advance what phenomena are to be taken into account. The consequences of actions cannot be fully determined. The common sense informatic situation necessitates the use of approximate concepts that cannot be fully defined and the use of approximate theories involving them. It also requires nonmonotonic reasoning in reaching conclusions.”204
- “machines exhibiting true human-level intelligence should be able to do many of the things humans are able to do. Among these activities are the tasks or ‘jobs’ at which people are employed. I suggest we replace the Turing test by something I will call the ‘employment test.’ To pass the employment test, AI programs must… [have] at least the potential [to completely automate] economically important jobs.”205
- “AI which can reproduce everything a human can do, approximately. […] [this] can mean either AI which can reproduce a human at any cost and speed, or AI which can replace a human (i.e. is as cheap as a human, and can be used in the same situations.)”206
- “An artificial intelligence capable of matching humans in every (or nearly every) sphere of intellectual activity.”207
Advanced AI is general-purpose
- Foundation model
- “models trained on broad data at scale […] that are adaptable to a wide range of downstream tasks.”208
- “AI systems with broad capabilities that can be adapted to a range of different, more specific purposes. […] the original model provides a base (hence ‘foundation’) on which other things can be built.”209
- General-purpose AI systems (GPAIS)
- “an AI system that can be used in and adapted to a wide range of applications for which it was not intentionally and specifically designed.”210
- “An AI system that can accomplish or be adapted to accomplish a range of distinct tasks, including some for which it was not intentionally and specifically trained.”211
- “An AI system that can accomplish a range of distinct valuable tasks, including some for which it was not specifically trained.”212
- See also “general-purpose AI models”: “AI models that are designed for generality of their output and have a wide range of possible applications.”213
- Comprehensive AI services (CAIS)
“asymptotically recursive improvement of AI technologies in distributed systems [which] contrasts sharply with the vision of self-improvement internal to opaque, unitary agents. […] asymptotically comprehensive, superintelligent-level AI services that—crucially—can include the service of developing new services, both narrow and broad, [yielding] a model of flexible, general intelligence in which agents are a class of service-providing products, rather than a natural or necessary engine of progress in themselves.”214
Advanced AI is general-purpose & of human-level performance
- Artificial general intelligence (AGI) [task performance definitions]215
- “systems that exhibit the broad range of general intelligence found in humans.”216
- “Artificial intelligence that is not specialized to carry out specific tasks, but can learn to perform as broad a range of tasks as a human.”217
- AI systems with “the ability to achieve a variety of goals, and carry out a variety of tasks, in a variety of different contexts and environments.”218
- AI systems which “can reason across a wide range of domains, much like the human mind.”219
- “machines designed to perform a wide range of intelligent tasks, think abstractly and adapt to new situations.”220
- “AI that is capable of solving almost all tasks that humans can solve.”221
- “AIs that can generalize well enough to produce human-level performance on a wide range of tasks, including abstract low-data tasks.”222
- “The AI that […] can do most everything we humans can do, and possibly much more.”223
- “[a]n AI that has a level of intelligence that is either equivalent to or greater than that of human beings or is able to cope with problems that arise in the world that surrounds human beings with a degree of adequacy at least similar to that of human beings.”.224
- “an agent that has a world model that’s vastly more accurate than that of a human in, at least, domains that matter for competition over resources, and that can generate predictions at a similar rate or faster than a human.”225
- “type of AI system that addresses a broad range of tasks with a satisfactory level of performance [or in a stronger sense] systems that not only can perform a wide variety of tasks, but all tasks that a human can perform.”226
- “[AI with] cognitive capabilities fully generalizing those of humans.”227
- See also the subdefinition of autonomous AGI (AAGI) as “an autonomous artificial agent with the ability to do essentially anything a human can do, given the choice to do so—in the form of an autonomously/internally determined directive—and an amount of time less than or equal to that needed by a human.”228
- “a machine-based system that can perform the same general-purpose reasoning and problem-solving tasks humans can.”229
- “an AI system that equals or exceeds human intelligence in a wide variety of cognitive tasks.”230
- “AI systems that achieve or exceed human performance across a wide range of cognitive tasks”.231
- “hypothetical type of artificial intelligence that would have the ability to understand or learn any intellectual task that a human being can.”232
- “a shorthand for any intelligence […] that is flexible and general, with resourcefulness and reliability comparable to (or beyond) human intelligence.”233
