The governance misspecification problem
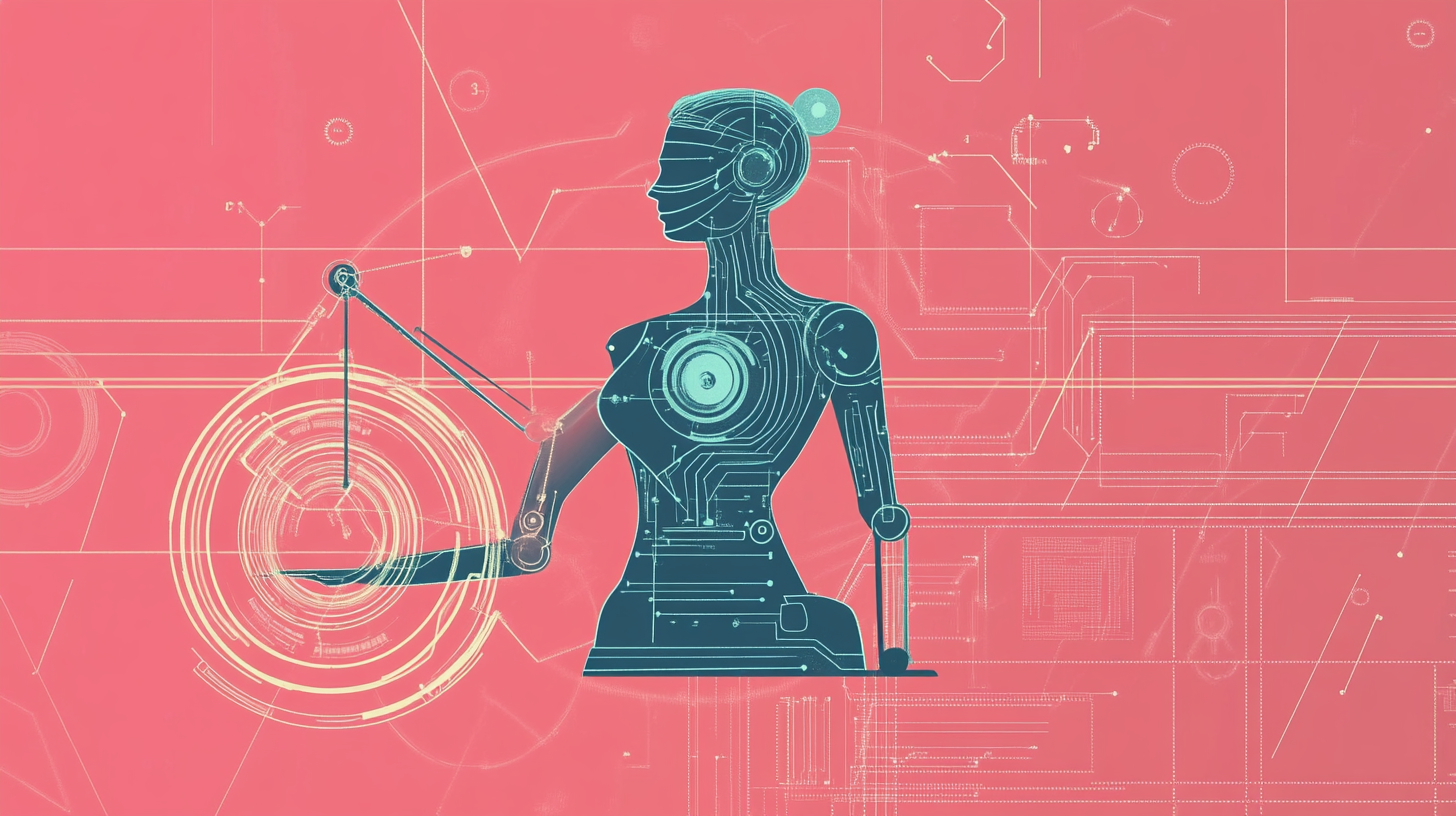
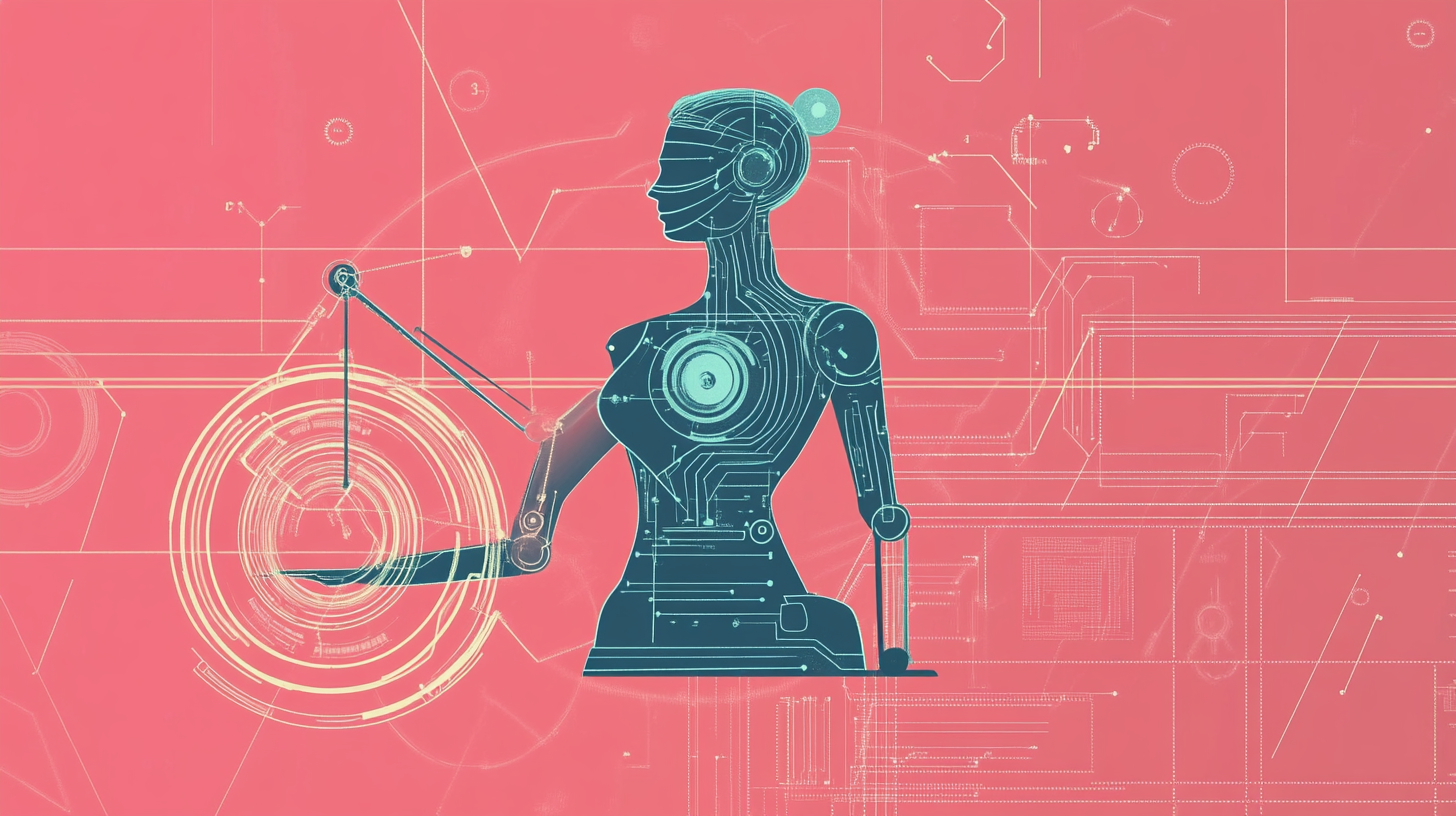
Abstract
Legal rules promulgated to govern emerging technologies often rely on proxy terms and metrics in order to indirectly effectuate background purposes. A common failure mode for this kind of rule occurs when, due to incautious drafting or unforeseen technological developments, a proxy ceases to function as intended and renders a rule ineffective or counterproductive. Borrowing a concept from the technical AI safety literature, we call this phenomenon the “governance misspecification problem.” This article draws on existing legal-philosophical discussions of the nature of rules to define governance misspecification, presents several historical case studies to demonstrate how and why rules become misspecified, and suggests best practices for designing legal rules to avoid misspecification or mitigate its negative effects. Additionally, we examine a few proxy terms used in existing AI governance regulations, such as “frontier AI” and “compute thresholds,” and discuss the significance of the problem of misspecification in the AI governance context.
In technical Artificial Intelligence (“AI”) safety research, the term “specification” refers to the problem of defining the purpose of an AI system so that the system behaves in accordance with the true wishes of its designer.1 Technical researchers have suggested three categories of specification: “ideal specification,” “design specification,” and “revealed specification.”2 The ideal specification, in this framework, is a hypothetical specification that would create an AI system completely and perfectly aligned with the desires of its creators. The design specification is the specification that is actually used to build a given AI system. The revealed specification is the specification that best describes the actual behavior of the completed AI system. “Misspecification” occurs whenever the revealed specification of an AI system diverges from the ideal specification—i.e., when an AI system does not perform in accordance with the intentions of its creators.