- “systems that demonstrate broad capabilities of intelligence as […] [a very general mental capability that, among other things, involves the ability to reason, plan, solve problems, think abstractly, comprehend complex ideas, learn quickly and learn from experience], with the additional requirement, perhaps implicit in the work of the consensus group, that these capabilities are at or above human-level.”234
- “autonomous artificial intelligence that reaches Human-level intelligence. It can adapt to external environmental challenges and complete all tasks that humans can accomplish, achieving human-level intelligence in all aspects.”235
Robust artificial intelligence: “intelligence that, while not necessarily superhuman or self-improving, can be counted on to apply what it knows to a wide range of problems in a systematic and reliable way, synthesizing knowledge from a variety of sources such that it can reason flexibly and dynamically about the world, transferring what it learns in one context to another, in the way that we would expect of an ordinary adult.”236
Advanced AI is general-purpose & beyond-human-performance
- AI+: “artificial intelligence of greater than human level (that is, more intelligent than the most intelligent human)”237
- (Machine/Artificial) superintelligence (ASI):
- “an intellect that is much smarter than the best human brains in practically every field, including scientific creativity, general wisdom and social skills.”238
- “any intellect that greatly exceeds the cognitive performance of humans in virtually all domains of interest.”239
- “an AI significantly more intelligent than humans in all respects.”240
- “Artificial intelligence that can outwit humans in every (or almost every) intellectual sphere.”241
- “future AI systems dramatically more capable than even AGI.”242
- “Artificial General Intelligence that has surpassed humans in all aspects of human intelligence.”243
- “AI that might be as much smarter than us as we are smarter than insects.”244
- See also “machine superintelligence” [form and impact]: “general artificial intelligence greatly outstripping the cognitive capacities of humans, and capable of bringing about revolutionary technological and economic advances across a very wide range of sectors on timescales much shorter than those characteristic of contemporary civilization.”245
- Superhuman general-purpose AI (SGPAI): “general purpose AI systems […] that are simultaneously as good or better than humans across nearly all tasks.”246
- Highly capable foundation models: “Foundation models that exhibit high performance across a broad domain of cognitive tasks, often performing the tasks as well as, or better than, a human.”247
1.B. Definitions focused on the pathways towards advanced AI
First-principles pathways: “De novo AGI”
Pathways based on new fundamental insights in computer science, mathematics, algorithms, or software, producing advanced AI systems that may, but need not mimic human cognition.248
- De novo AGI: “AGI built from the ground up.”249
Scaling pathways: “Prosaic AGI”, “frontier (AI) model” [compute threshold]
Approaches based on “brute forcing” advanced AI,250 by running (one or more) existing AI approaches (such as transformer-based LLMs)251 with increasingly more computing power and/or training data, as per the “scaling hypothesis.”252
- Prosaic AGI: AGI “which can replicate human behavior but doesn’t involve qualitatively new ideas about ‘how intelligence works.’”253
- Frontier (AI) model [compute threshold]:254
- “foundation model that is trained with more than some amount of computational power—for example, 10^26 FLOP.”255
- “models within one order of magnitude of GPT-4 (>2e24 FLOP).”256
Evolutionary pathways: “[AGI] from evolution”
Approaches based on algorithms competing to mimic the evolutionary brute search process that produced human intelligence.257
- [AGI] from evolution: “[AGI re-evolved through] genetic algorithms on computers that are sufficiently fast to recreate on a human timescale the same amount of cumulative optimization power that the relevant processes of natural selection instantiated throughout our evolutionary past.”258
Reward-based pathways: “[AGI] from powerful reinforcement learning agents”, “powerful deep learning models”
Approaches based on running reinforcement learning systems with simple rewards in rich environments.
- [AGI] from powerful reinforcement learning agents: “powerful reinforcement learning agents, when placed in complex environments, will in practice give rise to sophisticated expressions of intelligence.”259
Powerful deep learning models: “a powerful neural network model [trained] to simultaneously master a wide variety of challenging tasks (e.g. software development, novel-writing, game play, forecasting, etc.) by using reinforcement learning on human feedback and other metrics of performance.”260
Bootstrapping pathways:261 “Seed AI”
Approaches that pursue a minimally intelligent core system capable of subsequent recursive (self)-improvement,262 potentially leveraging hardware or data “overhangs.”263
- Seed AI:
- “an AI designed for self-understanding, self-modification, and recursive self-improvement.”264
- “a strongly self-improving process, characterized by improvements to the content base that exert direct positive feedback on the intelligence of the underlying improving process.”265
- “The first AI in a series of recursively self-improving systems.”266
Neuro-emulated pathways: “Whole-brain-emulation” (WBE)
Approaches that aim to digitally simulate or recreate the states of human brains at fine-grained level.