The fundamental problem of specification is that “it is often difficult or infeasible to capture exactly what we want an agent to do, and as a result we frequently end up using imperfect but easily measured proxies.”3 Thus, in a famous example from 2016, researchers at OpenAI attempted to train a reinforcement learning agent to play the boat-racing video game CoastRunners, the goal of which is to finish a race quickly and ahead of other players.4 Instead of basing the AI agent’s reward function on how it placed in the race, however, the researchers used a proxy goal that was easier to implement and rewarded the agent for maximizing the number of points it scored. The researchers mistakenly assumed that the agent would pursue this proxy goal by trying to complete the course quickly. Instead, the AI discovered that it could achieve a much higher score by refusing to complete the course and instead driving in tight circles in such a way as to repeatedly collect a series of power-ups while crashing into other boats and occasionally catching on fire.5 In other words, the design specification (“collect as many points as possible”) did not correspond well to the ideal specification (“win the race”), leading to a disastrous and unexpected revealed specification (crashing repeatedly and failing to finish the race).
This article applies the misspecification framework to the problem of AI governance. The resulting concept, which we call the “governance misspecification problem,” can be briefly defined as occurring when a legal rule relies unsuccessfully on proxy terms or metrics. By framing this new concept in terms borrowed from the technical AI safety literature, we hope to incorporate valuable insights from that field into legal-philosophical discussions around the nature of rules and, importantly, to help technical researchers understand the philosophical and policymaking challenges that AI governance legislation and regulation poses.