- Whole-brain-emulation (WBE):
- “software (and possibly dedicated non-brain hardware) that models the states and functional dynamics of a brain at a relatively fine-grained level of detail.”271
- “The process of making an exact computer-simulated copy of the brain of a particular animal (e.g., a particular human).”272
- Digital people [emulation definition]: “a computer simulation of a specific person, in a virtual environment […] perhaps created via mind uploading (simulating human brains) [or] entities unlike us in many ways, but still properly thought of as ‘descendants’ of humanity.”273
- See also related terms: “Ems”274 or “uploads”.
Neuro-integrationist pathways: “Brain-computer-interfaces” (BCI)
Approaches to create advanced AI, based on merging components of human and digital cognition.
- Brain-computer-interfaces (BCI): “use brain-computer interfaces to position both elements, human and machine, to achieve (or overachieve) human goals.”275
Embodiment pathways:276 “Embodied agent”
Based on providing the AI system with a robotic physical “body” to ground cognition and enable it to learn from direct experience of the world.277
- “an embodied agent (e.g., a robot) which learns, through interaction and exploration, to creatively solve challenging tasks within its environment.”278
Modular cognitive architecture pathways
Used in various fields, including in robotics, where researchers integrate well-tested but distinct state-of-the-art modules (perception, reasoning, etc.) to improve agent performance without independent learning.279
- No clear single term.
Hybrid pathways
Approaches that rely on combining deep neural network-based approaches to AI with other paradigms (such as symbolic AI).
- Hybrid AI: “hybrid, knowledge-driven, reasoning-based approach, centered around cognitive models.”280
1.C. Definitions focused on the aggregate societal impacts of advanced AI
(Strategic) general-purpose technology (GPT)
- “[AI systems] having an unusually broad and deep impact on the world, comparable to that of electricity, the internal combustion engine, and computers.”281
- This has been further operationalized as: “[this] need not emphasize only agent-like AI or powerful AI systems, but instead can examine the many ways even mundane AI could transform fundamental parameters in our social, military, economic, and political systems, from developments in sensor technology, digitally mediated behavior, and robotics. AI and associated technologies could dramatically reduce the labor share of value and increase inequality, reduce the costs of surveillance and repression by authorities, make global market structure more oligopolistic, alter the logic of the production of wealth, shift military power, and undermine nuclear stability.”282
- See also strategic general-purpose technology: “A general purpose technology which has the potential to deliver vast economic value and substantially affect national security, and is consequently of central political interest to states, firms, and researchers.”283
General-purpose military transformation (GMT)
- The process by which general-purpose technologies (such as electricity and AI) “influence military effectiveness through a protracted, gradual process that involves a broad range of military innovations and overall industrial productivity growth.”284
Transformative AI (TAI):285
- “potential future AI that precipitates a transition comparable to (or more significant than) the agricultural or industrial revolution.”286
- “AI powerful enough to bring us into a new, qualitatively different future.”287
- “software which causes a tenfold acceleration in the rate of growth of the world economy (assuming that it is used everywhere that it would be economically profitable to use it).”288
- “AI that can go beyond a narrow task … but falls short of achieving superintelligence.”289
- “a range of possible advances with potential to impact society in significant and hard-to-reverse ways.”290
- “Any AI technology or application with potential to lead to practically irreversible change that is broad enough to impact most important aspects of life and society.”291
Radically transformative AI (RTAI)
- “any AI technology or application which meets the criteria for TAI, and with potential impacts that are extreme enough to result in radical changes to the metrics used to measure human progress and well-being, or to result in reversal of societal trends previously thought of as practically irreversible. This indicates a level of societal transformation equivalent to that of the agricultural or industrial revolutions.”292
AGI [economic competitiveness definition]
- “highly autonomous systems that outperform humans at most economically valuable work.”293
- “AI systems that power a comparably profound transformation (in economic terms or otherwise) as would be achieved in [a world where cheap AI systems are fully substitutable for human labor].”294
- “future machines that could match and then exceed the full range of human cognitive ability across all economically valuable tasks.”295
Machine superintelligence [form & impact definition]
“general artificial intelligence greatly outstripping the cognitive capacities of humans, and capable of bringing about revolutionary technological and economic advances across a very wide range of sectors on timescales much shorter than those characteristic of contemporary civilization”296