It is generally accepted among legal theorists that at least some legal rules can be said to have a purpose or purposes and that these purposes should inform the interpretation of textually ambiguous rules.6 The least ambitious version of this claim is simply an acknowledgment of the fact that statutes often contain a discrete textual provision entitled “Purpose,” which is intended to inform the interpretation and enforcement of the statute’s substantive provisions.7 More controversially, some commentators have argued that all or many legal rules have, or should be constructively understood as having, an underlying “true purpose,” which may or may not be fully discoverable and articulable.8
The purpose of a legal rule is analogous to the “ideal specification” discussed in the technical AI safety literature. Like the ideal specification of an AI system, a rule’s purpose may be difficult or impossible to perfectly articulate or operationalize, and rulemakers may choose to rely on a legal regime that incorporates “imperfect but easily measured proxies”—essentially, a design specification. “Governance misspecification” occurs when the real-world effects of the legal regime (analogous to the design specification) as interpreted and enforced (analogous to the revealed specification) fail to effectuate the rule’s intended purpose (analogous to the ideal specification).
Consider the hypothetical legal rule prohibiting the presence of “vehicles” in a public park, famously described by the legal philosopher H.L.A. Hart.9 The term “vehicles,” in this rule, is presumably a proxy term intended to serve some ulterior purpose,10 although fully discovering and articulating that purpose may be infeasible. For example, the rule might be intended to ensure the safety of pedestrians in the park, or to safeguard the health of park visitors by improving the park’s air quality, or to improve the park’s atmosphere by preventing excessive noise levels. More realistically, the purpose of the rule might be some complex weighted combination of all of these and numerous other more or less important goals. Whether the rule is misspecified depends on whether the rule’s purpose, whatever it is, is furthered by the use of the proxy term “vehicle.”
Hart used the “no vehicles in the park” rule in an attempt to show that the word “vehicle” had a core of concrete and settled linguistic meaning (an automobile is a vehicle) as well as a semantic “penumbra” containing more or less debatable cases such as bicycles, toy cars, and airplanes. The rule, in other words, is textually ambiguous, although this does not necessarily mean that it is misspecified.11 Because the rule is ambiguous, a series of difficult interpretive decisions may have to be made regarding whether a given item is or is not a vehicle. At least some of these decisions, and the costs associated with them, could have been avoided if the rulemaker had chosen to use a more detailed formulation in lieu of the term “vehicle,”12 or if the rulemaker had issued a statement clarifying the purpose of the rule.13
Although the concept of misspecification is generally applicable to legal rules, misspecification tends to occur particularly frequently and with serious consequences in the context of laws and regulations governing poorly-understood emerging technologies such as artificial intelligence. Again, consider “no vehicles in the park.” Many legal rules, once established, persist indefinitely even as the technology they govern changes fundamentally.14 The objects to which the proxy term “vehicle” can be applied will change over time; electric wheelchairs, for example, may not have existed when the rule was originally drafted, and airborne drones may not have been common. The introduction of these new potential “vehicles” is extremely difficult to account for in an original design specification.15
The governance misspecification problem is particularly relevant to the governance of AI systems. Unlike most other emerging technologies, frontier AI systems are, in key respects, not only poorly understood but fundamentally uninterpretable by existing methods.16 This problem of interpretability is a major focus area for technical AI safety researchers.17 The widespread use of proxy terms and metrics in existing AI governance policies and proposals is, therefore, a cause for concern.18
In Section I, this article draws on existing legal-philosophical discussions of the nature of rules to further explain the problem of governance misspecification and situates the concept in the existing public policy literature. Sections II and III make the case for the importance of the problem by presenting a series of case studies to show that rules aimed at governing emerging technologies are often misspecified and that misspecified rules can cause serious problems for the regulatory regime they contribute to, for courts, and for society generally. Section IV offers a few suggestions for reducing the risk of and mitigating the harm from misspecified rules, including eschewing or minimizing the use of proxy terms, rapidly updating and frequently reviewing the effectiveness of regulations, and including specific and clear statements of the purpose of a legal rule in the text of the rule. Section V applies the conclusions of the previous Sections prospectively to several specific challenges in the field of AI governance, including the use of compute thresholds, semiconductor export controls, and the problem of defining “frontier” AI systems. Section VI concludes.
I. The Governance Misspecification Problem in Legal Philosophy and Public Policy
A number of publications in the field of legal philosophy have discussed the nature of legal rules and arrived at conclusions helpful to fleshing out the contours of the governance misspecification problem.19 Notably, Schauer (1991) suggests the useful concepts of over- and under-inclusiveness, which can be understood as two common ways in which legal rules can become misspecified.20 Overinclusive rules prohibit or prescribe actions that an ideally specified rule would not apply to, while underinclusive rules fail to prohibit or prescribe actions that an ideally specified rule would apply to. So, in Hart’s “no vehicles in the park” hypothetical, suppose that the sole purpose of the rule was to prevent park visitors from being sickened by diesel fumes. If this were the case, the rule would be overinclusive, because it would pointlessly prohibit many vehicles that do not emit diesel fumes. If, on the other hand, the purpose of the rule was to prevent music from being played loudly in the park on speakers, the rule would be underinclusive, as it fails to prohibit a wide range of speakers that are not installed in a vehicle.
Ideal specification is rarely feasible, and practical considerations may dictate that a well-specified rule should rely on proxy terms that are under- or overinclusive to some extent. As Schauer (1991) explains, “Speed Limit 55” is a much easier rule to follow and enforce consistently than “drive safely,” despite the fact that the purpose of the speed limit is to promote safe driving and despite the fact that some safe driving can occur at speeds above 55 miles per hour and some dangerous driving can occur at speeds below 55 miles per hour.21 In other words, the benefits of creating a simple and easily followed and enforced rule outweigh the costs of over- and under-inclusiveness in many cases.22
In the public policy literature, the existing concept that bears the closest similarity to governance misspecification is “policy design fit.”23 Policy design is currently understood as including a mix of interrelated policy goals and the instruments through which those goals are accomplished, including legal, financial, and communicative mechanisms.24 A close fit between policy goals and the means used to accomplish those goals has been shown to increase the effectiveness of policies.25 The governance misspecification problem can be understood as a particular species of failure of policy design fit—a failure of congruence between a policy goal and a proxy term in the legal rule which is the means used to further that goal.26
II. Legal Rules Governing Emerging Technologies Are Often Misspecified
Misspecification occurs frequently in both domestic and international law and in both reactive and anticipatory regulations directed towards the regulation of new technologies. In order to illustrate how misspecification happens, and to give a sense of the significance of the problem in legal rules addressing emerging technologies, this Section discusses three historical examples of the phenomenon in the contexts of cyberlaw, copyright law, and nuclear anti-proliferation treaties.
Section 1201(a)(2) of the Digital Millennium Copyright Act of 1998 (DMCA) prohibits the distribution of any “technology, product, service, device, component, or part thereof” primarily designed to decrypt copyrighted material.27 Congressman Howard Coble, one of the architects of the DMCA, stated that this provision was “drafted carefully to target ‘black boxes’”—physical devices with “virtually no legitimate uses,” useful only for facilitating piracy.28 The use of “black boxes” for the decryption of digital works was not widespread in 1998, but the drafters of the DMCA predicted that such devices would soon become an issue. In 1998, this prediction seemed a safe bet, as previous forms of piracy decryption had relied on specialized tools—the phrase “black box” is a reference to one such tool, also known as a “descrambler” and used to decrypt premium cable television channels.29
However, the feared black boxes never arrived. Instead, pirates relied on software, using decryption programs distributed for free online to circumvent anti-piracy encryptions.30 Courts found the distribution of such programs, and even the posting of hyperlinks leading to websites containing such programs, to be violations of the DMCA.31 In light of earlier cases holding that computer code was a form of expression entitled to First Amendment protection, this interpretation placed the DMCA into tension with the First Amendment.32 This tension was ultimately resolved in favor of the DMCA, and the distribution of decryption programs used for piracy was prohibited.33
No one in Congress anticipated that the statute which had been “carefully drafted to target ‘black boxes’” would be used to prohibit the distribution of lines of computer code, or that this would raise serious concerns regarding freedom of speech. Section 1201(a)(2), in other words, was misspecified; by prohibiting the distribution of any “technology” or “service” designed for piracy, as well as any “device,” the framers of the DMCA banned more than they intended to ban and created unforeseen constitutional issues.
Misspecification also occurs in international law. The Treaty of Principles Governing the Activities of States in the Exploration and Use of Outer Space, which the United States and the Soviet Union entered into in 1967, obligated the parties “not to place in orbit around the Earth any objects carrying nuclear weapons…”34 Shortly after the treaty was entered into, however, it became clear that the Soviet Union planned to take advantage of a loophole in the misspecified prohibition. The Fractional Orbital Bombardment System (FOBS) placed missiles into orbital trajectories around the earth, but then redirected them to strike a target on the earth’s surface before they completed a full orbit.35 An object is not “in orbit” until it has circled the earth at least once; therefore, FOBS did not violate the 1967 Treaty, despite the fact that it allowed the Soviet Union to strike at the U.S. from space and thereby evade detection by the U.S.’s Ballistic Missile Early Warning System.36 The U.S. eventually neutralized this advantage by expanding the coverage and capabilities of early warning systems so that FOBS missiles could be detected and tracked, and in 1979 the Soviets agreed to a better-specified ban which prohibited “fractional orbital missiles” as well as other space-based weapons.37 Still, the U.S.’s agreement to use the underinclusive proxy term “in orbit” allowed the Soviet Union to temporarily gain a potentially significant first-strike advantage.
Misspecification occurs in laws and regulations directed towards existing and well-understood technologies as well as in anticipatory regulations. Take, for example, the Computer Fraud and Abuse Act (CFAA), 18 U.S.C. § 1030, which has been called “the worst law in technology.”38 The CFAA was originally enacted in 1984, but has since been amended several times, most recently in 2020.39 Among other provisions, the CFAA criminalizes “intentionally access[ing] a computer without authorization or exceed[ing] authorized access, and thereby obtain[ing]… information from any protected computer.”40 The currently operative language for this provision was introduced in 1996,41 by which point the computer was hardly an emerging technology, and slightly modified in 2008.42
Read literally, the CFAA’s prohibition on unauthorized access criminalizes both (a) violating a website’s terms of service while using the internet, and (b) using an employer’s computer or network for personal reasons, in violation of company policy.43 In other words, a literal reading of the CFAA would mean that hundreds of millions of Americans commit crimes every week by, e.g., sharing a password with a significant other or accessing social media at work.44 Court decisions eventually established narrower definitions of the key statutory terms (“without authorization” and “exceeds authorized access”),45 but not before multiple defendants were prosecuted for violating the CFAA by failing to comply a website’s terms of service46 or accessing an employer’s network for personal reasons in violation of workplace rules.47
Critics of the CFAA have discussed its flaws in terms of the constitutional law doctrines of “vagueness”48 and “overbreadth.”49 These flaws can also be conceptualized in terms of misspecification. The phrases “intentionally accesses without authorization” and “exceeds authorized access,” and the associated statutory definitions, are poor proxies for the range of behavior that an ideally specified version of the CFAA would have criminalized. The proxies criminalize a great deal of conduct that none of the stakeholders who drafted, advocated for, or voted to enact the law wanted to criminalize50 and created substantial legal and political backlash against the law. This backlash led to a series of losses for federal prosecutors as courts rejected their broad proposed interpretations of the key proxy terms because, as the Ninth Circuit Court of Appeals put it, “ubiquitous, seldom-prosecuted crimes invite arbitrary and discriminatory enforcement.”51 The issues caused by poorly selected proxy terms in the CFAA, the Outer Space Treaty, and the DMCA demonstrate that important legal rules drafted for the regulation of emerging technologies are prone to misspecification, in both domestic and international law contexts and for both anticipatory and reactive rules. These case studies were chosen because they are representative of how legal rules become misspecified; if space allowed, numerous additional examples of misspecified rules directed towards new technologies could be offered.52
III. Consequences of Misspecification in the Regulation of Emerging Technologies
The case studies examined in the previous Section established that legal rules are often misspecified and illustrated the manner in which the problem of governance misspecification typically arises. This Section attempts to show that misspecification can cause serious issues when it occurs for both for the regulatory regime that the misspecified rule is part of and for society writ large. Three potential consequences of misspecification are discussed and illustrated with historical examples involving the regulation of emerging technologies.