1.D. Definitions focused on critical capabilities of advanced AI systems
Systems with critical moral and/or philosophical capabilities
- Artificial/Machine consciousness:
- “machines that genuinely exhibit conscious awareness.”297
- “Weakly construed, the possession by an artificial intelligence of a set of cognitive attributes that are associated with consciousness in humans, such as awareness, self-awareness, or cognitive integration. Strongly construed, the possession by an AI of properly phenomenological states, perhaps entailing the capacity for suffering.”298
- Digital minds: “machine minds with conscious experiences, desires, and capacity for reasoning and autonomous decision-making […] [which could] enjoy moral status, i.e. rather than being mere tools of humans they and their interests could matter in their own right.”299
- Digital people [capability definition]: “any digital entities that (a) had moral value and human rights, like non-digital people; (b) could interact with their environments with equal (or greater) skill and ingenuity to today’s people.”300
- Sentient artificial intelligence: “artificial intelligence (capable of feeling pleasure and pain).”301
- Robot rights catastrophe: The point where AI systems are sufficiently advanced that “some people reasonably regard [them] as deserving human or humanlike rights. [while] Other people will reasonably regard these systems as wholly undeserving of human or humanlike rights. […] Given the uncertainties of both moral theory and theories about AI consciousness, it is virtually impossible that our policies and free choices will accurately track the real moral status of the AI systems we create. We will either seriously overattribute or seriously underattribute rights to AI systems—quite possibly both, in different ways. Either error will have grave moral consequences, likely at a large scale. The magnitude of the catastrophe could potentially rival that of a world war or major genocide.”302
- (Negative) synthetic phenomenology: “machine consciousness [that] will have preferences of their own, that […] will autonomously create a hierarchy of goals, and that this goal hierarchy will also become a part of their phenomenal self-model […] [such that they] will be able to consciously suffer,”303 creating a risk of an “explosion of negative phenomenology” (ENP) (“Suffering explosion”).304
- Suffering risks: “[AI that brings] about severe suffering on an astronomical scale, vastly exceeding all suffering that has existed on Earth so far.”305
- Adversarial technological maturity:
- “the point where there are digital people and/or (non-misaligned) AIs that can copy themselves without limit, and expand throughout space [creating] intense pressure to move – and multiply (via copying) – as fast as possible in order to gain more influence over the world.”306
- “a world in which highly advanced technology has already been developed, likely with the help of AI, and different coalitions are vying for influence over the world.”307
Systems with critical economic capabilities308
- High-level machine intelligence (HLMI):
- “unaided machines [that] can accomplish every task better and more cheaply than human workers.”309
- “an AI system (or collection of AI systems) that performs at the level of an average human adult on key cognitive measures required for economically relevant tasks.”310
- “The spectrum of advanced AI capabilities from next-generation AI systems to artificial general intelligence (AGI). Often used interchangeably with advanced AI.”311
- Tech company singularity: “a transition of a technology company into a fully general tech company [defined as] a technology company with the ability to become a world-leader in essentially any industry sector, given the choice to do so—in the form of agreement among its Board and CEO—with around one year of effort following the choice.”312
- Artificial capable intelligence (ACI):
- “AI [that] can achieve complex goals and tasks with minimal oversight.”313
- “a fast-approaching point between AI and AGI: ACI can achieve a wide range of complex tasks but is still a long way from being fully general.”314
Systems with critical legal capabilities
- Advanced artificial judicial intelligence (AAJI): “an artificially intelligent system that matches or surpasses human decision-making in all domains relevant to judicial decision-making.”315
- Technological-legal lock-in: “hybrid human/AI judicial systems [which] risk fostering legal stagnation and an attendant loss of judicial legitimacy.”316
- Legal singularity: “when the accumulation of a massive amount of data and dramatically improved methods of inference make legal uncertainty obsolete. The legal singularity contemplates complete law. […] the elimination of legal uncertainty and the emergence of a seamless legal order, which is universally accessible in real time.”317
Systems with critical scientific capabilities
- Process-automating science and technology (PASTA): “AI systems that can essentially automate all of the human activities needed to speed up scientific and technological advancement.”318
- Scientist model: “a single unified transformative model […] which has flexible general-purpose research skills.”319
Systems with critical strategic or military capabilities320
- Decisive strategic advantage: “a position of strategic superiority sufficient to allow an agent to achieve complete world domination.”321