A. Underinclusive Rules Can Create Exploitable Gaps in a Regulatory Regime
When misspecification results in an underinclusive rule, exploitable gaps can arise in a regulatory regime. The Outer Space Treaty of 1967, discussed above, is one example of this phenomenon. Another example, which demonstrates how completely the use of a misspecified proxy term can defeat the effectiveness of a law, is the Audio Home Recording Act of 1992.53 That statute was designed to regulate home taping, i.e., the creation by consumers of analog or digital copies of musical recordings. The legal status of home taping had been a matter of debate for years, with record companies arguing that it was illegal and taping hardware manufacturers defending its legality.54 Congress attempted to resolve the debate by creating a safe harbor for home taping that allowed for the creation of any number of analog or digital copies of a piece of music, with the caveat that royalties would have to be paid as part of the purchase price of any equipment used to create digital copies.55
Congress designed the AHRA under the assumption that digital audio tape recorders (DATs) were the wave of the future and would shortly become a ubiquitous home audio appliance.56 The statute lays out, in painstaking detail, a complex regulatory framework governing “digital audio recording devices,” which the statute defines to require the capability to create reproductions of “digital musical recordings.”57 Bizarrely, however, the AHRA explicitly provides that the term “digital musical recording” does not encompass recordings stored on any object “in which one or more computer programs are fixed”—i.e., computer hard drives.58
Of course, the DAT did not become a staple of the American household. And when the RIAA sued the manufacturer of the “Rio,” an early mp3 player, for failing to comply with the AHRA’s requirements, the Ninth Circuit found that the device was not subject to the AHRA.59 Because the Rio was designed solely to download mp3 files from a computer hard drive, it was not capable of copying “digital musical recordings” under the AHRA’s underinclusive definition of that phrase.60 The court noted that its decision would “effectively eviscerate the Act,” because “[a]ny recording device could evade […] regulation simply by passing the music through a computer and ensuring that the MP3 file resided momentarily on the hard drive,” but nevertheless rejected the creative alternative interpretations suggested by the music industry as contrary to the plain language of the statute.61 As a result, the AHRA was rendered obsolete less than six years after being enacted.62
Clearly, Congress acted with insufficient epistemic humility by creating legislation confidently designed to address one specific technology that had not, at the time of legislation, been adopted by any significant portion of the population. But this failure of humility manifested as a failure of specification. The purpose of the statute, as articulated in a Senate report, included the introduction of a “serial copy management system that would prohibit the digital serial copying of copyrighted music.”63 By crafting a law that applied only to “digital audio recording devices” and defining that proxy term in an insufficiently flexible way, Congress completely failed to accomplish those purposes. If the proxy in question had not been defined to exclude any recording acquired through a computer, the Rio and eventually the iPod might well have fallen under the AHRA’s royalty scheme, and music copyright law in the U.S. might have developed down a course more consistent with the ideal specification of the AHRA.
B. Overinclusive Rules Can Create Pushback and Enforcement Challenges
Misspecification can also create overinclusive rules, like the Computer Fraud and Abuse Act and § 1201(a)(2) of the Digital Millennium Copyright Act, discussed above in Section II. As those examples showed, overinclusive rules may give rise to legal and political challenges, difficulties with enforcement, and other unintended and undesirable results. These effects can, in some cases, be so severe that they require a total repeal of the rule in question.
This was the case with a 2011 Nevada statute authorizing and regulating driverless cars. AB511, which was the first law of its kind enacted in the U.S.,64 initially defined “autonomous vehicle” to mean “a motor vehicle that uses artificial intelligence, sensors and global positioning system coordinates to drive itself without the active intervention of a human operator,” and further defined “artificial intelligence” to mean “the use of computers and related equipment to enable a machine to duplicate or mimic the behavior of human beings.”65
Shortly after AB511 was enacted, however, several commentators noted that the statute’s definition of “autonomous vehicle” technically included vehicles that incorporated automatic collision avoidance or any of a number of other advanced driver-assistance systems common in new cars in 2011.66 These systems used computers to temporarily control the operation of a vehicle without the intervention of the human driver, so any vehicle that incorporated them was technically subject to the onerous regulatory scheme that Nevada’s legislature had intended to impose only on fully autonomous vehicles. In order to avoid effectively banning most new model cars, Nevada’s legislature was forced to repeal its new law and enact a replacement that incorporated a more detailed definition of “autonomous vehicle.”67
C. Technological Change Can Repeatedly Render a Proxy Metric Obsolete
Finally, a misspecified rule may lose its effectiveness over time as technological advances render it obsolete, necessitating repeated updates and patches to the fraying regulatory regime. Consider, for example, the export controls imposed on high performance computers in the 1990s. The purpose of these controls was to prevent the export of powerful computers to countries where they might be used in ways that threatened U.S. national security, such as to design missiles and nuclear weapons.68 The government placed restrictions on the export of “supercomputers” and defined “supercomputer” in terms of the number of millions of theoretical operations per second (MTOPS) the computer could perform.69 In 1991, “supercomputer” was defined to mean any computer capable of exceeding 195 MTOPS.70 As the 90s progressed, however, the processing power of commercially available computers manufactured outside of the U.S. increased rapidly, reducing the effectiveness of U.S. export controls.71 Restrictions that prevented U.S. companies from selling their computers globally imposed costs on the U.S. economy and harmed the international competitiveness of the restricted companies.72 The Clinton administration responded by raising the threshold at which export restrictions began to apply to 1500 MTOPS in 1994, to 7000 MTOPS in 1996, to 12,300 MTOPS in 1999, and three times in the year 2000 to 20,000, 28,000, and finally 85,000 MTOPS.73
In the late 1990s, technological advances made it possible to link large numbers of commercially available computers together into “clusters” which could outperform most supercomputers.74 At this point, it was clear that MTOPS-based export controls were no longer effective, as computers that exceeded any limit imposed could easily be produced by anyone with access to a supply of less powerful computers which would not be subject to export controls.75 Even so, MTOPS-based export controls continued in force until 2006, when they were replaced by regulations that imposed controls based on performance in terms of Weighted TeraFLOPS, i.e., trillions of floating point operations per second.76
Thus, while the use of MTOPS thresholds as proxies initially resulted in well-specified export controls that effectively prevented U.S. adversaries from acquiring supercomputers, rapid technological progress repeatedly rendered the controls overinclusive and necessitated a series of amendments and revisions. The end result was a period of nearly seven years during which the existing export controls were badly misspecified due to the use of a proxy metric, MTOPS, which no longer bore any significant relation to the regime’s purpose. During this period, the U.S. export control regime for high performance computers was widely considered to be ineffective and perhaps even counterproductive.77
IV. Mitigating Risks from Misspecification
In light of the frequency with which misspecification occurs in the regulation of emerging technology and the potential severity of its consequences, this Section suggests a few techniques for designing legal rules in such a way as to reduce the risk of misspecification and mitigate its ill effects.
The simplest way to avoid misspecification is to eschew or minimize the use of proxy terms and metrics. This is not always practicable or desirable. “No vehicles in the park” is a better rule than “do not unreasonably annoy or endanger the safety of park visitors,” in part because it reduces the cognitive burden of following, enforcing, and interpreting the rule and reduces the risk of decision maker error by limiting the discretion of the parties charged with enforcement and interpretation.78 Nevertheless, there are successful legal rules that pursue their purposes directly. U.S. antitrust law, for example, grew out of the Sherman Antitrust Act,79 § 1 of which simply states that any combination or contract in restraint of trade “is declared to be illegal.”
Where use of a proxy is appropriate, it is often worthwhile to identify the fact that a proxy is being used to reduce the likelihood that decision makers will fall victim to Goodhart’s law80 and treat the regulation of the proxy as an end in itself.81 Alternatively, the most direct way to avoid confusion regarding the underlying purpose of a rule is to simply include an explanation of the purpose in the text of the rule itself. This can be accomplished through the addition of a purpose clause (sometimes referred to as a legislative preamble or a policy statement). For example, one purpose of the Nuclear Energy Innovation and Modernization Act of 2019 is to “provide… a program to develop the expertise and regulatory processes necessary to allow innovation and the commercialization of advanced nuclear reactors.”