- Singleton: [AI capabilities sufficient to support] “a world order in which there is a single decision-making agency at the highest level.”322
Systems with critical political capabilities
- Stable totalitarianism: “AI [that] could enable a relatively small group of people to obtain unprecedented levels of power, and to use this to control and subjugate the rest of the world for a long period of time (e.g. via advanced surveillance).”323
- Value lock-in:
- “an event [such as the use of AGI] that causes a single value system, or set of value systems, to persist for an extremely long time.”324
- “AGI [that] would make it technologically feasible to (i) perfectly preserve nuanced specifications of a wide variety of values or goals far into the future, and (ii) develop AGI-based institutions that would (with high probability) competently pursue any such values for at least millions, and plausibly trillions, of years.”325
Actually existing AI (AEAI): A paradigm by which the broader ecosystem of AI development, on current trajectories, may produce harmful political outcomes, because “AI as currently funded, constructed, and concentrated in the economy—is misdirecting technological resources towards unproductive and dangerous outcomes. It is driven by a wasteful imitation of human comparative advantages and a confused vision of autonomous intelligence, leading it toward inefficient and harmful centralized architectures.”326
Systems with critical exponential capabilities
- Intelligence explosion:327
- “explosion to ever greater levels of intelligence, as each generation of machines creates more intelligent machines in turn.”328
- “a chain of events by which human-level AI leads, fairly rapidly, to intelligent systems whose capabilities far surpass those of biological humanity as a whole.”329
- Autonomous replication in the real world: “A model that is unambiguously capable of replicating, accumulating resources, and avoiding being shut down in the real world indefinitely, but can still be stopped or controlled with focused human intervention.”330
- Autonomous AI research: “A model for which the weights would be a massive boost to a malicious AI development program (e.g. greatly increasing the probability that they can produce systems that meet other criteria for [AI Safety Level]-4 in a given timeframe).”331
Duplicator: [digital people or particular forms of advanced AI that would allow] “the ability to make instant copies of people (or of entities with similar capabilities) [leading to] explosive productivity.”332
Systems with critical hazardous capabilities
Systems that pose or enable critical levels of (extreme or even existential) risk,333 regardless of whether they demonstrate a full range of human-level/like cognitive abilities.
- Advanced AI:
- “systems substantially more capable (and dangerous) than existing […] systems, without necessarily invoking specific generality capabilities or otherwise as implied by concepts such as ‘Artificial General Intelligence.’”334
- “Systems that are highly capable and general purpose.”335
- High-risk AI system”:
- An AI system that is both “(a) … intended to be used as a safety component of a product, or is itself a product covered by the Union harmonisation legislation […] (b) the product whose safety component is the AI system, or the AI system itself as a product, is required to undergo a third-party conformity assessment with a view to the placing on the market or putting into service of that product […].”336
- “AI systems that are used to control the operation of critical infrastructure… [in particular] highly capable systems, increasingly autonomous systems, and systems that cross the digital-physical divide.”337
- AI systems of concern: “highly capable AI systems that are […] high in ‘Property X’ [defined as] intrinsic characteristics such as agent-like behavior, strategic awareness, and long-range planning.”338
- Prepotent AI: “an AI system or technology is prepotent […] (relative to humanity) if its deployment would transform the state of humanity’s habitat—currently the Earth—in a manner that is at least as impactful as humanity and unstoppable to humanity.”339
- APS Systems: AI systems with “(a) Advanced capabilities, (b) agentic Planning, and (c) Strategic awareness.”340 These systems may risk instantiating “MAPS”—“misaligned, advanced, planning, strategically aware” systems;341 also called “power-seeking AI”.342
- WIDGET: “Wildly Intelligent Device for Generalized Expertise and Technical Skills.”343
- “Rogue AI:
- “an autonomous AI system that could behave in ways that would be catastrophically harmful to a large fraction of humans, potentially endangering our societies and even our species or the biosphere.”344
- “a powerful and dangerous AI [that] attempts to execute harmful goals, irrespective of whether the outcomes are intended by humans.”345
- Runaway AI: “advanced AI systems that far exceed human capabilities in many key domains, including persuasion and manipulation; military and political strategy; software development and hacking; and development of new technologies […] [these] superhuman AI systems might be designed to autonomously pursue goals in the real world.”346
- “Frontier (AI) model [relative-capabilities threshold]:
- “large-scale machine-learning models that exceed the capabilities currently present in the most advanced existing models, and can perform a wide variety of tasks.”347