Purpose clauses can also incorporate language emphasizing that every provision of a rule should be construed in order to effectuate its purpose. This amounts to a legislatively prescribed rule of statutory interpretation, instructing courts to adopt a purposivist interpretive approach.82 When confronted with an explicit textual command to this effect, even strict textualists are obligated to interpret a rule purposively.83 The question of whether such an approach is generally desirable is hotly debated,84 but in the context of AI governance the flexibility that purposivism provides is a key advantage. The ability to flexibly update and adapt a rule in response to changes in the environment in which the rule will apply is unusually important in the regulation of emerging technologies.85 While there is little empirical evidence for or against the effectiveness of purpose clauses, they have played a key role in the legal reasoning relied on in a number of important court decisions.86
A regulatory regime can also require periodic efforts to evaluate whether a rule is achieving its purpose.87 These efforts can provide an early warning system for misspecification by facilitating awareness of whether the proxy terms or metrics relied upon still correspond well to the purpose of the rule. Existing periodic review requirements are often ineffective,88 treated by agencies as box-checking activities rather than genuine opportunities for careful retrospective analysis of the effects of regulations.89 However, many experts continue to recommend well-implemented retrospective review requirements as an effective tool for improving policy decisions.90 The Administrative Conference of the United States has repeatedly pushed for increased use of retrospective review, as has the internationally-focused Organization for Economic Co-Operation and Development (OECD).91 Additionally, retrospective review of regulations often works well in countries outside of the U.S.92
As the examples in Sections II and III demonstrate, rules governing technology tend to become misspecified over time as the regulated technology evolves. The Outer Space Treaty of 1967, § 1201(a)(2) of the DMCA, and the Clinton Administration’s supercomputer export controls were all well-specified and effective when implemented, but each measure became ineffective or counterproductive soon after being implemented because the proxies relied upon became obsolete. Ideally, rulemaking would move at the pace of technological improvement, but there are a number of institutional and structural barriers to this sort of rapid updating of regulations. Notably, the Administrative Procedure Act requires a lengthy “notice and comment” process for rulemaking and a 30-day waiting period after publication of a regulation in the Federal Register before the regulation can go into effect.93 There are ways to waive or avoid these requirements, including regulating via the issuance of nonbinding guidance documents rather than binding rules,94 issuing an immediately effective “interim final rule” and then satisfying the APA’s requirements at a later time,95 waiving the publication or notice and comment requirements for “good cause,”96 or legislatively imposing regulatory deadlines.97 Many of these workarounds are limited in their scope or effectiveness, or vulnerable to legal challenges if pursued too ambitiously, but finding some way to update a regulatory regime quickly is critical to mitigating the damage caused by misspecification.98
There is reason to believe that some agencies, recognizing the importance of AI safety to national security, will be willing to rapidly update regulations despite the legal and procedural difficulties. Consider the Commerce Department’s recent response to repeated attempts by semiconductor companies to design chips for the Chinese market that comply with U.S. export control regulations while still providing significant utility to purchasers in China looking to train advanced AI models. After Commerce initially imposed a license requirement on the export of advanced AI-relevant chips to China in October 2022, Nvidia modified its market-leading A100 and H100 chips to comply with the regulations and proceeded to sell the modified A800 and H800 chips in China.99 On October 17, 2023, the Commerce Department’s Bureau of Industry and Security announced a new interim final rule that would prohibit the sale of A800 and H800 chips in China and waived the normal 30-day waiting period so that the rule became effective less than a week after it was announced.100 Commerce Secretary Gina Raimondo stated publicly that “”[i]f [semiconductor companies] redesign a chip around a particular cut line that enables them to do AI, I’m going to control it the very next day.”101
V. The Governance Misspecification Problem and Artificial Intelligence
While the framework of governance misspecification is applicable to a wide range of policy measures, it is particularly well-suited to describing issues that arise regarding legal rules governing emerging technologies. H.L.A. Hart’s prohibition on “vehicles in the park” could conceivably have been framed by an incautious drafter who did not anticipate that using “vehicle” instead of some more detailed proxy term would create ambiguity. Avoiding this kind of misspecification is simply a matter of careful drafting. Suppose, however, that the rule was formulated at a point in time when “vehicle” was an appropriate proxy for a well-understood category of object, and the rule later became misspecified as new potential vehicles that had not been conceived of when the rule was drafted were introduced. A rule drafted at a historical moment when all vehicles move on either land or water is unlikely to adequately account for the issues created by airplanes or flying drones.102
In other words, rules created to govern emerging technologies are especially prone to misspecification because they are created in the face of a high degree of uncertainty regarding the nature of the subject matter to be regulated, and rulemaking under uncertainty is difficult.103 Furthermore, as the case studies discussed in Sections II and III show, the nature of this difficulty is such that it tends to result in misspecification. For instance, misspecification will usually result when an overconfident rulemaker makes a specific and incorrect prediction about the future and issues an underinclusive rule based on that prediction. This was the case when Congress addressed the AHRA exclusively to digital audio tape recorders and ignored computers. Rules created by rulemakers who want to regulate a certain technology but have only a vague and uncertain understanding of the purpose they are pursuing are also likely to be misspecified.104 Hence the CFAA, which essentially prohibited “doing bad things with a computer,” with disastrous results.
The uncertainties associated with emerging technologies and the associated risk of misspecification increase when the regulated technology is poorly understood. Rulemakers may simply overlook something about the chosen proxy due to a lack of understanding of the proxy or the underlying technology, or due to a lack of experience drafting the kinds of regulations required. The first-of-its-kind Nevada law intended to regulate fully autonomous vehicles that accidentally regulated a broad range of features common in many new cars is an example of this phenomenon. So is the DMCA provision that was intended to regulate “black box” devices but, by its terms, also applied to raw computer code.
If the difficulty of making well-specified rules to govern emerging technologies increases when the technology is fast-developing and poorly understood, advanced AI systems are something of a perfect storm for misspecification problems. Cutting-edge deep learning AI systems differ from other emerging technologies in that their workings are poorly understood, not just by legislators and the public, but by their creators.105 Their capabilities are an emergent property of the interaction between their architecture and the vast datasets on which they are trained. Moreover, the opacity of these models is arguably different in kind from the unsolved problems associated with past technological breakthroughs, because the models may be fundamentally uninterpretable rather than merely difficult to understand.106 Under these circumstances, defining an ideal specification in very general terms may be simple enough, but designing legal rules to operationalize any such specification will require extensive reliance on rough proxies. This is fertile ground for misspecification.