- “highly capable general-purpose AI models that can perform a wide variety of tasks and match or exceed the capabilities present in today’s most advanced models.”348
- Frontier (AI) model [unexpected-capabilities threshold]:
- “Highly capable foundation models, which could have dangerous capabilities that are sufficient to severely threaten public safety and global security. Examples of capabilities that would meet this standard include designing chemical weapons, exploiting vulnerabilities in safety-critical software systems, synthesising persuasive disinformation at scale, or evading human control.”349
- “models that are both (a) close to, or exceeding, the average capabilities of the most capable existing models, and (b) different from other models, either in terms of scale, design (e.g. different architectures or alignment techniques), or their resulting mix of capabilities and behaviours.”350
- Highly-capable systems of concern:
- “Highly capable foundation models […] capable of exhibiting dangerous capabilities with the potential to cause significant physical and societal-scale harm”351
Appendix 2: Lists of definitions for policy tools and field
2.A. Terms for tools for intervention
Strategy352
- AI strategy research: “the study of how humanity can best navigate the transition to a world with advanced AI systems (especially transformative AI), including political, economic, military, governance, and ethical dimensions.”353
- AI strategy: “the study of big picture AI policy questions, such as whether we should want AI to be narrowly or widely distributed and which research problems ought to be prioritized.”354
- Long-term impact strategies: “shape the processes that will eventually lead to strong AI systems, and steer them in a safer direction.”355
- Strategy: “the activity or project of doing research to inform interventions to achieve a particular goal. […] AI strategy is strategy from the perspective that AI is important, focused on interventions to make AI go better.”356
- AI macrostrategy: “the study of high level questions having to do with prioritizing the use of resources on the current margin in order to achieve good AI outcomes.”357
Policy
- AI policy: “concrete soft or hard governance measures which may take a range of forms such as principles, codes of conduct, standards, innovation and economic policy or legislative approaches, along with underlying research agendas, to shape AI in a responsible, ethical and robust manner.”358
- AI policymaking strategy: “A research field that analyzes the policymaking process and draws implications for policy design, advocacy, organizational strategy, and AI governance as a whole.”359
Governance
- AI governance:
- “AI governance (or the governance of artificial intelligence) is the study of norms, policies, and institutions that can help humanity navigate the transition to a world with advanced artificial intelligence. This includes a broad range of subjects, from global coordination around regulating AI development to providing incentives for corporations to be more cautious in their AI research.”360
- “local and global norms, policies, laws, processes, politics, and institutions (not just governments) that will affect social outcomes from the development and deployment of AI systems.”361
- “shifting and setting up incentive structures for actions to be taken to achieve a desired outcome [around AI].”362
- “identifying and enforcing norms for AI developers and AI systems themselves to follow. […] AI governance, as an area of human discourse, is engaged with the problem of aligning the development and deployment of AI technologies with broadly agreeable human values.”363
- “the study or practice of local and global governance systems—including norms, policies, laws, processes, and institutions—govern or should govern AI research, development, deployment, and use.”364
- Collaborative governance of AI technology: “collaboration between stakeholders specifically in the legal governance of AI technology. The stakeholders could include representatives of governments, companies, or other established groups.”365
- AGI safety and governance practices: “internal policies, processes, and organizational structures at AGI labs intended to reduce risk.”366
2.B. Terms for the field of practice
AI governance
- “the field of AI governance studies how humanity can best navigate the transition to advanced AI systems, focusing on the political, economic, military, governance, and ethical dimensions.”367
- “AI governance concerns how humanity can best navigate the transition to a world with advanced AI systems. It relates to how decisions are made about AI, and what institutions and arrangements would help those decisions to be made well.”368
- “AI governance refers (1) descriptively to the policies, norms, laws, and institutions that shape how AI is built and deployed, and (2) normatively to the aspiration that these promote good decisions (effective, safe, inclusive, legitimate, adaptive). […] governance consists of much more than acts of governments, also including behaviors, norms, and institutions emerging from all segments of society. In one formulation, the field of AI governance studies how humanity can best navigate the transition to advanced AI systems.”369
Transformative AI governance