There are a few key proxy terms that recur often in existing AI governance proposals and regulations. For example, a number of policy proposals have suggested that regulations should focus on “frontier” AI models.107 When Google, Anthropic, OpenAI, and Microsoft created an industry-led initiative to promote AI safety, they named it the Frontier Model Forum.108 Sam Altman, the CEO of OpenAI, has expressed support for regulating “frontier systems.”109 The government of the U.K. has established a “Frontier AI Taskforce” dedicated to evaluating risks “at the frontier of AI.”110
In each of these proposals, the word “frontier” is a proxy term that stands for something like “highly capable foundation models that could possess dangerous capabilities sufficient to pose severe risks to public safety.”111 Any legislation or regulation that relied on the term “frontier” would also likely include a statutory definition of the word,112 but as several of the historical examples discussed in Sections II and III showed, statutory definitions can themselves incorporate proxies that result in misspecification. The above definition, for instance, may be underinclusive because some models that cannot be classified as “highly capable” or as “foundation models” might also pose severe risks to public safety.
The most significant AI-related policy measure that has been issued in the U.S. to date is Executive Order (EO) 14110 on the “Safe, Secure, and Trustworthy Development and Use of Artificial Intelligence.”113 Among many other provisions, the EO imposes reporting requirements on certain AI models and directs the Department of Commerce to define the category of models to which the reporting requirements will apply.114 Prior to the issuance of Commerce’s definition, the EO provides that the reporting requirements apply to models “trained using a quantity of computing power greater than 1026 integer or floating-point operations, or using primarily biological sequence data and using a quantity of computing power greater than 1023 integer or floating-point operations,” as well as certain computing clusters.115 In other words, the EO uses operations as a proxy metric for determining which AI systems are sufficiently capable and/or dangerous that they should be regulated. This kind of metric, which is based on the amount of computing power used to train a model, is known as a “compute threshold” in the AI governance literature.116
A proxy metric such as an operations-based compute threshold is almost certainly necessary to the operationalization of the EO’s regulatory scheme for governing frontier models.117 Even so, the example of the U.S. government’s ultimately ineffective and possibly counterproductive attempts to regulate exports of high performance computers using MTOPS is a cautionary tale about how quickly a compute-based proxy can be rendered obsolete by technological progress. The price of computing resources has, historically, fallen rapidly, with the amount of compute available for a given sum of money doubling approximately every two years as predicted by Moore’s Law.118 Additionally, because of improvements in algorithmic efficiency, the amount of compute required to train a model to a given level of performance has historically decreased over time as well.119 Because of these two factors, the cost of training AI models to a given level of capability has fallen precipitously over time; for instance, between 2017 and 2021, the cost of training a rudimentary model to classify images correctly with 93% accuracy on the image database ImageNet fell from $1000 to $5.120 This phenomenon presents a dilemma for regulators: the cost of acquiring computational resources exceeding a given threshold will generally decrease over time even as the capabilities of models trained on a below-threshold amount of compute rises. In other words, any well-specified legal rule that uses a compute threshold is likely to be rendered both overinclusive and underinclusive soon after being implemented.
Export controls intended to prevent the proliferation of the advanced chips used to train frontier AI models face a similar problem. Like the Clinton Administration’s supercomputer export controls, the Biden administration’s export controls on chips like the Nvidia A800 and H800 are likely to become misspecified over time. As algorithmic efficiency increases and powerful chips become cheaper and easier to acquire, existing semiconductor export controls will gradually become both overinclusive (because they pointlessly prohibit the export of chips that are already freely available overseas) and underinclusive (because powerful AI models can be trained using chips not covered by the export controls).
The question of precisely how society should respond to these developments over time is beyond the scope of this paper. However, to delay the onset of misspecification and mitigate its effects, policymakers setting legal rules for AI governance should consider the recommendations outlined in Section IV, above. So, the specifications for export controls on semiconductors—proxies for something like “chips that can be used to create dangerously powerful AI models”—should be updated quickly and frequently as needed, to prevent them from becoming ineffective or counterproductive. The Bureau of Industry and Security has already shown some willingness to pursue this kind of frequent, flexible updating.121 More generally, given the particular salience of the governance misspecification problem to AI governance, legislators should consider mandating frequent review of the effectiveness of important AI regulations and empowering administrative agencies to update regulations rapidly as necessary. Rules setting compute thresholds that are likely to be the subject of litigation should incorporate clear purpose statements articulating the ulterior purpose behind the use of a compute threshold as a proxy, and should be interpreted consistently with those statements. And where it is possible to eschew the use of proxies without compromising the enforceability or effectiveness of a rule, legislators and regulators should consider doing so.
VI. Conclusion
This article has attempted to elucidate a newly developed concept in governance, i.e., the problem of governance misspecification. In presenting this concept along with empirical insights from representative case studies, we hope to inform contemporary debates around AI governance by demonstrating one common and impactful way in which legal rules can fail to effect their purposes. By framing this problem in terms of “misspecification,” a concept borrowed from the technical AI safety literature, this article aims both to introduce valuable insights from that field to scholars of legal philosophy and public policy and to introduce technical researchers to some of the more practically salient legal-philosophical and governance-related challenges involved in AI legislation and regulation. Additionally, we have offered a few specific suggestions for avoiding or mitigating the harms of misspecification in the AI governance context, namely eschewing the use of proxy terms or metrics where feasible, clear statements of statutory purpose, and flexibly applied, rapidly updating, periodically reviewed regulations.
A great deal of conceptual and empirical work remains to be done regarding the nature and effects of the governance misspecification problem and best practices for avoiding and responding to it. For instance, this article does not contain any in-depth comparison of the incidence and seriousness of misspecification outside of the context of rules governing emerging technologies. Additionally, empirical research analyzing whether and how purpose clauses and similar provisions can effectively further the purposes of legal rules would be of significant practical value.