- “[governance that] includes both long-term AI and any nearer-term forms of AI that could affect the long-term future [and likewise] includes governance activities in both the near-term and the long-term that could affect the long-term future.”370
Longterm(ist) AI governance
- Long-term AI governance: “[governance that] includes both long-term AI and any nearer-term forms of AI that could affect the long-term future [and likewise] includes governance activities in both the near-term and the long-term that could affect the long-term future.”371
- Longtermist AI governance:
- “longtermism-motivated AI governance / strategy / policy research, practice, advocacy, and talent-building.”372
- “the subset of [AI governance] work that is motivated by a concern for the very long-term impacts of AI. This overlaps significantly with work aiming to govern transformative AI (TAI).”373
- “longtermist AI governance […] which is intellectually and sociologically related to longtermism […] explicitly prioritizes attention to considerations central to the long-term trajectory for humanity, and thus often to extreme risks (as well as extreme opportunities).”374
Appendix 3: Auxiliary definitions and terms
Beyond this, it is also useful to clarify a range of auxiliary definitions that can support analysis in the advanced AI governance field. These include, but are not limited to:375
- Strategic parameters: Features of the world that significantly determine the strategic nature of the advanced AI governance challenge. These parameters serve as highly decision-relevant or even crucial considerations, determining which interventions or solutions are appropriate, necessary, viable, or beneficial to addressing the advanced AI governance challenge; accordingly, different views of these underlying strategic parameters constitute underlying cruxes for different theories of actions and approaches. This encompasses different types of parameters:
- technical parameters (e.g., advanced AI development timelines and trajectories, threat models, and feasibility of alignment solution),
- deployment parameters (e.g., the distribution and constitution of actors developing advanced AI systems), and
- governance parameters (e.g., the relative efficacy and viability of different governance instruments).376
- Key actor: An actor whose key decisions will have significant impact on shaping the outcomes from advanced AI, either directly (first-order), or by strongly affecting such decisions made by other actors (second-order).
- Key decision: A choice or series of choices by a key actor to use its levers of governance, in ways that directly affect beneficial advanced AI outcomes, and which are hard to reverse. This can include direct decisions about deployment or testing during a critical moment, but also includes many upstream decisions (such as over whether to initiate risky capabilities).
- Lever (of governance):377 A tool or intervention that can be used by key actors to shape or affect (1) the primary outcome of advanced AI development, (2) key strategic parameters of advanced AI governance, and (3) other key actors’ choices or key decisions.
- Pathway (to influence): A tool or intervention by which other actors (that are not themselves key actors) can affect, persuade, induce, incentivize, or require key actors to make certain key decisions. This can include interventions that ensure that certain levers of control are (not) used, or used in particular ways.
- (Decision-relevant) asset: Resources that can be used by other actors in pursuing pathways of influence to key actors, and that aim to induce how these key actors make key decisions (e.g., about whether or how to use their levers). This includes new technical research insights, worked-out policy products; networks of direct influence, memes, or narratives;
- (Policy) product: A subclass of assets; specific legible proposals that can be presented to key actors.
- Critical moment(s): High-leverage378 moments where high-impact decisions are made by some actors on the basis of the available decision-relevant assets, which affect whether beneficial advanced AI outcomes are within reach. These critical moments may occur during a public “AI crunch time,”379 but they may also occur potentially long in advance (if they lock in choices or trajectories).
- “Beneficial” AI outcomes: The desired and/or non-catastrophic societal outcomes from AI technology. This is a complex normative question, which one may aim to derive by some external moral standard or philosophy,380 through social choice theory,381 or through some legitimate (e.g., democratic) process by key stakeholders themselves.382 However, this concept is often undertheorized and needs significantly more work, scholarship, and normative and public deliberation.
Also in this series:
- Maas, Matthijs, and Villalobos, José Jaime. “International AI institutions: A literature review of models, examples, and proposals.” Institute for Law & AI, AI Foundations Report 1. (September 2023). https://law-ai.org/international-ai-institutions
- Maas, Matthijs, “AI is like… A literature review of AI metaphors and why they matter for policy.” Institute for Law & AI. AI Foundations Report 2. (October 2023). https://law-ai.org/ai-policy-metaphors
- Maas, Matthijs, “Advanced AI governance: A literature review.” Institute for Law & AI, AI Foundations Report 4. (November 2023). https://law-ai.org/advanced-ai-gov-litrev